MolPLA: A Molecular Pretraining Framework for Learning Cores, R-Groups and Their Linker Joints
BIOINFORMATICS(2024)
摘要
MOTIVATION:Molecular core structures and R-groups are essential concepts in drug development. Integration of these concepts with conventional graph pre-training approaches can promote deeper understanding in molecules. We propose MolPLA, a novel pre-training framework that employs masked graph contrastive learning in understanding the underlying decomposable parts in molecules that implicate their core structure and peripheral R-groups. Furthermore, we formulate an additional framework that grants MolPLA the ability to help chemists find replaceable R-groups in lead optimization scenarios. RESULTS:Experimental results on molecular property prediction show that MolPLA exhibits predictability comparable to current state-of-the-art models. Qualitative analysis implicate that MolPLA is capable of distinguishing core and R-group sub-structures, identifying decomposable regions in molecules and contributing to lead optimization scenarios by rationally suggesting R-group replacements given various query core templates. AVAILABILITY AND IMPLEMENTATION:The code implementation for MolPLA and its pre-trained model checkpoint is available at https://github.com/dmis-lab/MolPLA.
更多查看译文
关键词
Molecular Docking
AI 理解论文
溯源树
样例
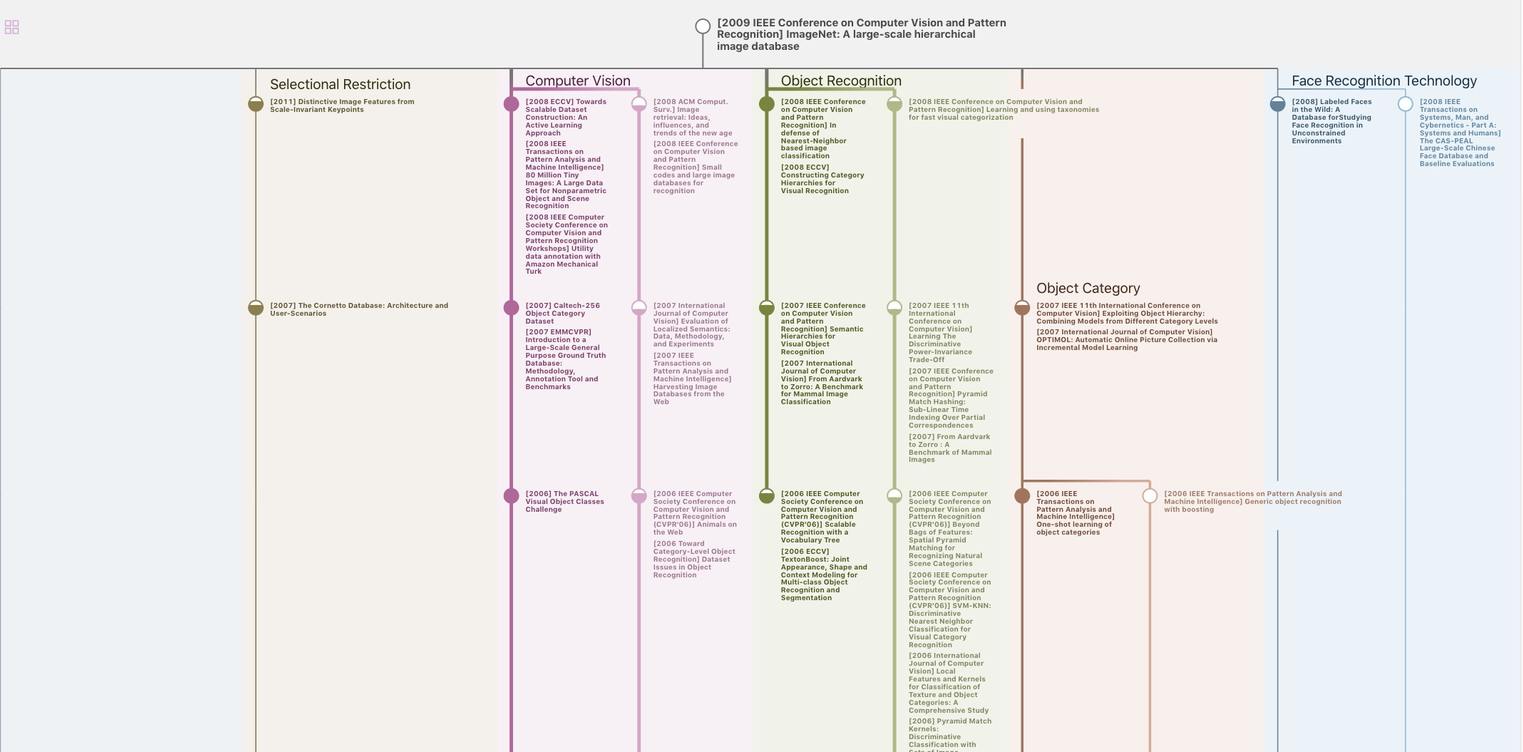
生成溯源树,研究论文发展脉络
数据免责声明
页面数据均来自互联网公开来源、合作出版商和通过AI技术自动分析结果,我们不对页面数据的有效性、准确性、正确性、可靠性、完整性和及时性做出任何承诺和保证。若有疑问,可以通过电子邮件方式联系我们:report@aminer.cn