Fast-NTK: Parameter-Efficient Unlearning for Large-Scale Models
Computer Vision and Pattern Recognition(2024)
摘要
The rapid growth of machine learning has spurred legislative initiatives such as “the Right to be Forgotten,” allowing users to request data removal. In response, “machine unlearning” proposes the selective removal of unwanted data without the need for retraining from scratch. While the Neural-Tangent-Kernel-based (NTK-based) unlearning method excels in performance, it suffers from significant computational complexity, especially for large-scale models and datasets. Our work introduces “Fast-NTK,” a novel NTK-based unlearning algorithm that significantly reduces the computational complexity by incorporating parameter-efficient fine-tuning methods, such as fine-tuning batch normalization layers in a CNN or visual prompts in a vision transformer. Our experimental results demonstrate scalability to much larger neural networks and datasets (e.g., 88M parameters; 5k images), surpassing the limitations of previous full-model NTK-based approaches designed for smaller cases (e.g., 8M parameters; 500 images). Notably, our approach maintains a performance comparable to the traditional method of retraining on the retain set alone. Fast-NTK can thus enable for practical and scalable NTK-based unlearning in deep neural networks.
更多查看译文
关键词
Large-scale Models,Neural Network,Scalable,Convolutional Neural Network,Deep Network,Computational Complexity,Deep Neural Network,Large-scale Datasets,Batch Normalization,Batch Normalization Layer,Vision Transformer,Large Neural Networks,Convolutional Layers,Multilayer Perceptron,Model Weights,Entire Model,Linear Probe,Drawing Inspiration,Subset Of Parameters,Hold-out Set,Multilayer Perceptron Layer,Multi-head Self-attention,Parameter Count
AI 理解论文
溯源树
样例
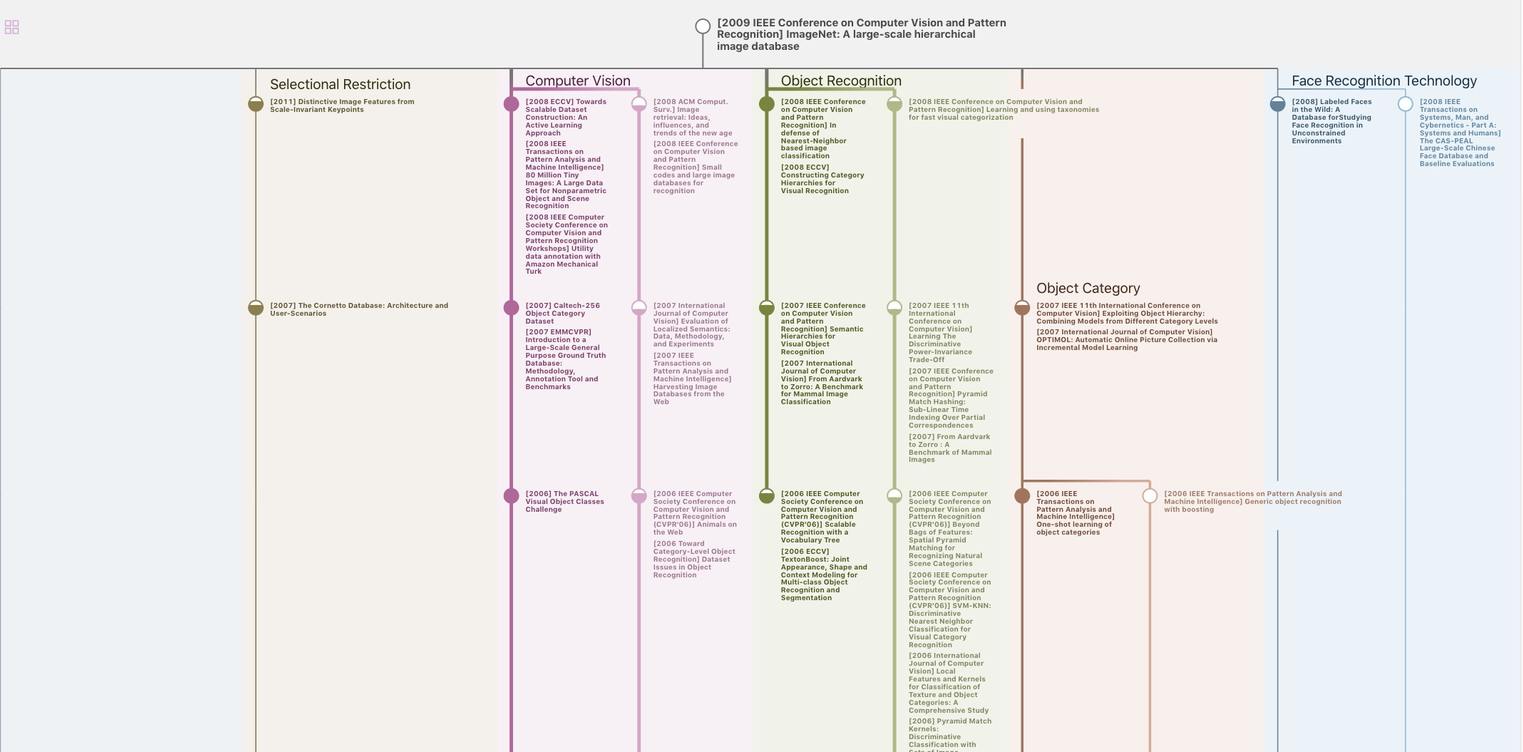
生成溯源树,研究论文发展脉络
数据免责声明
页面数据均来自互联网公开来源、合作出版商和通过AI技术自动分析结果,我们不对页面数据的有效性、准确性、正确性、可靠性、完整性和及时性做出任何承诺和保证。若有疑问,可以通过电子邮件方式联系我们:report@aminer.cn