Soft Reasoning on Uncertain Knowledge Graphs
arXiv (Cornell University)(2024)
摘要
The study of machine learning-based logical query-answering enables reasoningwith large-scale and incomplete knowledge graphs. This paper further advancesthis line of research by considering the uncertainty in the knowledge. Theuncertain nature of knowledge is widely observed in the real world, butdoes not align seamlessly with the first-order logic underpinningexisting studies. To bridge this gap, we study the setting of soft queries onuncertain knowledge, which is motivated by the establishment of soft constraintprogramming. We further propose an ML-based approach with both forwardinference and backward calibration to answer soft queries on large-scale,incomplete, and uncertain knowledge graphs. Theoretical discussions presentthat our methods share the same complexity as state-of-the-art inferencealgorithms for first-order queries. Empirical results justify the superiorperformance of our approach against previous ML-based methods with numberembedding extensions.
更多查看译文
关键词
Group Decision Making,Probabilistic Rough Sets,Decision Analysis,Information Granulation
AI 理解论文
溯源树
样例
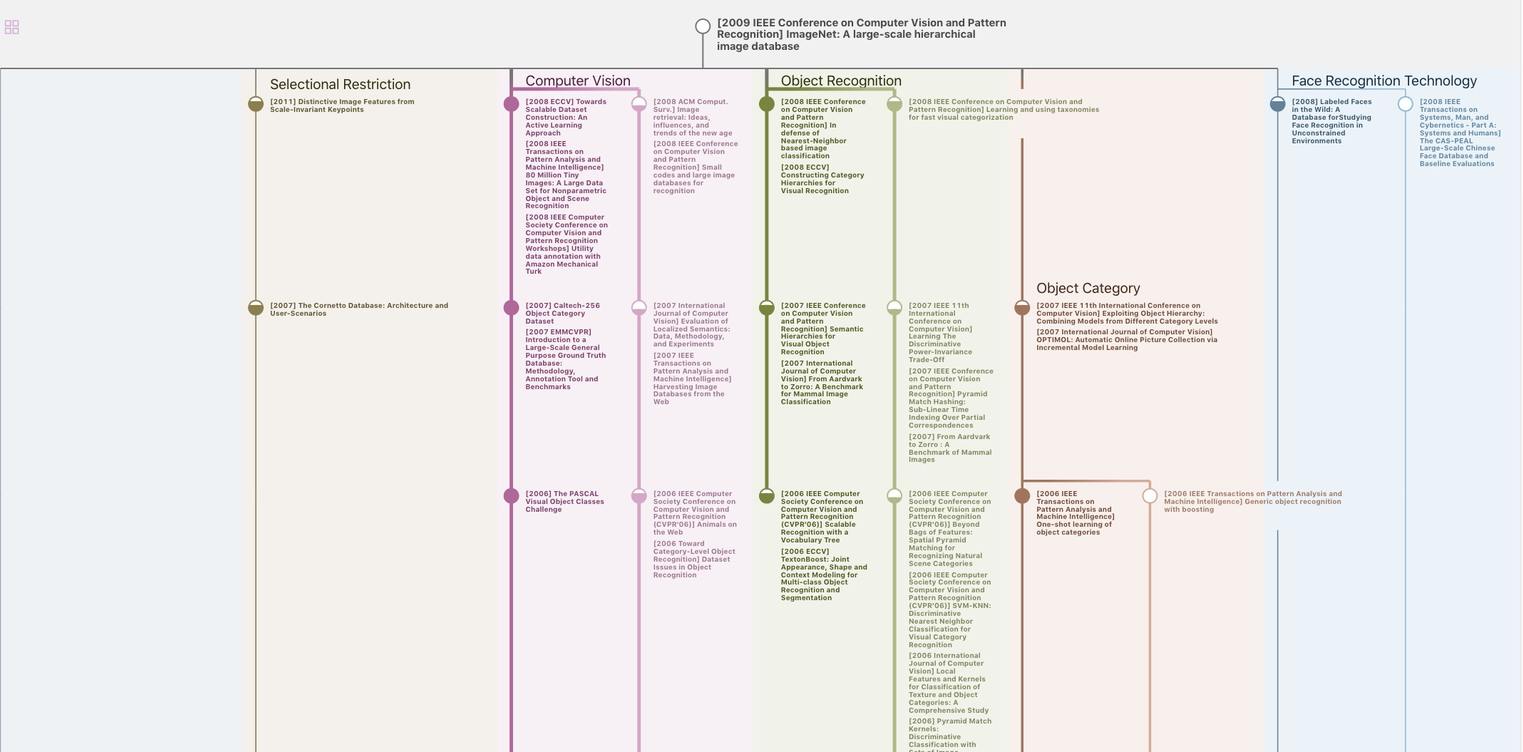
生成溯源树,研究论文发展脉络
数据免责声明
页面数据均来自互联网公开来源、合作出版商和通过AI技术自动分析结果,我们不对页面数据的有效性、准确性、正确性、可靠性、完整性和及时性做出任何承诺和保证。若有疑问,可以通过电子邮件方式联系我们:report@aminer.cn