Distributed Analytics for Big Data: A Survey
NEUROCOMPUTING(2024)
摘要
In recent years, a constant and fast information growing has characterized digital applications in the majority of real-life scenarios. Thus, a new information asset, namely Big Data, has been defined and lead to different challenges, mainly related to data storage, management and analysis. Focusing on the last challenge, several Big Data analytics techniques have been developed, based on Machine Learning and Deep Learning paradigms. When dealing with Big Data, traditional approaches often take a lot of time to produce even a single predictive model, due to the extremely high demand of computational resources. The design of approaches specifically oriented to Big Data is required to overcome these computational issues. Most solutions rely on the deployment of Big Data analytics infrastructures on a cluster of machines and/or on parallelization techniques. When deployment and parallelization apply to Machine Learning and Deep Learning, we can refer to the terms Distributed Machine Learning and Distributed Deep Learning, respectively. We here discuss the main principles and features of Distributed Machine Learning and Distributed Deep Learning frameworks. The main contribution of this work is a survey of solutions proposed in the literature, through the investigation of selected features and capabilities. In particular, the survey provides a comparative analysis according to the following classification criteria: implemented parallelization technique, supporting device, supported architecture, implemented communication mode, working mode, and class of algorithms. The paper also gives an overview of the most commonly used criteria and metrics for the performance evaluation of analyzed frameworks; finally, some emerging but promising optimization techniques are reviewed apart from our classification.
更多查看译文
关键词
Big data,Parallelization algorithms,Distributed machine learning,Distributed deep learning
AI 理解论文
溯源树
样例
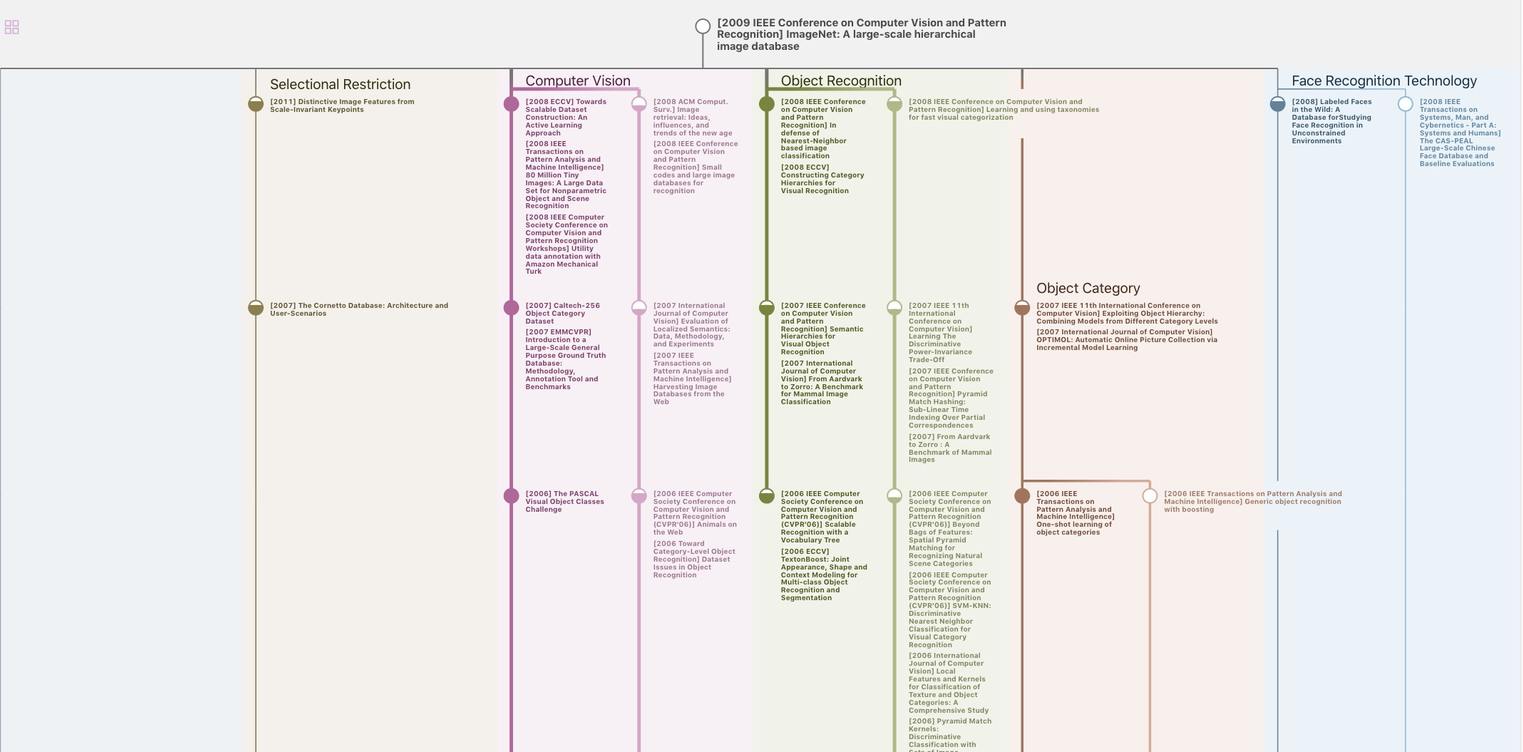
生成溯源树,研究论文发展脉络
数据免责声明
页面数据均来自互联网公开来源、合作出版商和通过AI技术自动分析结果,我们不对页面数据的有效性、准确性、正确性、可靠性、完整性和及时性做出任何承诺和保证。若有疑问,可以通过电子邮件方式联系我们:report@aminer.cn