SM^3: Self-Supervised Multi-task Modeling with Multi-view 2D Images for Articulated Objects
IEEE International Conference on Robotics and Automation(2024)
摘要
Reconstructing real-world objects and estimating their movable jointstructures are pivotal technologies within the field of robotics. Previousresearch has predominantly focused on supervised approaches, relying onextensively annotated datasets to model articulated objects within limitedcategories. However, this approach falls short of effectively addressing thediversity present in the real world. To tackle this issue, we propose aself-supervised interaction perception method, referred to as SM^3, whichleverages multi-view RGB images captured before and after interaction to modelarticulated objects, identify the movable parts, and infer the parameters oftheir rotating joints. By constructing 3D geometries and textures from thecaptured 2D images, SM^3 achieves integrated optimization of movable part andjoint parameters during the reconstruction process, obviating the need forannotations. Furthermore, we introduce the MMArt dataset, an extension ofPartNet-Mobility, encompassing multi-view and multi-modal data of articulatedobjects spanning diverse categories. Evaluations demonstrate that SM^3surpasses existing benchmarks across various categories and objects, while itsadaptability in real-world scenarios has been thoroughly validated.
更多查看译文
关键词
Deep Learning for Visual Perception,Computer Vision for Automation,Simulation and Animation
AI 理解论文
溯源树
样例
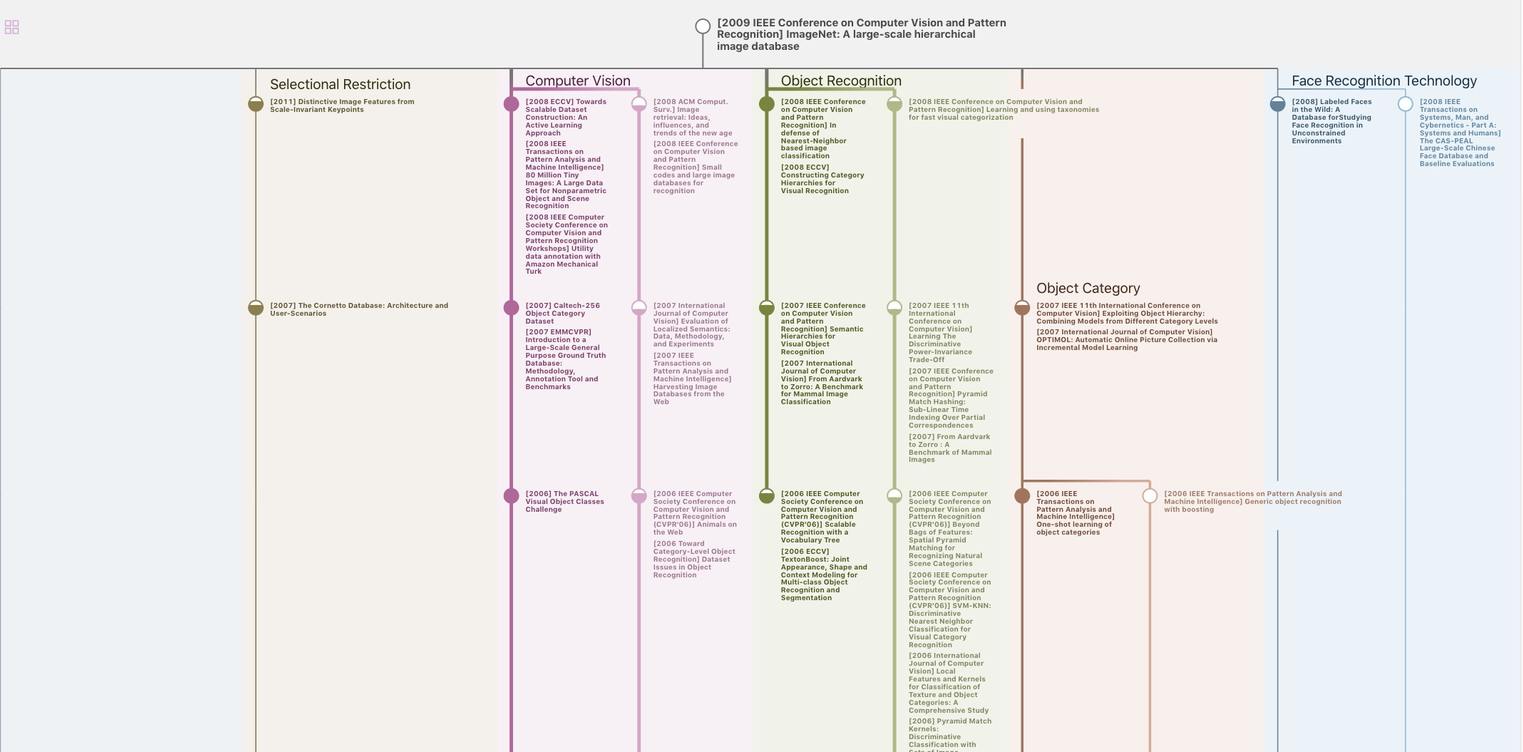
生成溯源树,研究论文发展脉络
数据免责声明
页面数据均来自互联网公开来源、合作出版商和通过AI技术自动分析结果,我们不对页面数据的有效性、准确性、正确性、可靠性、完整性和及时性做出任何承诺和保证。若有疑问,可以通过电子邮件方式联系我们:report@aminer.cn