Pearl: A Review-driven Persona-Knowledge Grounded Conversational Recommendation Dataset
arXiv (Cornell University)(2024)
摘要
Conversational recommender system is an emerging area that has garnered anincreasing interest in the community, especially with the advancements in largelanguage models (LLMs) that enable diverse reasoning over conversational input.Despite the progress, the field has many aspects left to explore. The currentlyavailable public datasets for conversational recommendation lack specific userpreferences and explanations for recommendations, hindering high-qualityrecommendations. To address such challenges, we present a novel conversationalrecommendation dataset named PEARL, synthesized with persona- andknowledge-augmented LLM simulators. We obtain detailed persona and knowledgefrom real-world reviews and construct a large-scale dataset with over 57kdialogues. Our experimental results demonstrate that utterances in PEARLinclude more specific user preferences, show expertise in the target domain,and provide recommendations more relevant to the dialogue context than those inprior datasets.
更多查看译文
关键词
Empathy Mapping
AI 理解论文
溯源树
样例
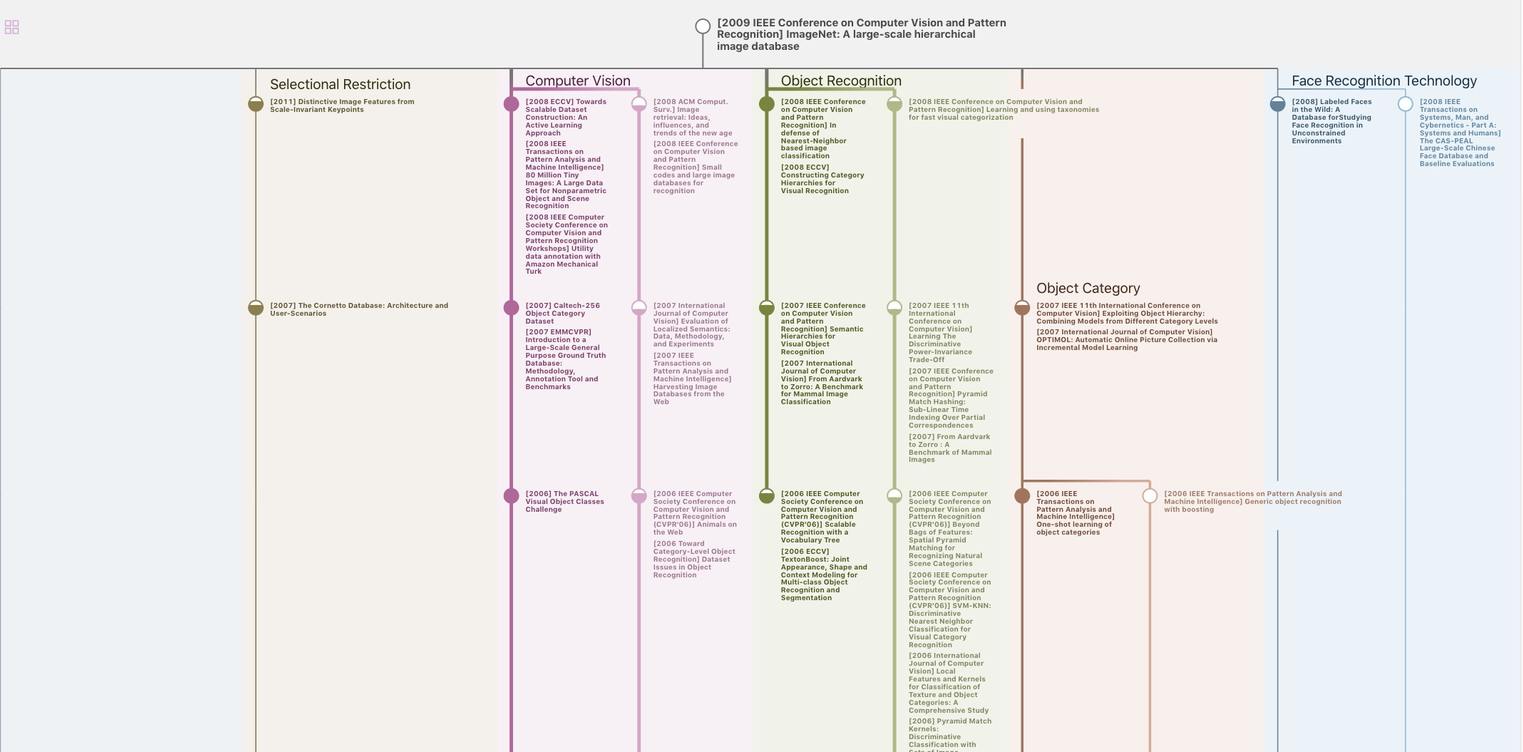
生成溯源树,研究论文发展脉络
数据免责声明
页面数据均来自互联网公开来源、合作出版商和通过AI技术自动分析结果,我们不对页面数据的有效性、准确性、正确性、可靠性、完整性和及时性做出任何承诺和保证。若有疑问,可以通过电子邮件方式联系我们:report@aminer.cn