Achieving Linear Speedup in Asynchronous Federated Learning with Heterogeneous Clients
IEEE Trans Mob Comput(2025)
摘要
Federated learning (FL) is an emerging distributed training paradigm thataims to learn a common global model without exchanging or transferring the datathat are stored locally at different clients. The Federated Averaging(FedAvg)-based algorithms have gained substantial popularity in FL to reducethe communication overhead, where each client conducts multiple localizediterations before communicating with a central server. In this paper, we focuson FL where the clients have diverse computation and/or communicationcapabilities. Under this circumstance, FedAvg can be less efficient since itrequires all clients that participate in the global aggregation in a round toinitiate iterations from the latest global model, and thus the synchronizationamong fast clients and straggler clients can severely slow down the overalltraining process. To address this issue, we propose an efficient asynchronousfederated learning (AFL) framework called Delayed Federated Averaging(DeFedAvg). In DeFedAvg, the clients are allowed to perform local training withdifferent stale global models at their own paces. Theoretical analysesdemonstrate that DeFedAvg achieves asymptotic convergence rates that are on parwith the results of FedAvg for solving nonconvex problems. More importantly,DeFedAvg is the first AFL algorithm that provably achieves the desirable linearspeedup property, which indicates its high scalability. Additionally, we carryout extensive numerical experiments using real datasets to validate theefficiency and scalability of our approach when training deep neural networks.
更多查看译文
关键词
Asynchronous federated learning,distributed optimization,edge machine learning,linear speedup,system heterogeneity
AI 理解论文
溯源树
样例
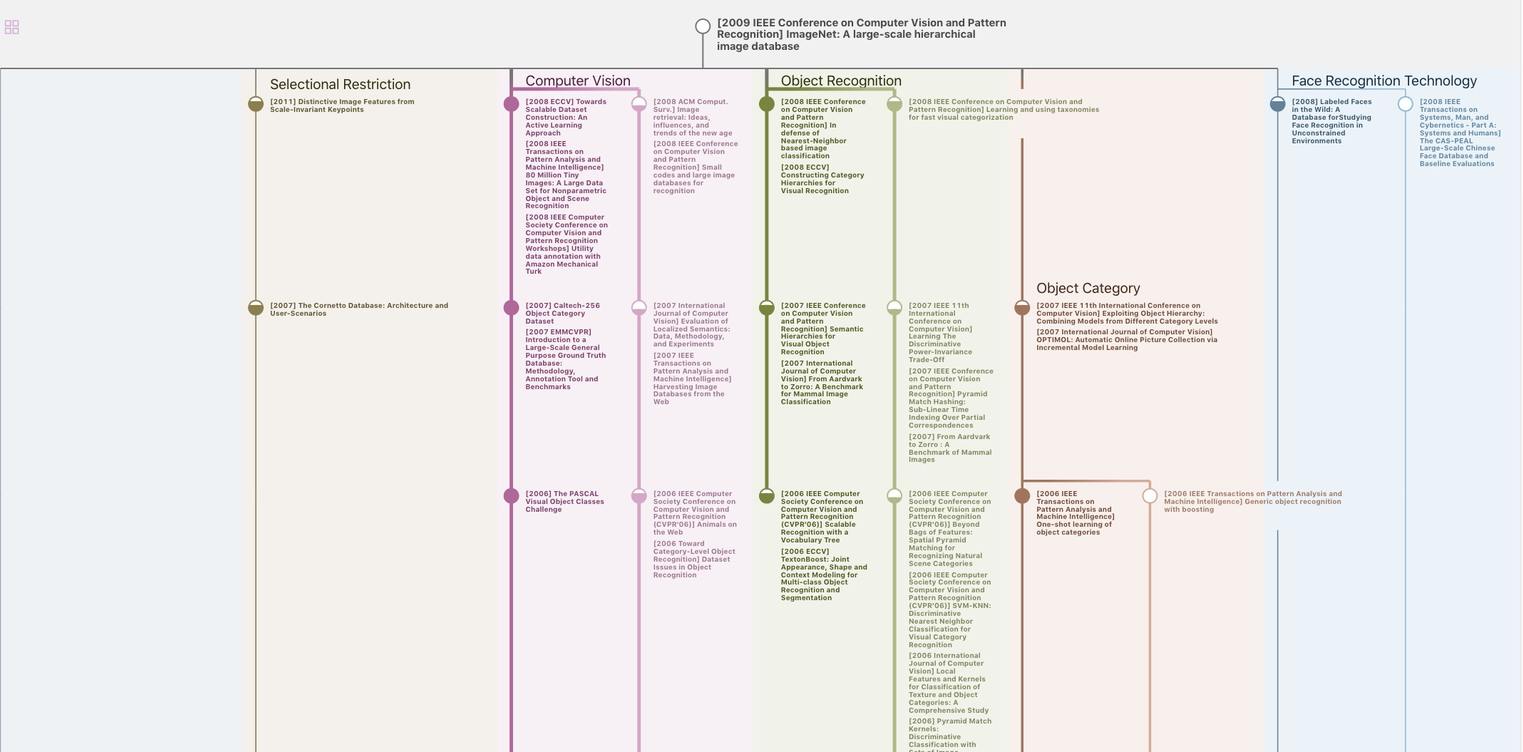
生成溯源树,研究论文发展脉络
数据免责声明
页面数据均来自互联网公开来源、合作出版商和通过AI技术自动分析结果,我们不对页面数据的有效性、准确性、正确性、可靠性、完整性和及时性做出任何承诺和保证。若有疑问,可以通过电子邮件方式联系我们:report@aminer.cn