Read to Play (R2-Play): Decision Transformer with Multimodal Game Instruction
CoRR(2024)
摘要
Developing a generalist agent is a longstanding objective in artificialintelligence. Previous efforts utilizing extensive offline datasets fromvarious tasks demonstrate remarkable performance in multitasking scenarioswithin Reinforcement Learning. However, these works encounter challenges inextending their capabilities to new tasks. Recent approaches integrate textualguidance or visual trajectory into decision networks to provide task-specificcontextual cues, representing a promising direction. However, it is observedthat relying solely on textual guidance or visual trajectory is insufficientfor accurately conveying the contextual information of tasks. This paperexplores enhanced forms of task guidance for agents, enabling them tocomprehend gameplay instructions, thereby facilitating a "read-to-play"capability. Drawing inspiration from the success of multimodal instructiontuning in visual tasks, we treat the visual-based RL task as a long-horizonvision task and construct a set of multimodal game instructions to incorporateinstruction tuning into a decision transformer. Experimental resultsdemonstrate that incorporating multimodal game instructions significantlyenhances the decision transformer's multitasking and generalizationcapabilities.
更多查看译文
AI 理解论文
溯源树
样例
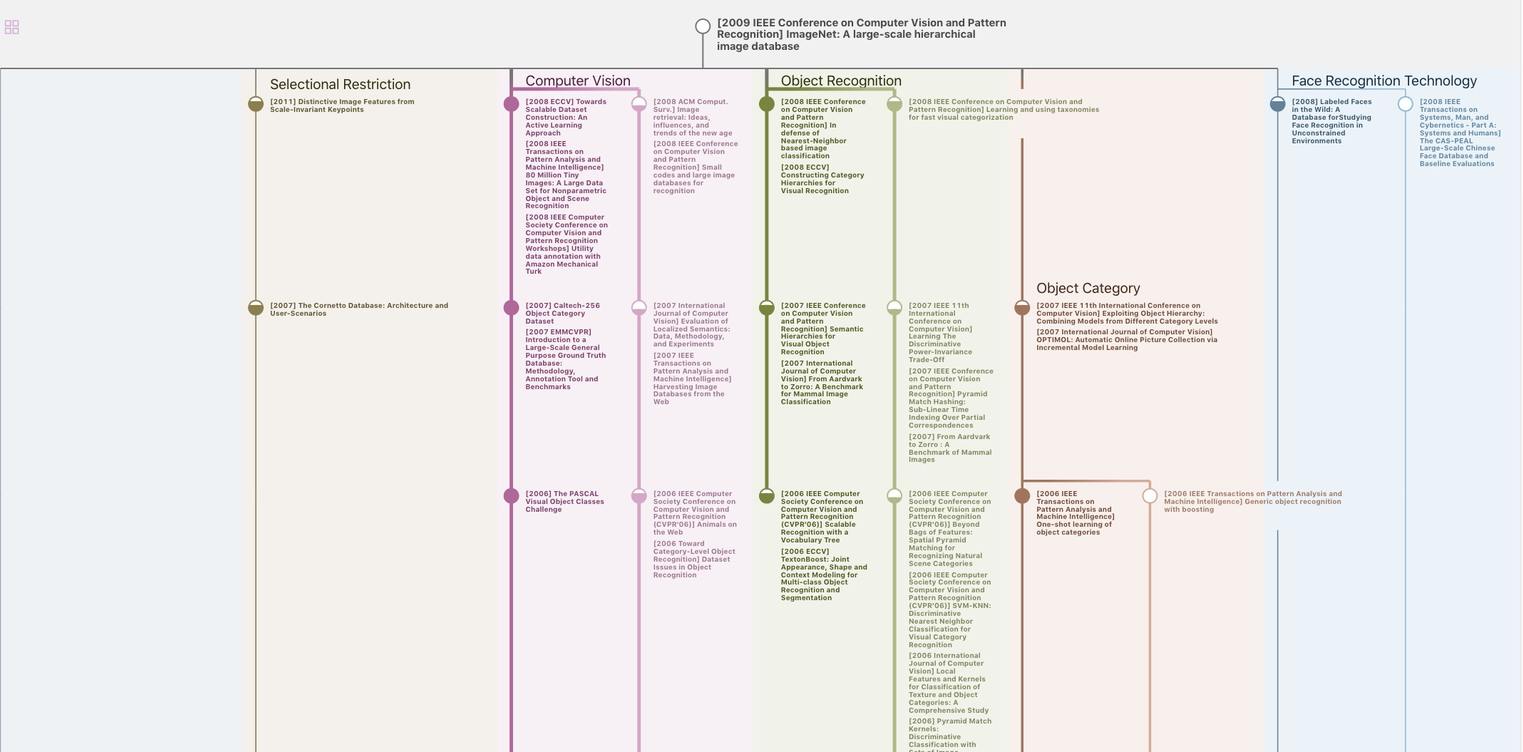
生成溯源树,研究论文发展脉络
数据免责声明
页面数据均来自互联网公开来源、合作出版商和通过AI技术自动分析结果,我们不对页面数据的有效性、准确性、正确性、可靠性、完整性和及时性做出任何承诺和保证。若有疑问,可以通过电子邮件方式联系我们:report@aminer.cn