Accurate and Semantic 3D Reconstruction of Maize Leaves
COMPUTERS AND ELECTRONICS IN AGRICULTURE(2024)
摘要
The rapid advances in equipment and technology for crop three-dimensional (3D) data acquisition have enabled the high-throughput and high-quality acquisition of crop 3D data. However, the challenge now lies in comprehending unstructured crop 3D data and extracting phenotypes and knowledge. Extracting semantic feature points from plant leaves and achieving high-precision 3D reconstruction is a prevalent and formidable issue in this field. In this study, we present an accurate method for semantic 3D reconstruction of maize leaves. The algorithm utilizes 3D point cloud data of maize leaves as the initial input. Subsequently, the semantic 3D reconstruction of maize leaves is achieved by means of point cloud preprocessing, initial triangular meshing, hole filling, remeshing, mesh parameterization, two-dimensional (2D) edge detection, 2D semantic point interpolation, 3D semantic point selection, mesh generation, and optimization of feature-point position. A total of 56 leaves from five different maize cultivars were reconstructed utilizing this method. The results indicate that the average distance error index between the reconstructed mesh vertices and the input point cloud is less than 0.3 cm2 for all five cultivars, and the mean of the maximum distance error index for each cultivar is within the range of 0.5-0.8 cm, indicating it is high-accuracy. The visualization of leaves shows good consistency between the reconstructed mesh and corresponding point cloud. Additionally, the reconstructed 3D mesh of the leaves exhibits enhanced triangular facet consistency. Each vertex can be precisely identified as a leaf vein point, leaf edge point, leaf tip point, or leaf surface point, and includes semantic data such as the position on the leaf blade, which can be directly employed for phenotype estimations. High-precision reconstruction of 3D models of crop organs that include semantic information and cultivar resolution yields significant benefits. This technology can improve the resolution and accuracy of crop morphological and structural research, and it is also crucial for crop phenomics, functional-structural plant modelling, and fusion research between plant phenotyping and crop models.
更多查看译文
关键词
Three-dimensional point cloud,Semantic reconstruction,Maize leaf,Plant phenotyping
AI 理解论文
溯源树
样例
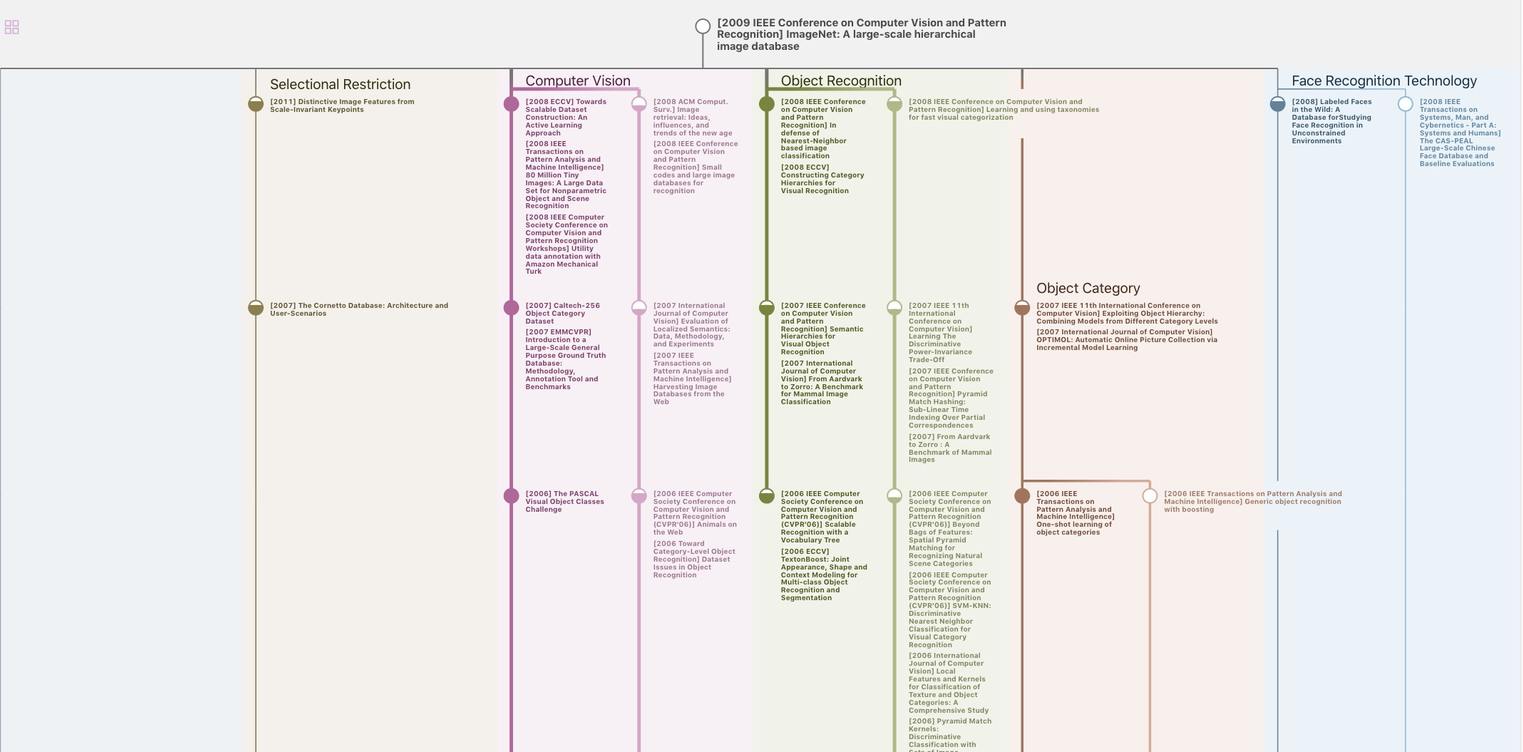
生成溯源树,研究论文发展脉络
数据免责声明
页面数据均来自互联网公开来源、合作出版商和通过AI技术自动分析结果,我们不对页面数据的有效性、准确性、正确性、可靠性、完整性和及时性做出任何承诺和保证。若有疑问,可以通过电子邮件方式联系我们:report@aminer.cn