Quantitative Analysis of Aflatoxin B1 of Peanut by Optimized Support Vector Machine Models Based on Near-Infrared Spectral Features.
SPECTROCHIMICA ACTA PART A-MOLECULAR AND BIOMOLECULAR SPECTROSCOPY(2023)
摘要
This study designs a chemometric framework for quantitatively evaluating aflatoxin B1 (AFB1) in peanuts based on near-infrared (NIR) spectroscopy technique. The NIR spectra of peanut samples exhibiting diverse fungal contamination levels were acquired using a portable NIR spectrometer. Subsequently, appropriate pre-processing techniques were employed for data refinement. To streamline the analysis, the iterative variable subset optimization (IVSO) technique was employed to conduct an initial screening of the pre-processed NIR spectra, eliminating numerous irrelevant variables. Building upon this screening process, the beluga whale optimization (BWO) algorithm was utilized to optimize the selected feature variables further. Subsequently, support vector machine (SVM) models were developed using the refined near-infrared spectral features to test AFB1 in peanuts quantitatively. The results indicate that the SVM model significantly improves detection performance and generalization proficiency, particularly after secondary optimization using BWO-IVSO. Among the different models considered, the SVM model established after BWO-IVSO optimization exhibited the most extraordinary level of generalization, with a root mean square error of prediction of 24.6322 & mu;g & BULL;kg 1, a correlation coefficient of 0.9761, and a relative percent deviation of 4.6999. Overall, this investigation highlights the effectiveness of the proposed NIR spectroscopy model based on BWO-IVSO-SVM for quantitatively analyzing AFB1 in peanuts. The study contributes valuable technical and methodological insights that can serve as a reference for rapidly determining mycotoxins in cereal crops.
更多查看译文
关键词
Aflatoxin B 1,Peanut,Near-infrared spectroscopy,Chemometrics analysis
AI 理解论文
溯源树
样例
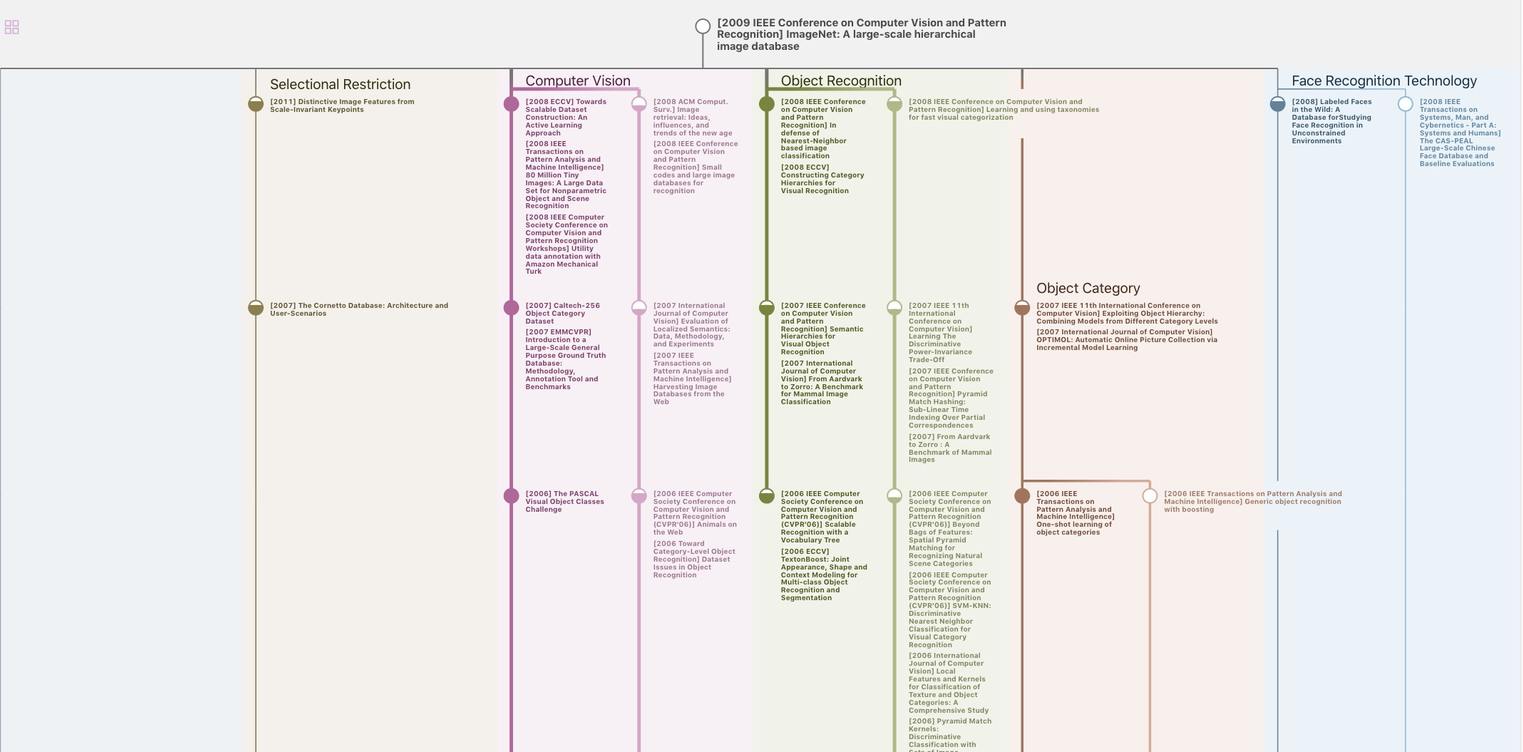
生成溯源树,研究论文发展脉络
数据免责声明
页面数据均来自互联网公开来源、合作出版商和通过AI技术自动分析结果,我们不对页面数据的有效性、准确性、正确性、可靠性、完整性和及时性做出任何承诺和保证。若有疑问,可以通过电子邮件方式联系我们:report@aminer.cn