WorldDreamer: Towards General World Models for Video Generation via Predicting Masked Tokens
CoRR(2024)
摘要
World models play a crucial role in understanding and predicting the dynamics
of the world, which is essential for video generation. However, existing world
models are confined to specific scenarios such as gaming or driving, limiting
their ability to capture the complexity of general world dynamic environments.
Therefore, we introduce WorldDreamer, a pioneering world model to foster a
comprehensive comprehension of general world physics and motions, which
significantly enhances the capabilities of video generation. Drawing
inspiration from the success of large language models, WorldDreamer frames
world modeling as an unsupervised visual sequence modeling challenge. This is
achieved by mapping visual inputs to discrete tokens and predicting the masked
ones. During this process, we incorporate multi-modal prompts to facilitate
interaction within the world model. Our experiments show that WorldDreamer
excels in generating videos across different scenarios, including natural
scenes and driving environments. WorldDreamer showcases versatility in
executing tasks such as text-to-video conversion, image-tovideo synthesis, and
video editing. These results underscore WorldDreamer's effectiveness in
capturing dynamic elements within diverse general world environments.
更多查看译文
AI 理解论文
溯源树
样例
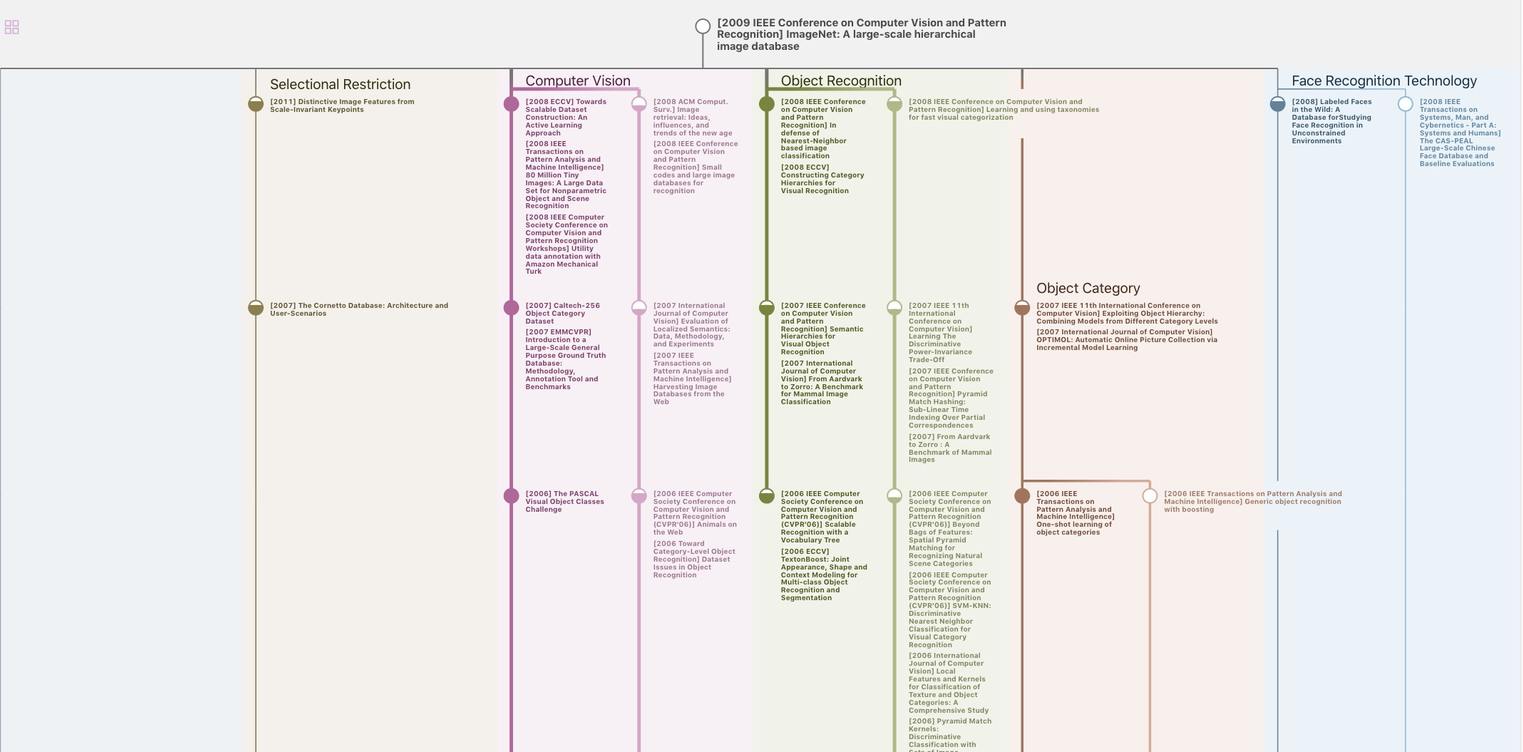
生成溯源树,研究论文发展脉络
数据免责声明
页面数据均来自互联网公开来源、合作出版商和通过AI技术自动分析结果,我们不对页面数据的有效性、准确性、正确性、可靠性、完整性和及时性做出任何承诺和保证。若有疑问,可以通过电子邮件方式联系我们:report@aminer.cn