Dual Flow Fusion Graph Convolutional Network for Traffic Flow Prediction
INTERNATIONAL JOURNAL OF MACHINE LEARNING AND CYBERNETICS(2024)
摘要
In recent decades, motor vehicle ownership has increased worldwide year by year, which causes that the accurate prediction of traffic flow on urban road networks becomes more important. However, the dual dependence on the micro layer and the macro layer creates a huge challenge for the prediction task. Previous models lack comprehensive analysis of the macro features at different time granularities. In this paper, we propose a novel Dual Flow Fusion Graph Convolutional Network (DFFGCN) to solve this problem. For capturing more macro features, we build the interactions between the micro layer and the macro layer at more time granularities. Then the spatial-temporal normalization model is introduced to separate the temporal and spatial influences. Therefore, the proposed DFFGCN has a better learning ability compared with other advanced models. Finally, we give experiments to show the effectiveness and superiority of our proposed model. Experimental results on three traffic datasets demonstrate that DFFGCN can achieve state-of-the-art performance consistently. And the ablation studies confirm the importance of each element of DFFGCN.
更多查看译文
关键词
Traffic flow forecasting,Deep learning,Spatio-temporal forecasting,Macro and micro information flow,Intelligent transportation system
AI 理解论文
溯源树
样例
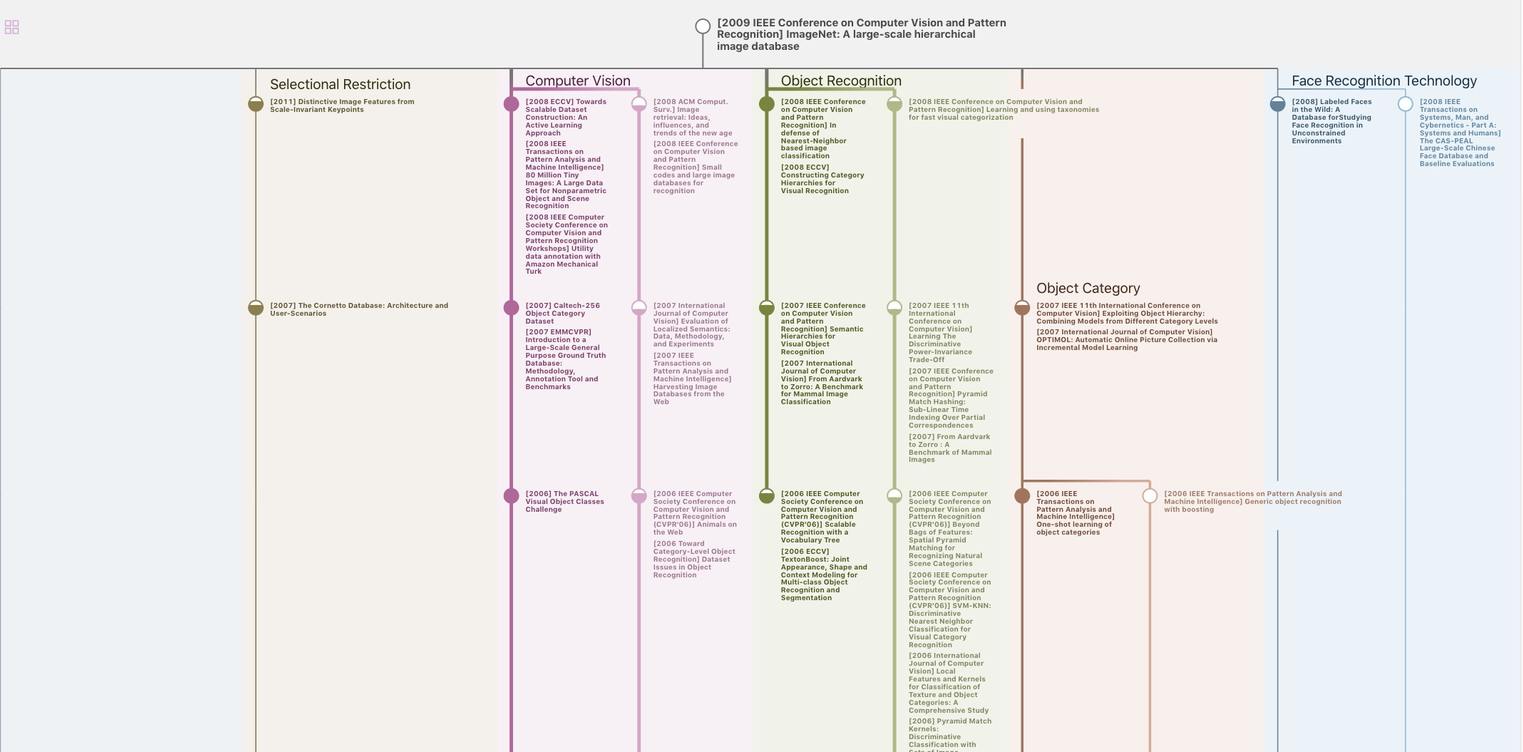
生成溯源树,研究论文发展脉络
数据免责声明
页面数据均来自互联网公开来源、合作出版商和通过AI技术自动分析结果,我们不对页面数据的有效性、准确性、正确性、可靠性、完整性和及时性做出任何承诺和保证。若有疑问,可以通过电子邮件方式联系我们:report@aminer.cn