BootTOD: Bootstrap Task-oriented Dialogue Representations by Aligning Diverse Responses
arXiv (Cornell University)(2024)
摘要
Pre-trained language models have been successful in many scenarios. However,their usefulness in task-oriented dialogues is limited due to the intrinsiclinguistic differences between general text and task-oriented dialogues.Current task-oriented dialogue pre-training methods rely on a contrastiveframework, which faces challenges such as selecting true positives and hardnegatives, as well as lacking diversity. In this paper, we propose a noveldialogue pre-training model called BootTOD. It learns task-oriented dialoguerepresentations via a self-bootstrapping framework. Unlike contrastivecounterparts, BootTOD aligns context and context+response representations anddismisses the requirements of contrastive pairs. BootTOD also uses multipleappropriate response targets to model the intrinsic one-to-many diversity ofhuman conversations. Experimental results show that BootTOD outperforms strongTOD baselines on diverse downstream dialogue tasks.
更多查看译文
关键词
Spoken Dialogue Systems,Language Modeling,Dialog Management,Topic Modeling,Multimodal Interaction
AI 理解论文
溯源树
样例
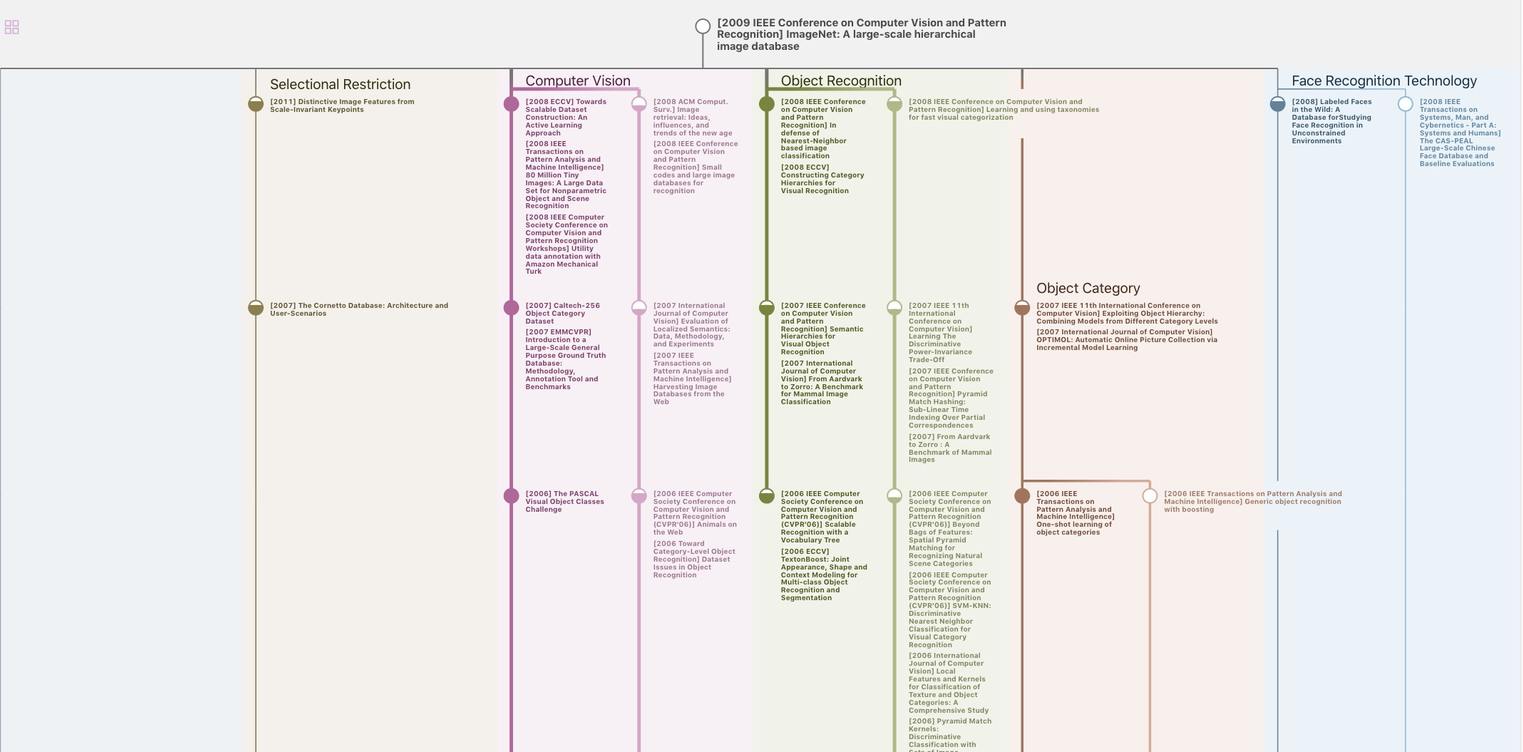
生成溯源树,研究论文发展脉络
数据免责声明
页面数据均来自互联网公开来源、合作出版商和通过AI技术自动分析结果,我们不对页面数据的有效性、准确性、正确性、可靠性、完整性和及时性做出任何承诺和保证。若有疑问,可以通过电子邮件方式联系我们:report@aminer.cn