FedDQA: A Novel Regularization-Based Deep Learning Method for Data Quality Assessment in Federated Learning
Decision Support Systems(2024)
摘要
Researchers strive to design artificial intelligence (AI) models that can fully utilize the potentials of data while protecting privacy. Federated learning is a promising solution because it utilizes data but shields them from those who do not own them. However, assessing data quality becomes a challenge in federated learning. We propose a data quality assessment method, Federated Data Quality Assessment (FedDQA), and compare it with traditional federated learning methods. FedDQA identifies low-quality data from participants and reduces their influence on the global model. We integrate data quality regularization strategies at the instance, feature, and participant levels into federate learning model. In various data poisoning settings, FedDQA outperforms existing federated learning methods in prediction performance and the accuracy in detecting low-quality data.
更多查看译文
关键词
Federated learning,Data quality,Data heterogeneity,Regularization-based strategies
AI 理解论文
溯源树
样例
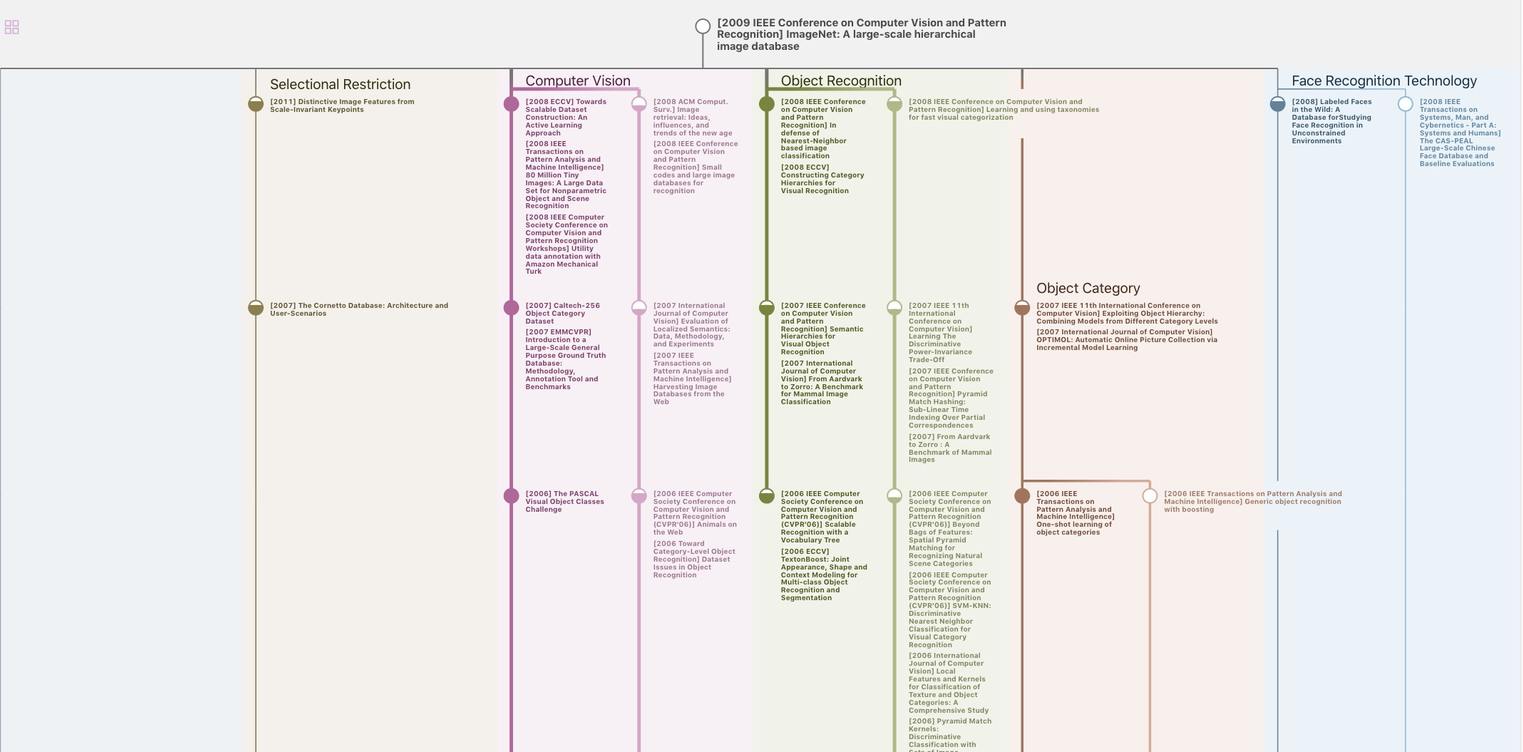
生成溯源树,研究论文发展脉络
数据免责声明
页面数据均来自互联网公开来源、合作出版商和通过AI技术自动分析结果,我们不对页面数据的有效性、准确性、正确性、可靠性、完整性和及时性做出任何承诺和保证。若有疑问,可以通过电子邮件方式联系我们:report@aminer.cn