AlphaRank: an Artificial Intelligence Approach for Ranking and Selection Problems.
CoRR(2024)
摘要
We introduce AlphaRank, an artificial intelligence approach to address thefixed-budget ranking and selection (R S) problems. We formulate the sequentialsampling decision as a Markov decision process and propose a Monte Carlosimulation-based rollout policy that utilizes classic R S procedures as basepolicies for efficiently learning the value function of stochastic dynamicprogramming. We accelerate online sample-allocation by using deep reinforcementlearning to pre-train a neural network model offline based on a given prior. Wealso propose a parallelizable computing framework for large-scale problems,effectively combining "divide and conquer" and "recursion" for enhancedscalability and efficiency. Numerical experiments demonstrate that theperformance of AlphaRank is significantly improved over the base policies,which could be attributed to AlphaRank's superior capability on the trade-offamong mean, variance, and induced correlation overlooked by many existingpolicies.
更多查看译文
AI 理解论文
溯源树
样例
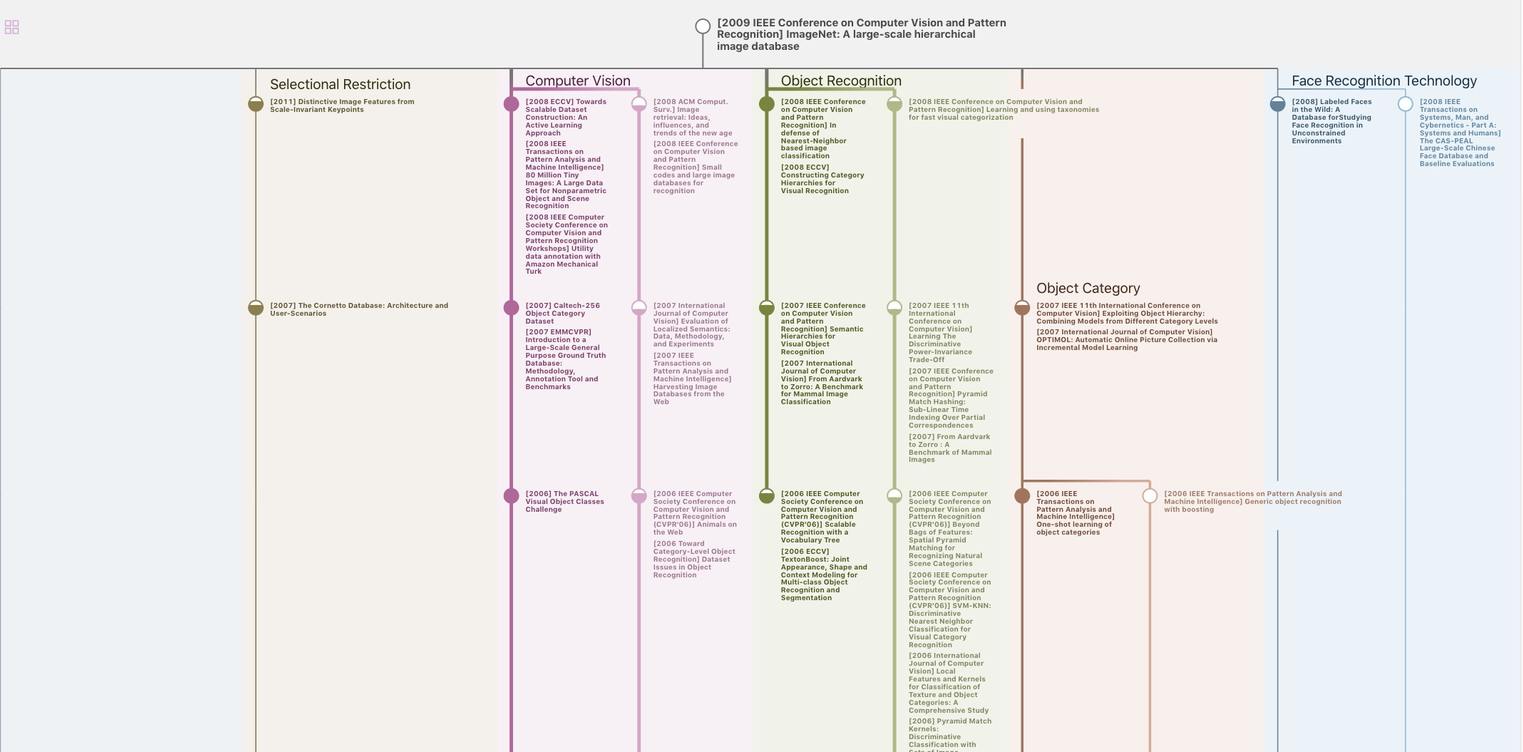
生成溯源树,研究论文发展脉络
数据免责声明
页面数据均来自互联网公开来源、合作出版商和通过AI技术自动分析结果,我们不对页面数据的有效性、准确性、正确性、可靠性、完整性和及时性做出任何承诺和保证。若有疑问,可以通过电子邮件方式联系我们:report@aminer.cn