An Efficient Generalizable Framework for Visuomotor Policies via Control-aware Augmentation and Privilege-guided Distillation
CoRR(2024)
摘要
Visuomotor policies, which learn control mechanisms directly from
high-dimensional visual observations, confront challenges in adapting to new
environments with intricate visual variations. Data augmentation emerges as a
promising method for bridging these generalization gaps by enriching data
variety. However, straightforwardly augmenting the entire observation shall
impose excessive burdens on policy learning and may even result in performance
degradation. In this paper, we propose to improve the generalization ability of
visuomotor policies as well as preserve training stability from two aspects: 1)
We learn a control-aware mask through a self-supervised reconstruction task
with three auxiliary losses and then apply strong augmentation only to those
control-irrelevant regions based on the mask to reduce the generalization gaps.
2) To address training instability issues prevalent in visual reinforcement
learning (RL), we distill the knowledge from a pretrained RL expert processing
low-level environment states, to the student visuomotor policy. The policy is
subsequently deployed to unseen environments without any further finetuning. We
conducted comparison and ablation studies across various benchmarks: the
DMControl Generalization Benchmark (DMC-GB), the enhanced Robot Manipulation
Distraction Benchmark (RMDB), and a specialized long-horizontal drawer-opening
robotic task. The extensive experimental results well demonstrate the
effectiveness of our method, e.g., showing a 17% improvement over previous
methods in the video-hard setting of DMC-GB.
更多查看译文
AI 理解论文
溯源树
样例
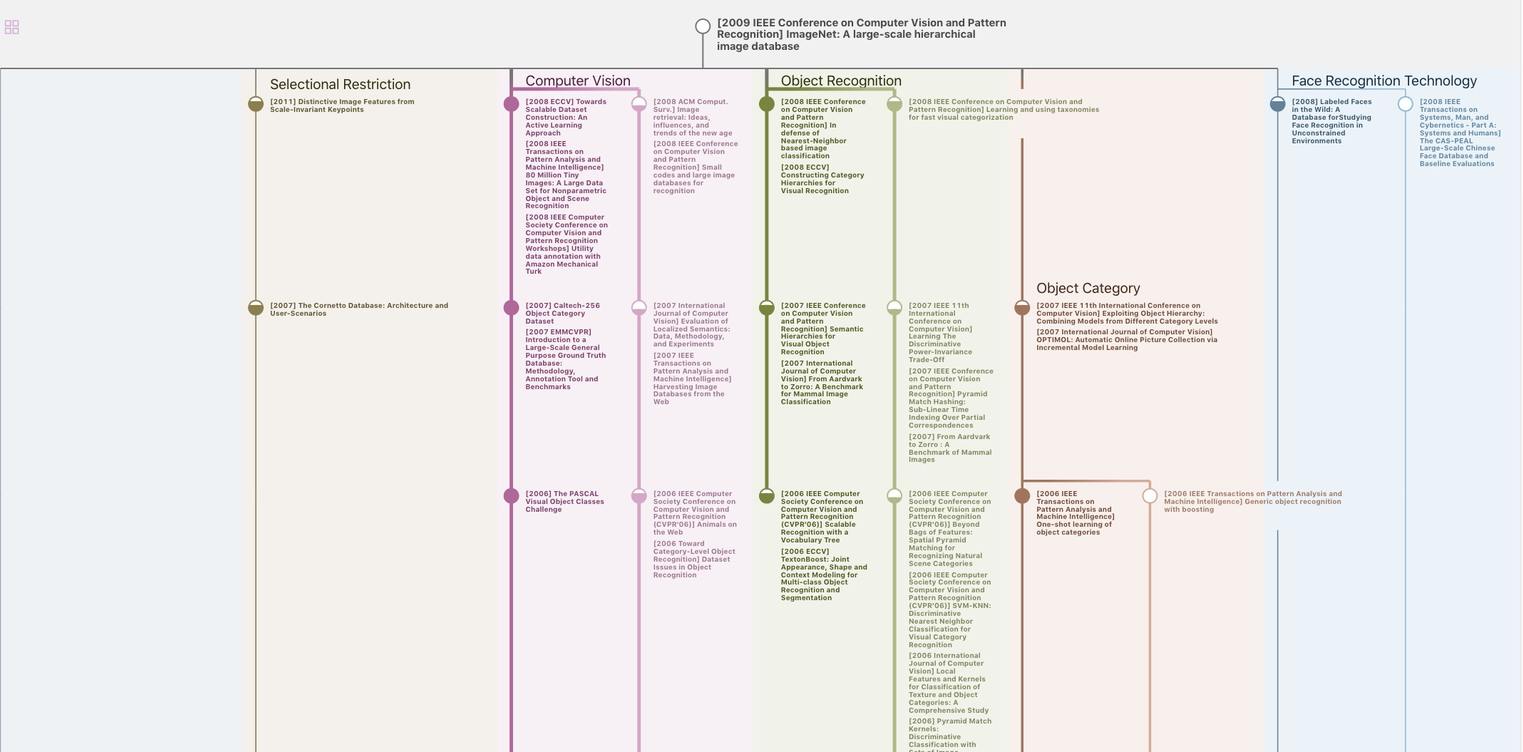
生成溯源树,研究论文发展脉络
数据免责声明
页面数据均来自互联网公开来源、合作出版商和通过AI技术自动分析结果,我们不对页面数据的有效性、准确性、正确性、可靠性、完整性和及时性做出任何承诺和保证。若有疑问,可以通过电子邮件方式联系我们:report@aminer.cn