AbsInstruct: Eliciting Abstraction Ability from LLMs Through Explanation Tuning with Plausibility Estimation
Proceedings of the 62nd Annual Meeting of the Association for Computational Linguistics (Volume 1 Long Papers)(2024)
摘要
Abstraction ability is crucial in human intelligence, which can also benefitvarious tasks in NLP study. Existing work shows that LLMs are deficient inabstract ability, and how to improve it remains unexplored. In this work, wedesign the framework AbsInstruct to enhance LLMs' abstraction ability throughinstruction tuning. The framework builds instructions with in-depthexplanations to assist LLMs in capturing the underlying rationale ofabstraction. Meanwhile, we introduce a plausibility estimator to selectinstructions that are more consistent with the abstraction knowledge of LLMs tobe aligned. Then, our framework combines abstraction instructions withgeneral-purpose ones to build a hybrid dataset. Extensive experiments andanalyses demonstrate that our framework can considerably enhance LLMs'abstraction ability with strong generalization performance while maintainingtheir general instruction-following abilities.
更多查看译文
AI 理解论文
溯源树
样例
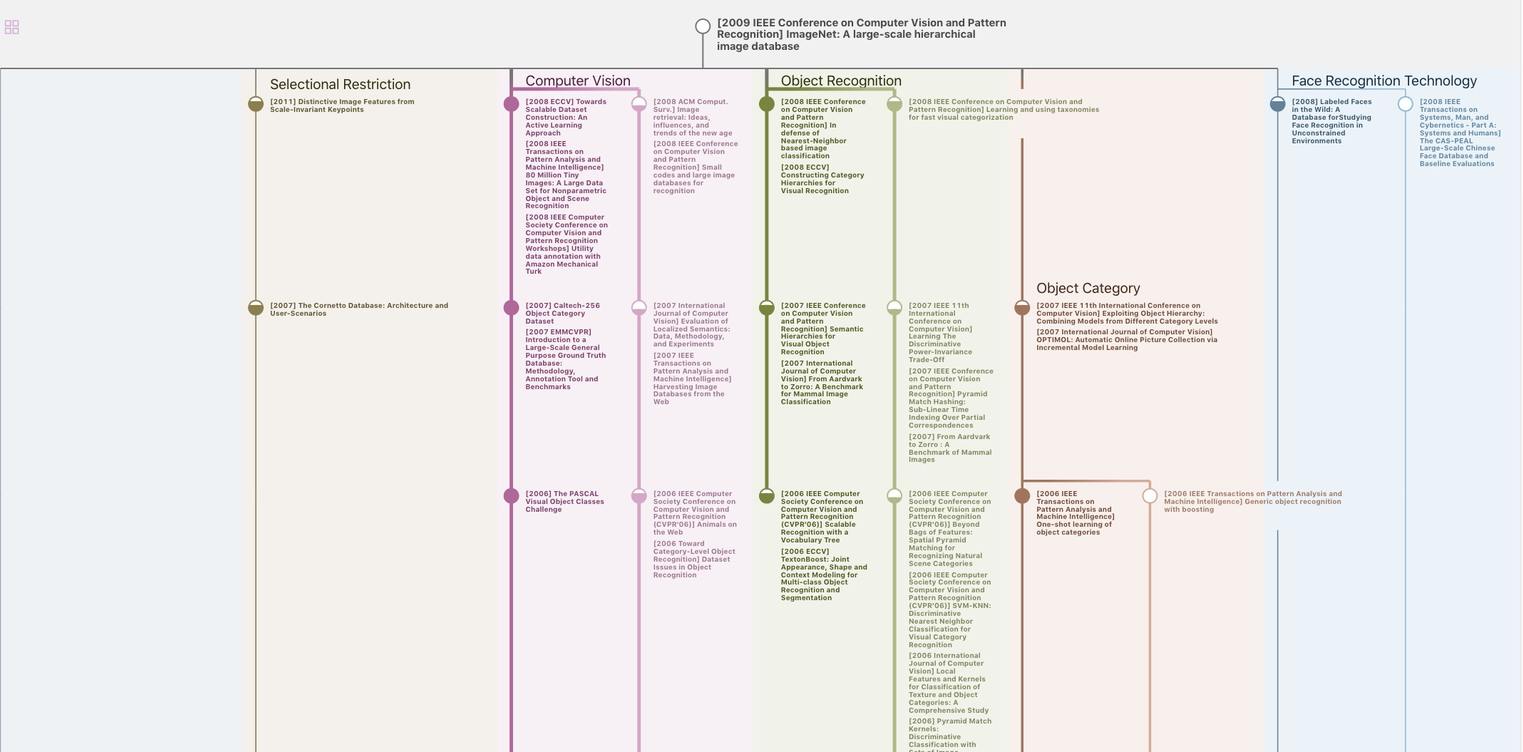
生成溯源树,研究论文发展脉络
数据免责声明
页面数据均来自互联网公开来源、合作出版商和通过AI技术自动分析结果,我们不对页面数据的有效性、准确性、正确性、可靠性、完整性和及时性做出任何承诺和保证。若有疑问,可以通过电子邮件方式联系我们:report@aminer.cn