Efficient Title Reranker for Fast and Improved Knowledge-Intense NLP
CoRR(2023)
摘要
In recent RAG approaches, rerankers play a pivotal role in refining retrieval
accuracy with the ability of revealing logical relations for each pair of query
and text. However, existing rerankers are required to repeatedly encode the
query and a large number of long retrieved text. This results in high
computational costs and limits the number of retrieved text, hindering
accuracy. As a remedy of the problem, we introduce the Efficient Title Reranker
via Broadcasting Query Encoder, a novel technique for title reranking that
achieves a 20x-40x speedup over the vanilla passage reranker. Furthermore, we
introduce Sigmoid Trick, a novel loss function customized for title reranking.
Combining both techniques, we empirically validated their effectiveness,
achieving state-of-the-art results on all four datasets we experimented with
from the KILT knowledge benchmark.
更多查看译文
AI 理解论文
溯源树
样例
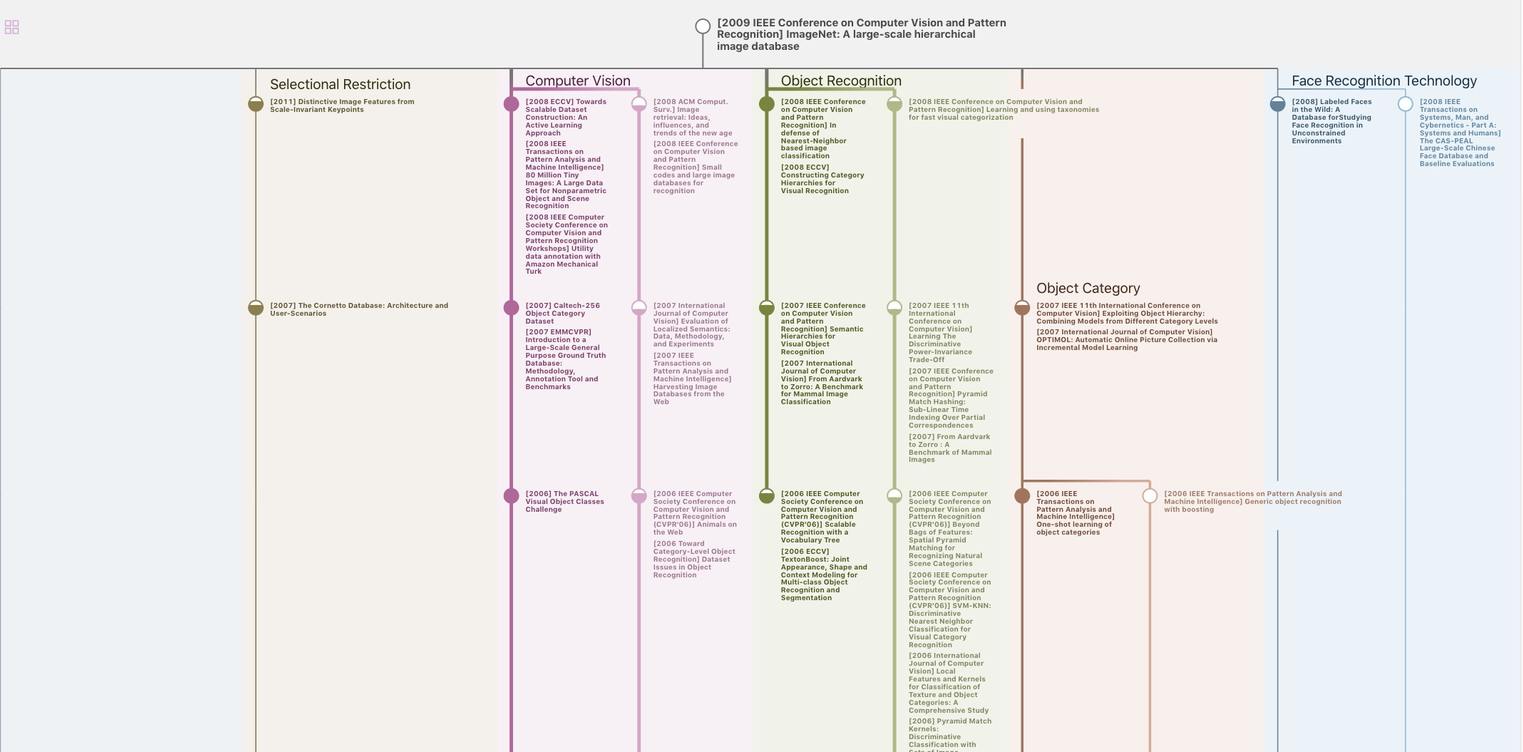
生成溯源树,研究论文发展脉络
数据免责声明
页面数据均来自互联网公开来源、合作出版商和通过AI技术自动分析结果,我们不对页面数据的有效性、准确性、正确性、可靠性、完整性和及时性做出任何承诺和保证。若有疑问,可以通过电子邮件方式联系我们:report@aminer.cn