Aligners: Decoupling LLMs and Alignment
Conference on Empirical Methods in Natural Language Processing(2024)
摘要
Large Language Models (LLMs) need to be aligned with human expectations toensure their safety and utility in most applications. Alignment is challenging,costly, and needs to be repeated for every LLM and alignment criterion. Wepropose to decouple LLMs and alignment by training aligner models that can beused to align any LLM for a given criteria on an as-needed basis, thus alsoreducing the potential negative impacts of alignment on performance. Our recipefor training the aligner models solely relies on synthetic data generated witha (prompted) LLM and can be easily adjusted for a variety of alignmentcriteria. We illustrate our method by training an "ethical" aligner and verifyits efficacy empirically.
更多查看译文
关键词
Interoperable
AI 理解论文
溯源树
样例
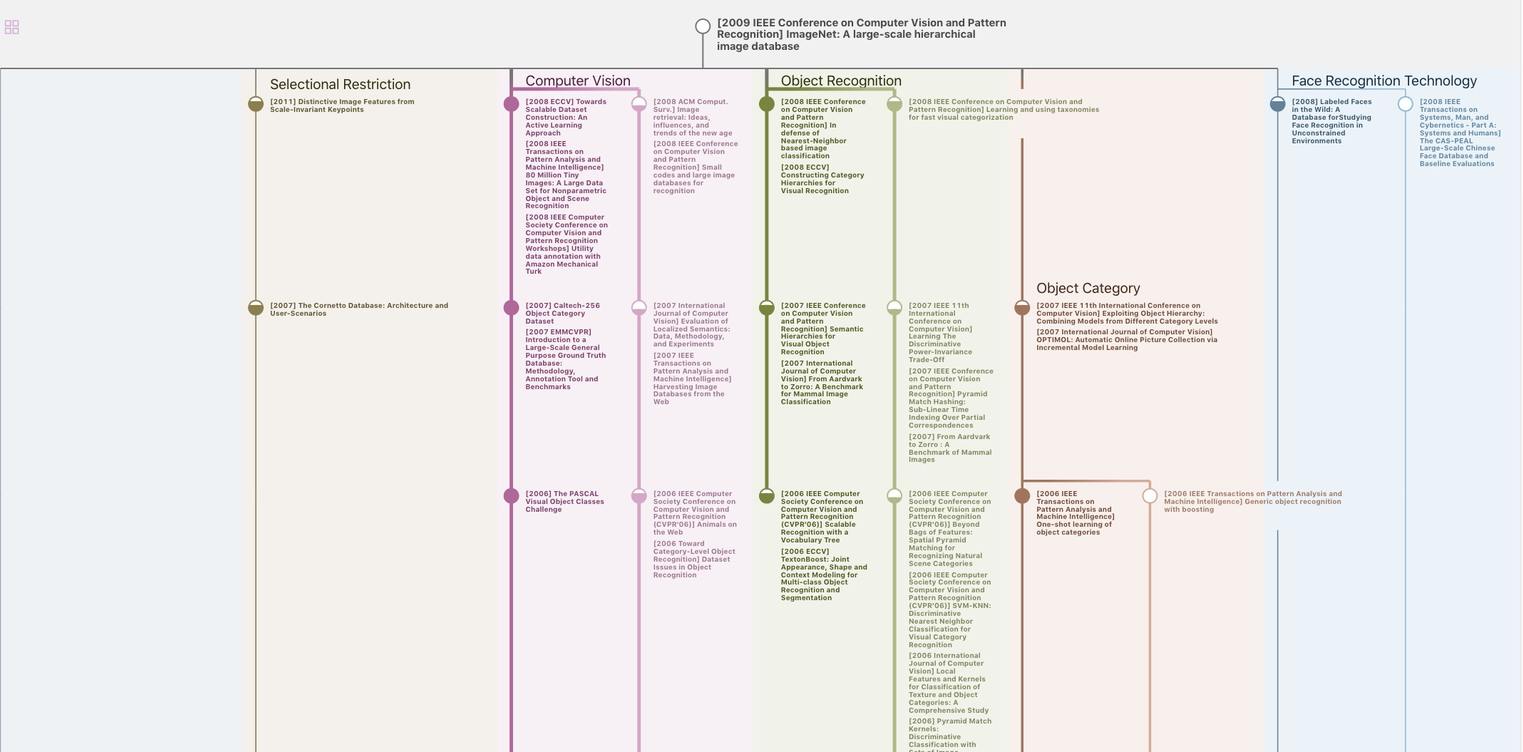
生成溯源树,研究论文发展脉络
数据免责声明
页面数据均来自互联网公开来源、合作出版商和通过AI技术自动分析结果,我们不对页面数据的有效性、准确性、正确性、可靠性、完整性和及时性做出任何承诺和保证。若有疑问,可以通过电子邮件方式联系我们:report@aminer.cn