Human-Instruction-Free LLM Self-Alignment with Limited Samples
CoRR(2024)
摘要
Aligning large language models (LLMs) with human values is a vital task for
LLM practitioners. Current alignment techniques have several limitations: (1)
requiring a large amount of annotated data; (2) demanding heavy human
involvement; (3) lacking a systematic mechanism to continuously improve. In
this work, we study aligning LLMs to a new domain with limited samples (e.g. <
100). We propose an algorithm that can self-align LLMs iteratively without
active human involvement. Unlike existing works, our algorithm relies on
neither human-crafted instructions nor labeled rewards, significantly reducing
human involvement. In addition, our algorithm can self-improve the alignment
continuously. The key idea is to first retrieve high-quality samples related to
the target domain and use them as In-context Learning examples to generate more
samples. Then we use the self-generated samples to finetune the LLM
iteratively. We show that our method can unlock the LLMs' self-generalization
ability to perform alignment with near-zero human supervision. We test our
algorithm on three benchmarks in safety, truthfulness, and
instruction-following, and show good performance in alignment, domain
adaptability, and scalability.
更多查看译文
AI 理解论文
溯源树
样例
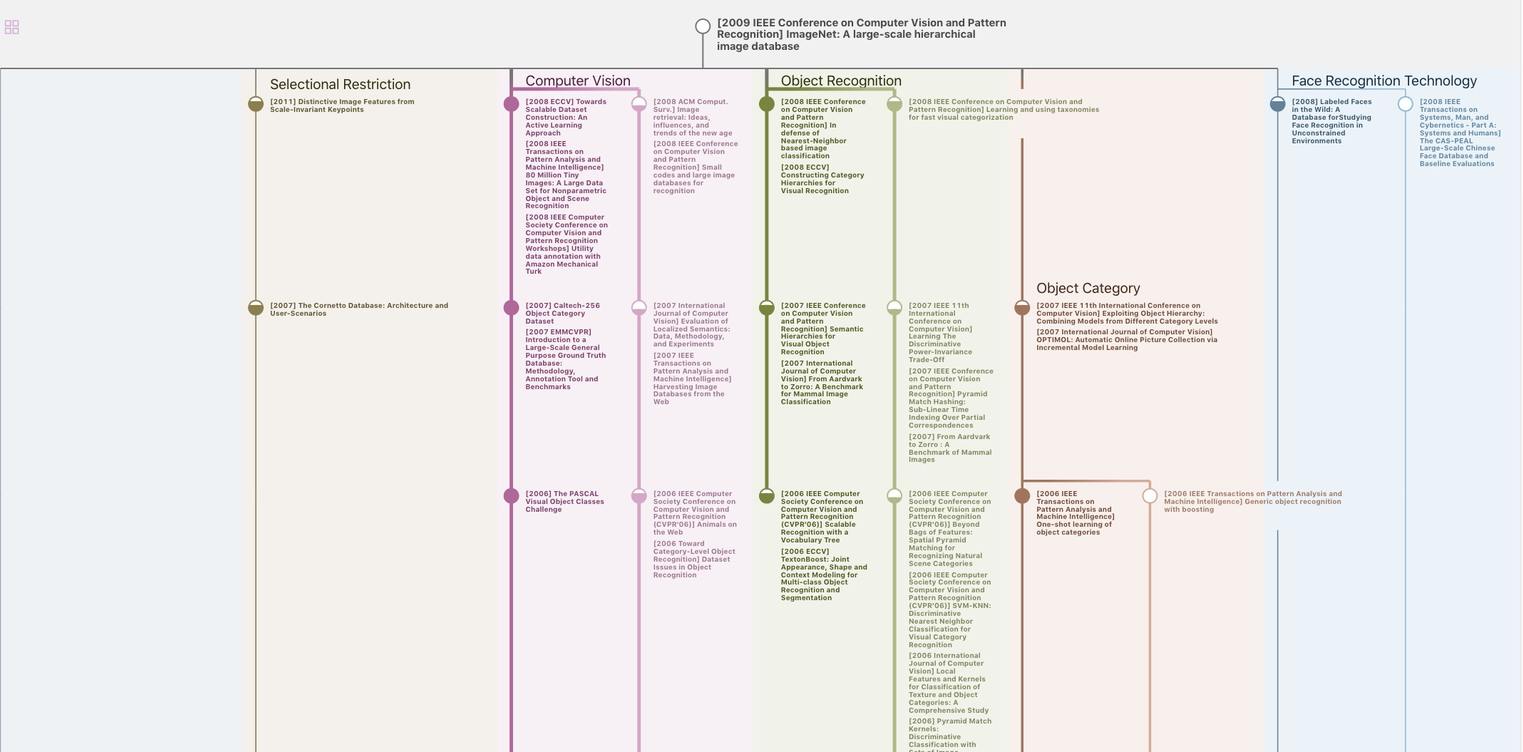
生成溯源树,研究论文发展脉络
数据免责声明
页面数据均来自互联网公开来源、合作出版商和通过AI技术自动分析结果,我们不对页面数据的有效性、准确性、正确性、可靠性、完整性和及时性做出任何承诺和保证。若有疑问,可以通过电子邮件方式联系我们:report@aminer.cn