Efficient and Effective Vocabulary Expansion Towards Multilingual Large Language Models
arXiv (Cornell University)(2024)
摘要
This report introduces , a Korean adaptation oflarge language models that exhibit remarkable capabilities across English andKorean text understanding. Building on recent highly capable butEnglish-centric LLMs, such as SOLAR-10.7B and Phi-2, where non-English textsare inefficiently processed with English-centric tokenizers, we present anefficient and effective vocabulary expansion (EEVE) method, which encompassesparameter freezing and subword initialization. In contrast to previous effortsthat believe new embeddings require trillions of training tokens, we show thatour method can significantly boost non-English proficiency within just 2billion tokens. Surpassing most instruction-tuned LLMs on the Open Ko-LLMLeaderboard, as of January 2024, our model ranks as the leading Korean pre-trained model in the open-source community,according to Hugging Face's leaderboard. We open-source our models onHuggingface to empower the open research community in various languages.
更多查看译文
关键词
End-to-End Speech Recognition,Language Modeling,Statistical Language Modeling,Neural Machine Translation,Named Entity Recognition
AI 理解论文
溯源树
样例
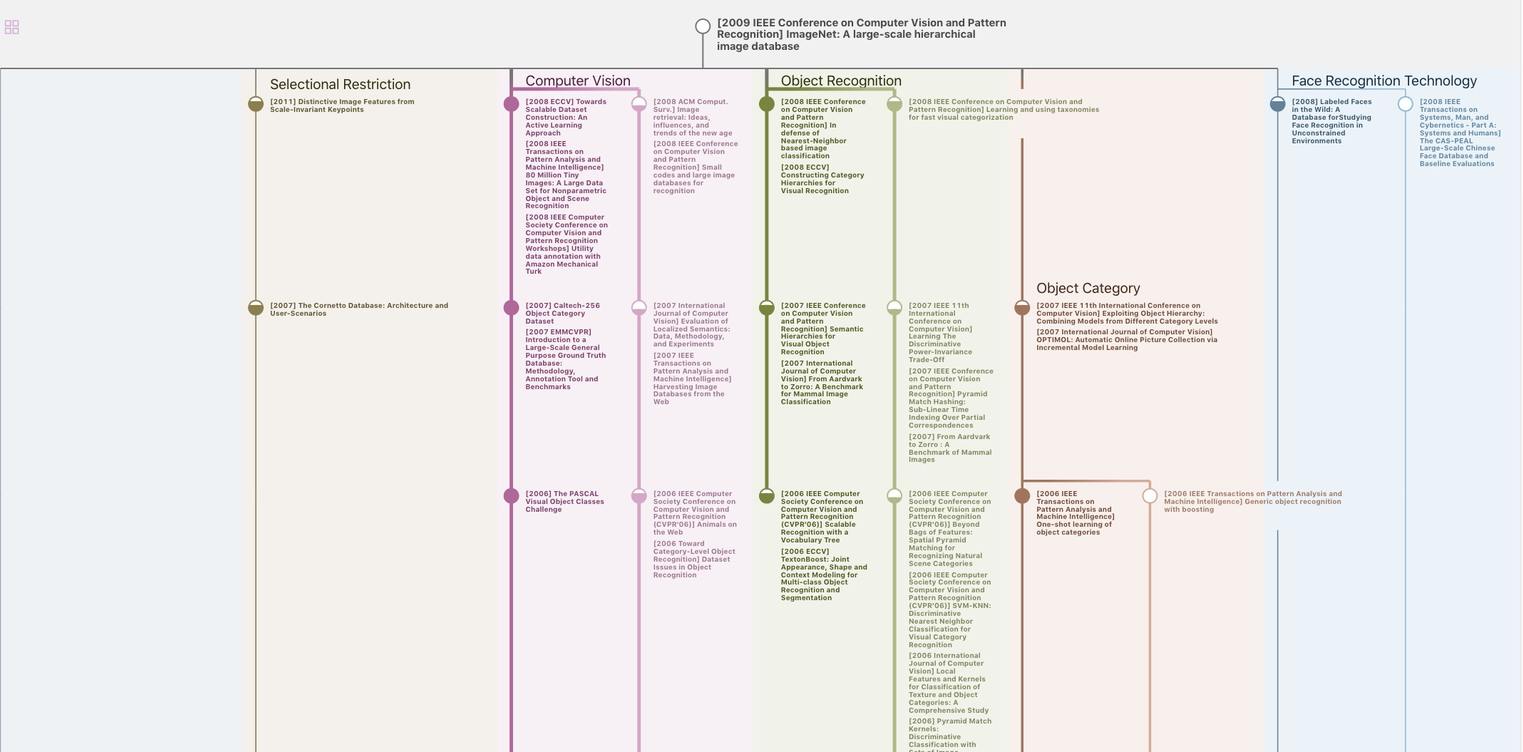
生成溯源树,研究论文发展脉络
数据免责声明
页面数据均来自互联网公开来源、合作出版商和通过AI技术自动分析结果,我们不对页面数据的有效性、准确性、正确性、可靠性、完整性和及时性做出任何承诺和保证。若有疑问,可以通过电子邮件方式联系我们:report@aminer.cn