DeepSpeed-FastGen: High-throughput Text Generation for LLMs via MII and DeepSpeed-Inference
CoRR(2024)
摘要
The deployment and scaling of large language models (LLMs) have become
critical as they permeate various applications, demanding high-throughput and
low-latency serving systems. Existing frameworks struggle to balance these
requirements, especially for workloads with long prompts. This paper introduces
DeepSpeed-FastGen, a system that employs Dynamic SplitFuse, a novel prompt and
generation composition strategy, to deliver up to 2.3x higher effective
throughput, 2x lower latency on average, and up to 3.7x lower (token-level)
tail latency, compared to state-of-the-art systems like vLLM. We leverage a
synergistic combination of DeepSpeed-MII and DeepSpeed-Inference to provide an
efficient and easy-to-use serving system for LLMs. DeepSpeed-FastGen's advanced
implementation supports a range of models and offers both non-persistent and
persistent deployment options, catering to diverse user scenarios from
interactive sessions to long-running applications. We present a detailed
benchmarking methodology, analyze the performance through latency-throughput
curves, and investigate scalability via load balancing. Our evaluations
demonstrate substantial improvements in throughput and latency across various
models and hardware configurations. We discuss our roadmap for future
enhancements, including broader model support and new hardware backends. The
DeepSpeed-FastGen code is readily available for community engagement and
contribution.
更多查看译文
AI 理解论文
溯源树
样例
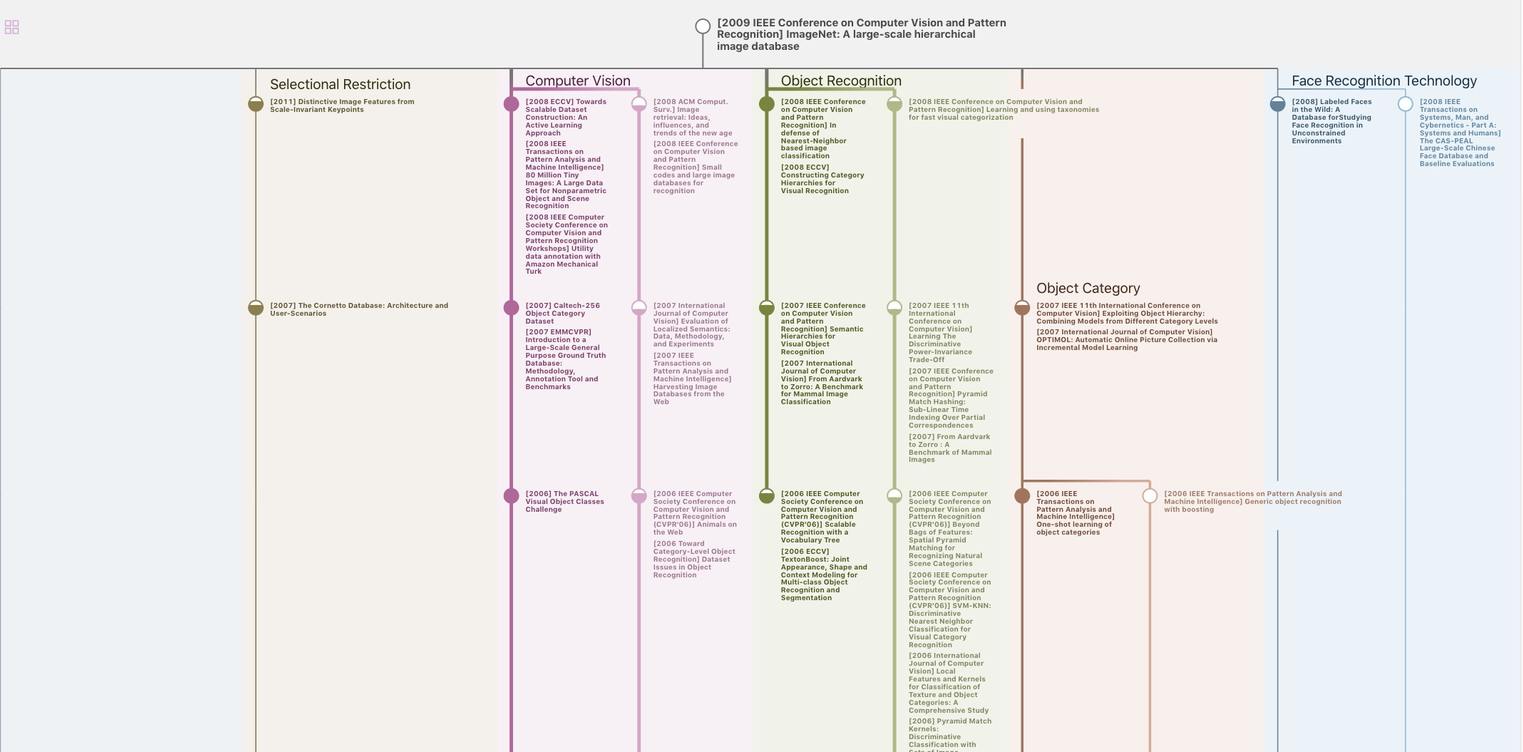
生成溯源树,研究论文发展脉络
数据免责声明
页面数据均来自互联网公开来源、合作出版商和通过AI技术自动分析结果,我们不对页面数据的有效性、准确性、正确性、可靠性、完整性和及时性做出任何承诺和保证。若有疑问,可以通过电子邮件方式联系我们:report@aminer.cn