EVA-GAN: Enhanced Various Audio Generation via Scalable Generative Adversarial Networks
CoRR(2024)
摘要
The advent of Large Models marks a new era in machine learning, significantly
outperforming smaller models by leveraging vast datasets to capture and
synthesize complex patterns. Despite these advancements, the exploration into
scaling, especially in the audio generation domain, remains limited, with
previous efforts didn't extend into the high-fidelity (HiFi) 44.1kHz domain and
suffering from both spectral discontinuities and blurriness in the
high-frequency domain, alongside a lack of robustness against out-of-domain
data. These limitations restrict the applicability of models to diverse use
cases, including music and singing generation. Our work introduces Enhanced
Various Audio Generation via Scalable Generative Adversarial Networks
(EVA-GAN), yields significant improvements over previous state-of-the-art in
spectral and high-frequency reconstruction and robustness in out-of-domain data
performance, enabling the generation of HiFi audios by employing an extensive
dataset of 36,000 hours of 44.1kHz audio, a context-aware module, a
Human-In-The-Loop artifact measurement toolkit, and expands the model to
approximately 200 million parameters. Demonstrations of our work are available
at https://double-blind-eva-gan.cc.
更多查看译文
AI 理解论文
溯源树
样例
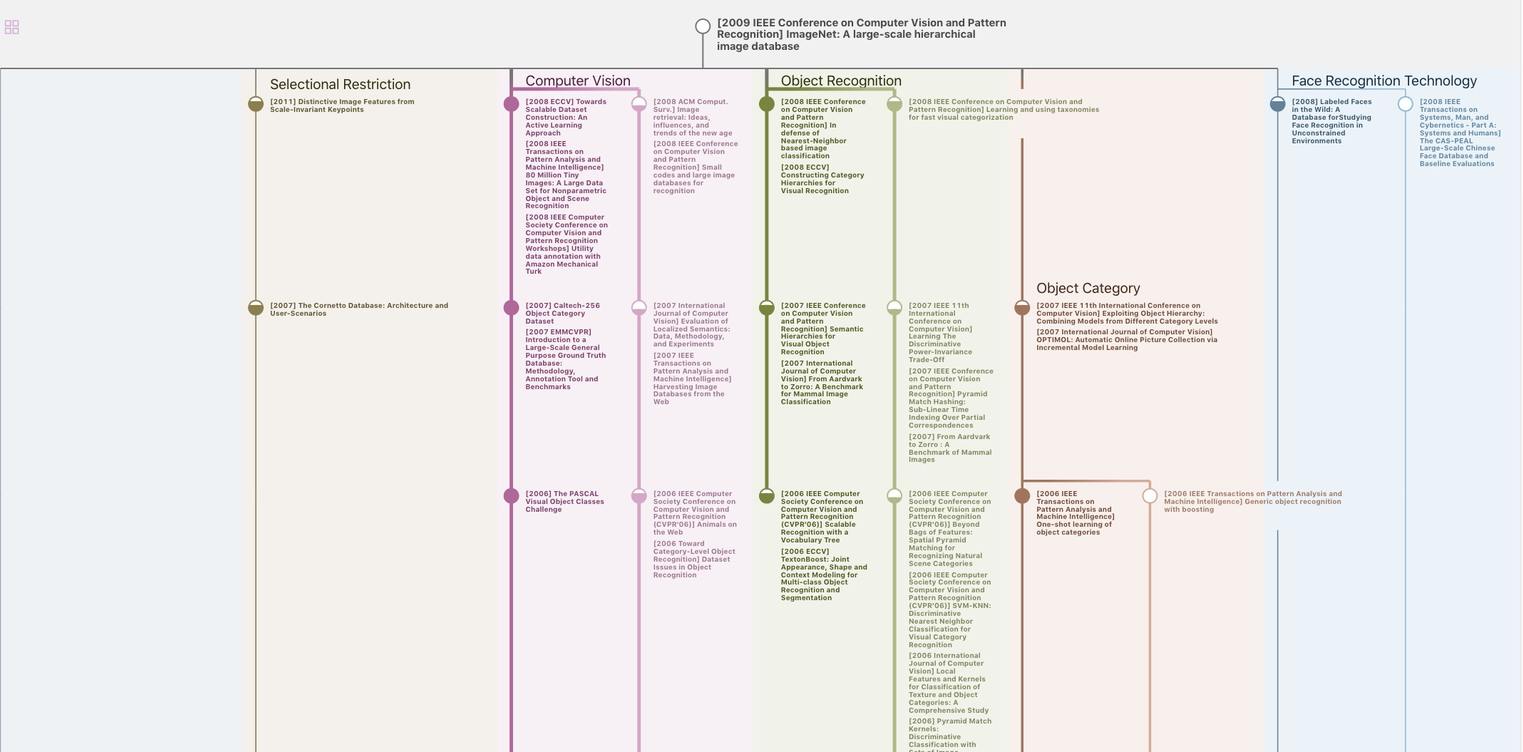
生成溯源树,研究论文发展脉络
数据免责声明
页面数据均来自互联网公开来源、合作出版商和通过AI技术自动分析结果,我们不对页面数据的有效性、准确性、正确性、可靠性、完整性和及时性做出任何承诺和保证。若有疑问,可以通过电子邮件方式联系我们:report@aminer.cn