Secrets of RLHF in Large Language Models Part II: Reward Modeling
arXiv (Cornell University)(2024)
摘要
Reinforcement Learning from Human Feedback (RLHF) has become a crucialtechnology for aligning language models with human values and intentions,enabling models to produce more helpful and harmless responses. Reward modelsare trained as proxies for human preferences to drive reinforcement learningoptimization. While reward models are often considered central to achievinghigh performance, they face the following challenges in practical applications:(1) Incorrect and ambiguous preference pairs in the dataset may hinder thereward model from accurately capturing human intent. (2) Reward models trainedon data from a specific distribution often struggle to generalize to examplesoutside that distribution and are not suitable for iterative RLHF training. In this report, we attempt to address these two issues. (1) From a dataperspective, we propose a method to measure the strength of preferences withinthe data, based on a voting mechanism of multiple reward models. Experimentalresults confirm that data with varying preference strengths have differentimpacts on reward model performance. We introduce a series of novel methods tomitigate the influence of incorrect and ambiguous preferences in the datasetand fully leverage high-quality preference data. (2) From an algorithmicstandpoint, we introduce contrastive learning to enhance the ability of rewardmodels to distinguish between chosen and rejected responses, thereby improvingmodel generalization. Furthermore, we employ meta-learning to enable the rewardmodel to maintain the ability to differentiate subtle differences inout-of-distribution samples, and this approach can be utilized for iterativeRLHF optimization.
更多查看译文
关键词
Language Modeling,Interpretable Models,Topic Modeling,Model Interpretability
AI 理解论文
溯源树
样例
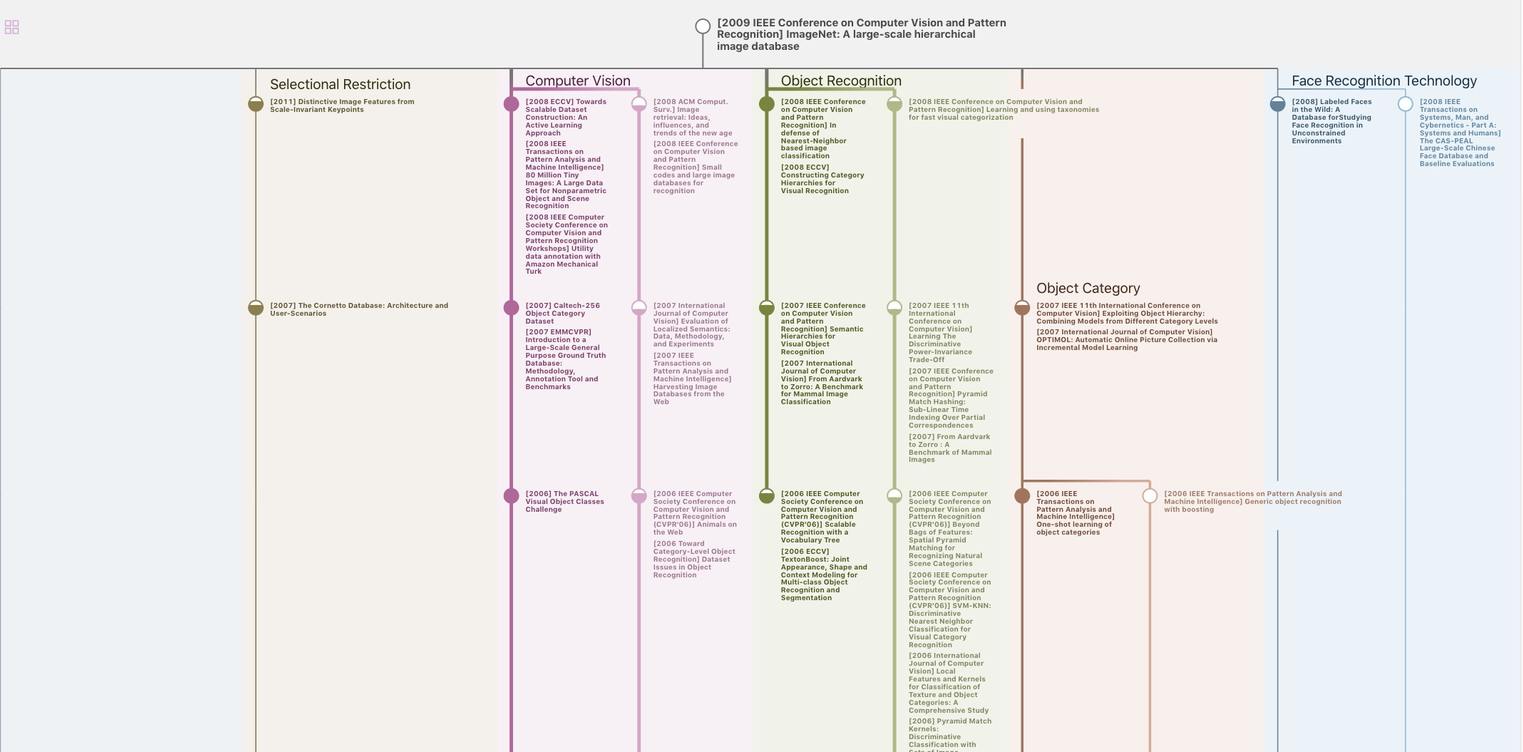
生成溯源树,研究论文发展脉络
数据免责声明
页面数据均来自互联网公开来源、合作出版商和通过AI技术自动分析结果,我们不对页面数据的有效性、准确性、正确性、可靠性、完整性和及时性做出任何承诺和保证。若有疑问,可以通过电子邮件方式联系我们:report@aminer.cn