Preference-Conditioned Language-Guided Abstraction
PROCEEDINGS OF THE 2024 ACM/IEEE INTERNATIONAL CONFERENCE ON HUMAN-ROBOT INTERACTION, HRI 2024(2024)
摘要
Learning from demonstrations is a common way for users to teach robots, butit is prone to spurious feature correlations. Recent work constructs stateabstractions, i.e. visual representations containing task-relevant features,from language as a way to perform more generalizable learning. However, theseabstractions also depend on a user's preference for what matters in a task,which may be hard to describe or infeasible to exhaustively specify usinglanguage alone. How do we construct abstractions to capture these latentpreferences? We observe that how humans behave reveals how they see the world.Our key insight is that changes in human behavior inform us that there aredifferences in preferences for how humans see the world, i.e. their stateabstractions. In this work, we propose using language models (LMs) to query forthose preferences directly given knowledge that a change in behavior hasoccurred. In our framework, we use the LM in two ways: first, given a textdescription of the task and knowledge of behavioral change between states, wequery the LM for possible hidden preferences; second, given the most likelypreference, we query the LM to construct the state abstraction. In thisframework, the LM is also able to ask the human directly when uncertain aboutits own estimate. We demonstrate our framework's ability to construct effectivepreference-conditioned abstractions in simulated experiments, a user study, aswell as on a real Spot robot performing mobile manipulation tasks.
更多查看译文
关键词
state abstraction,learning from human input,human preferences
AI 理解论文
溯源树
样例
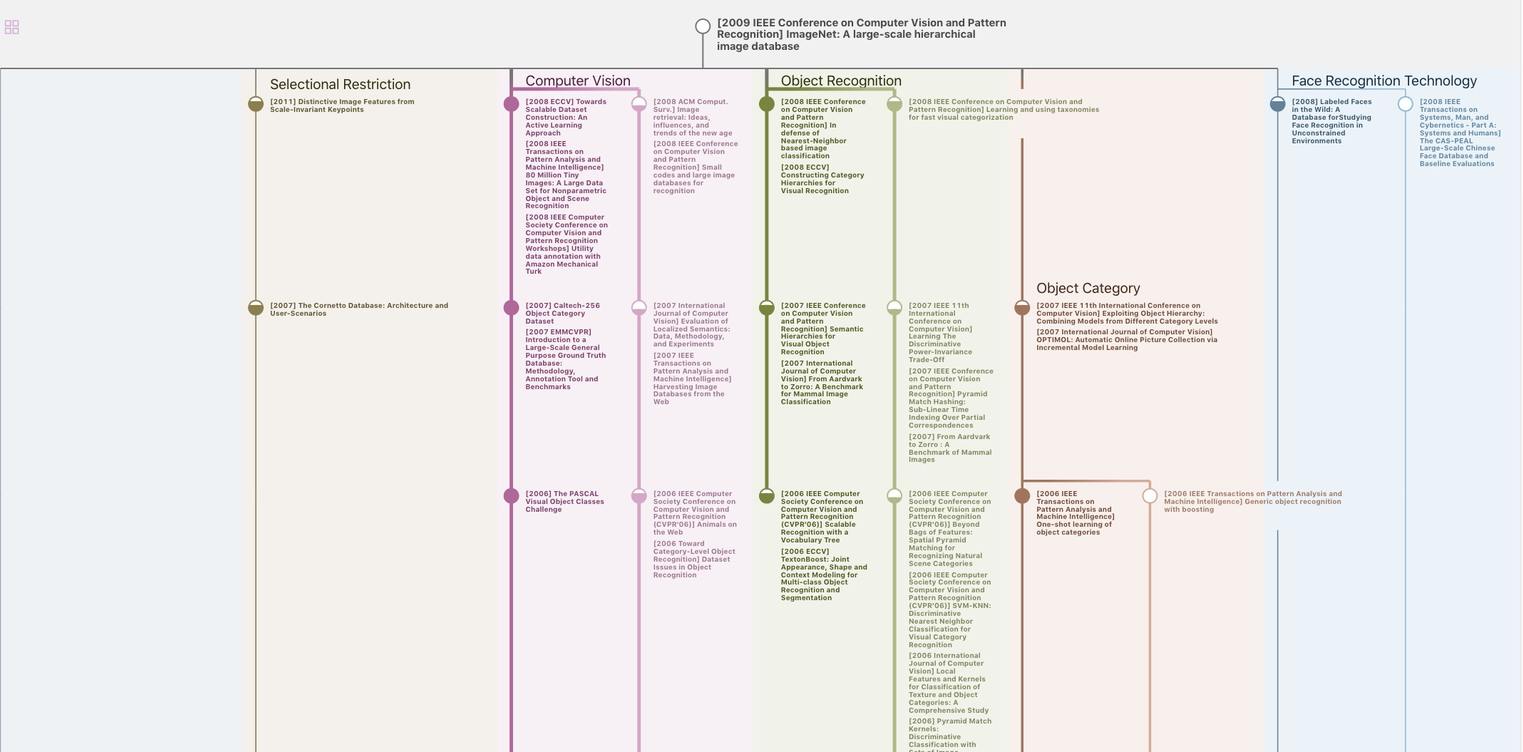
生成溯源树,研究论文发展脉络
数据免责声明
页面数据均来自互联网公开来源、合作出版商和通过AI技术自动分析结果,我们不对页面数据的有效性、准确性、正确性、可靠性、完整性和及时性做出任何承诺和保证。若有疑问,可以通过电子邮件方式联系我们:report@aminer.cn