Navigating the OverKill in Large Language Models
Proceedings of the 62nd Annual Meeting of the Association for Computational Linguistics (Volume 1 Long Papers)(2024)
摘要
Large language models are meticulously aligned to be both helpful andharmless. However, recent research points to a potential overkill which meansmodels may refuse to answer benign queries. In this paper, we investigate thefactors for overkill by exploring how models handle and determine the safety ofqueries. Our findings reveal the presence of shortcuts within models, leadingto an over-attention of harmful words like 'kill' and prompts emphasizingsafety will exacerbate overkill. Based on these insights, we introduceSelf-Contrastive Decoding (Self-CD), a training-free and model-agnosticstrategy, to alleviate this phenomenon. We first extract such over-attention byamplifying the difference in the model's output distributions when respondingto system prompts that either include or omit an emphasis on safety. Then wedetermine the final next-token predictions by downplaying the over-attentionfrom the model via contrastive decoding. Empirical results indicate that ourmethod has achieved an average reduction of the refusal rate by 20% whilehaving almost no impact on safety.
更多查看译文
AI 理解论文
溯源树
样例
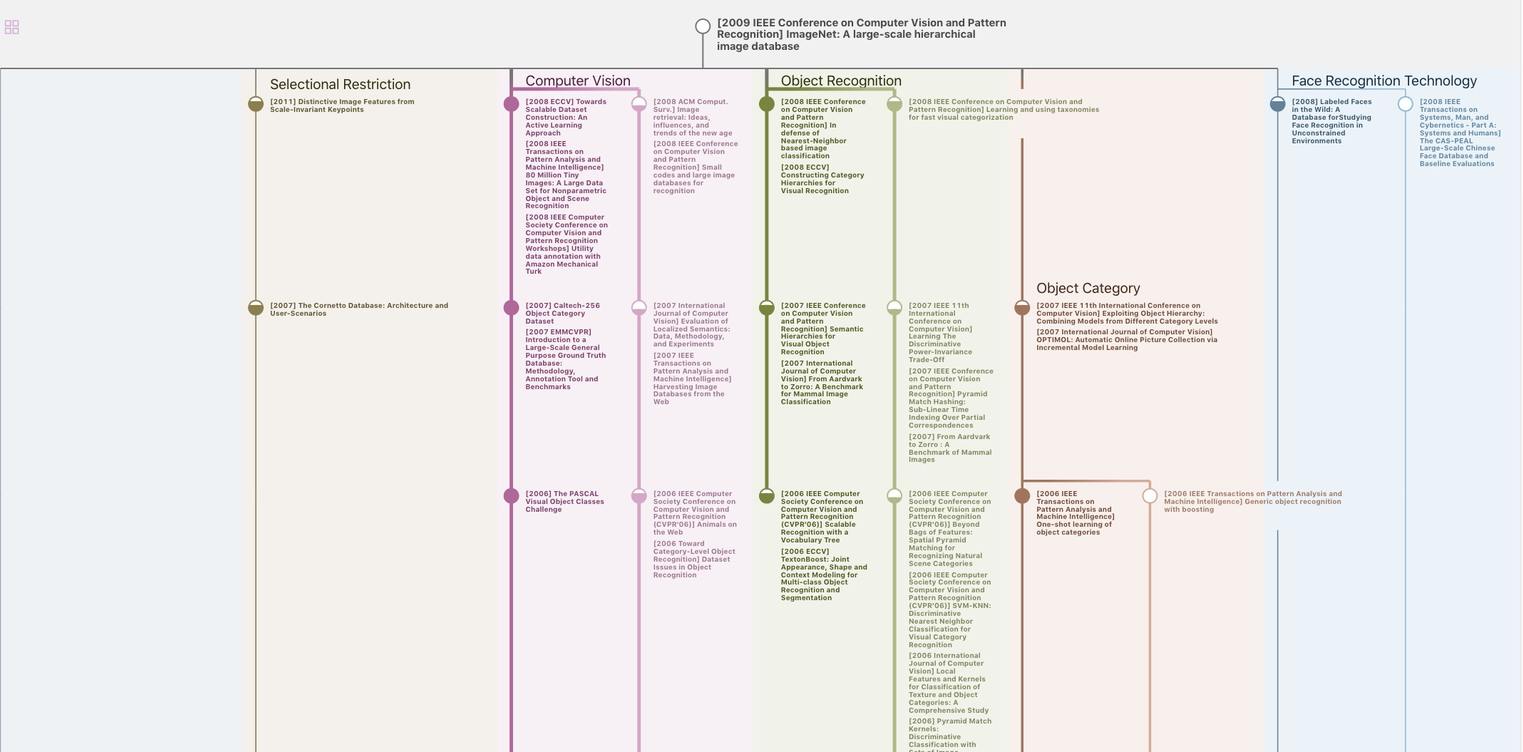
生成溯源树,研究论文发展脉络
数据免责声明
页面数据均来自互联网公开来源、合作出版商和通过AI技术自动分析结果,我们不对页面数据的有效性、准确性、正确性、可靠性、完整性和及时性做出任何承诺和保证。若有疑问,可以通过电子邮件方式联系我们:report@aminer.cn