Understanding Subjectivity through the Lens of Motivational Context in Model-Generated Image Satisfaction
CoRR(2024)
摘要
Image generation models are poised to become ubiquitous in a range of
applications. These models are often fine-tuned and evaluated using human
quality judgments that assume a universal standard, failing to consider the
subjectivity of such tasks. To investigate how to quantify subjectivity, and
the scale of its impact, we measure how assessments differ among human
annotators across different use cases. Simulating the effects of ordinarily
latent elements of annotators subjectivity, we contrive a set of motivations
(t-shirt graphics, presentation visuals, and phone background images) to
contextualize a set of crowdsourcing tasks. Our results show that human
evaluations of images vary within individual contexts and across combinations
of contexts. Three key factors affecting this subjectivity are image
appearance, image alignment with text, and representation of objects mentioned
in the text. Our study highlights the importance of taking individual users and
contexts into account, both when building and evaluating generative models
更多查看译文
AI 理解论文
溯源树
样例
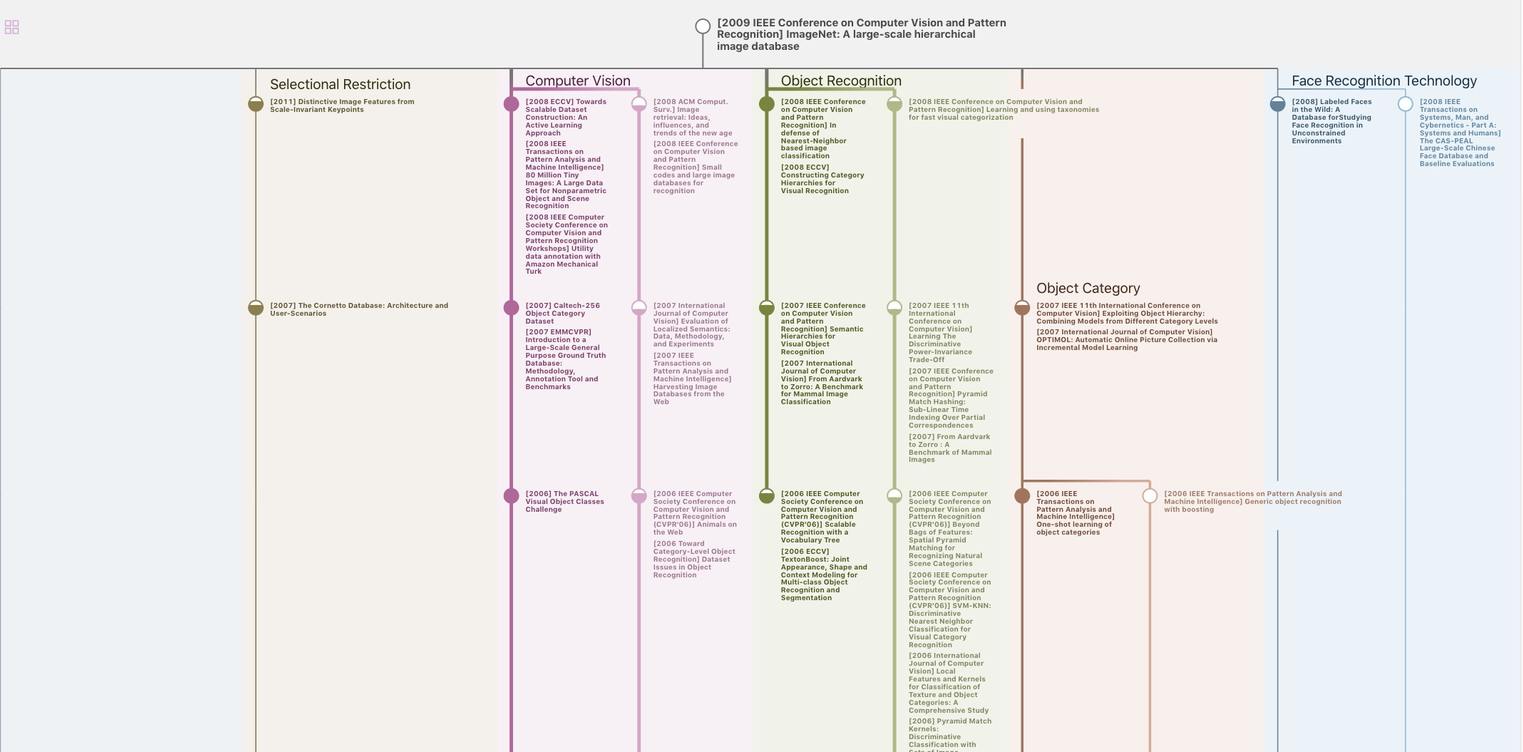
生成溯源树,研究论文发展脉络
数据免责声明
页面数据均来自互联网公开来源、合作出版商和通过AI技术自动分析结果,我们不对页面数据的有效性、准确性、正确性、可靠性、完整性和及时性做出任何承诺和保证。若有疑问,可以通过电子邮件方式联系我们:report@aminer.cn