COVID-19 Pandemic Simulation Modelling in Anaesthesia Residency Training to Predict Delays and Workforce Deficiencies: A Case Study of the Singapore Residency Training Program.
CUREUS JOURNAL OF MEDICAL SCIENCE(2024)
摘要
Background COVID-19 has been the worst pandemic of this century, resulting in economic, social, and educational disruptions. Residency training is no exception, with training restrictions delaying the progression and graduation of residents. We sought to utilize simulation modelling to predict the impact on future cohorts in the event of repeated and prolonged movement restrictions due to COVID-19 and future pandemics of a similar nature. Method A Delphi study was conducted to determine key Accreditation Council for Graduate Medical Education-International (ACGME-I) training variables affected by COVID-19. Quantitative resident datasets on these variables were collated and analysed from 2018 to 2021. Using the Vensim® software (Ventana Systems, Inc., Harvard, MA), historical resident data and pandemic progression delays were used to create a novel simulation model to predict future progression delay. Various durations of delay were also programmed into the software to simulate restrictions of varying severity that would impact resident progression. Results Using the model with scenarios simulating varying pandemic length, we found that the estimated average delay for residents in each accredited year ranged from an increase of one month for year 2 residents to more than three months for year 4 residents. Movement restrictions lasting a year would require up to six years before the program returned to a pre-pandemic equilibrium. Conclusion Systems dynamic modelling can be used to predict delays in residency training programs during a pandemic. The impact on the workforce can thus be projected, allowing residency programs to institute mitigating measures to avoid progression delay.
更多查看译文
关键词
simulation,modelling,anaesthesiology,residency,pandemic,covid-19
AI 理解论文
溯源树
样例
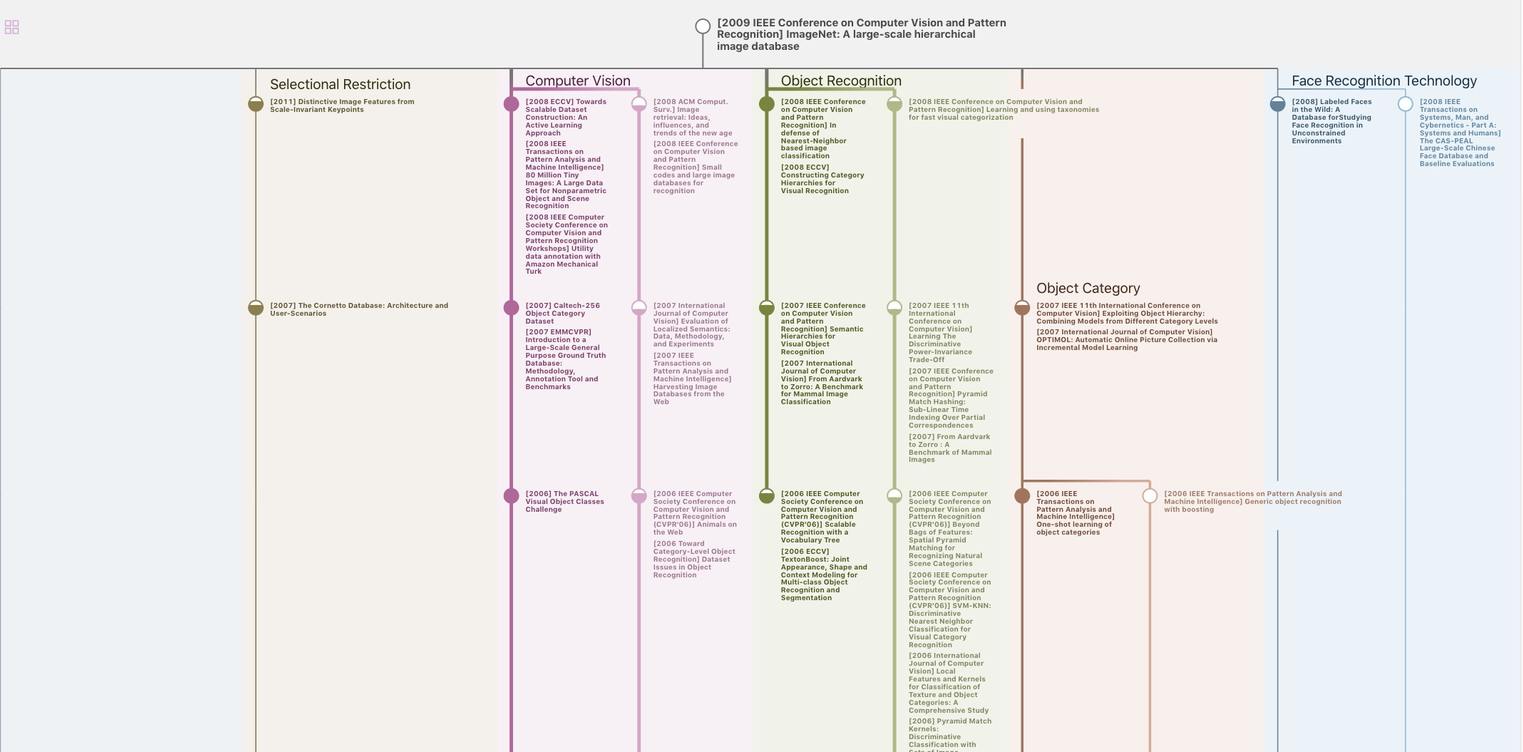
生成溯源树,研究论文发展脉络
数据免责声明
页面数据均来自互联网公开来源、合作出版商和通过AI技术自动分析结果,我们不对页面数据的有效性、准确性、正确性、可靠性、完整性和及时性做出任何承诺和保证。若有疑问,可以通过电子邮件方式联系我们:report@aminer.cn