Dataset Condensation for Time Series Classification Via Dual Domain Matching
KDD(2024)
摘要
Time series data has been demonstrated to be crucial in various researchfields. The management of large quantities of time series data presentschallenges in terms of deep learning tasks, particularly for training a deepneural network. Recently, a technique named Dataset Condensation hasemerged as a solution to this problem. This technique generates a smallersynthetic dataset that has comparable performance to the full real dataset indownstream tasks such as classification. However, previous methods areprimarily designed for image and graph datasets, and directly adapting them tothe time series dataset leads to suboptimal performance due to their inabilityto effectively leverage the rich information inherent in time series data,particularly in the frequency domain. In this paper, we propose a novelframework named Dataset Condensation forTime SeriesClassification via Dual Domain Matching (CondTSC)which focuses on the time series classification dataset condensation task.Different from previous methods, our proposed framework aims to generate acondensed dataset that matches the surrogate objectives in both the time andfrequency domains. Specifically, CondTSC incorporates multi-view dataaugmentation, dual domain training, and dual surrogate objectives to enhancethe dataset condensation process in the time and frequency domains. Throughextensive experiments, we demonstrate the effectiveness of our proposedframework, which outperforms other baselines and learns a condensed syntheticdataset that exhibits desirable characteristics such as conforming to thedistribution of the original data.
更多查看译文
关键词
Dimensionality Reduction,Dynamic Time Warping,Clustering,Deep Learning
AI 理解论文
溯源树
样例
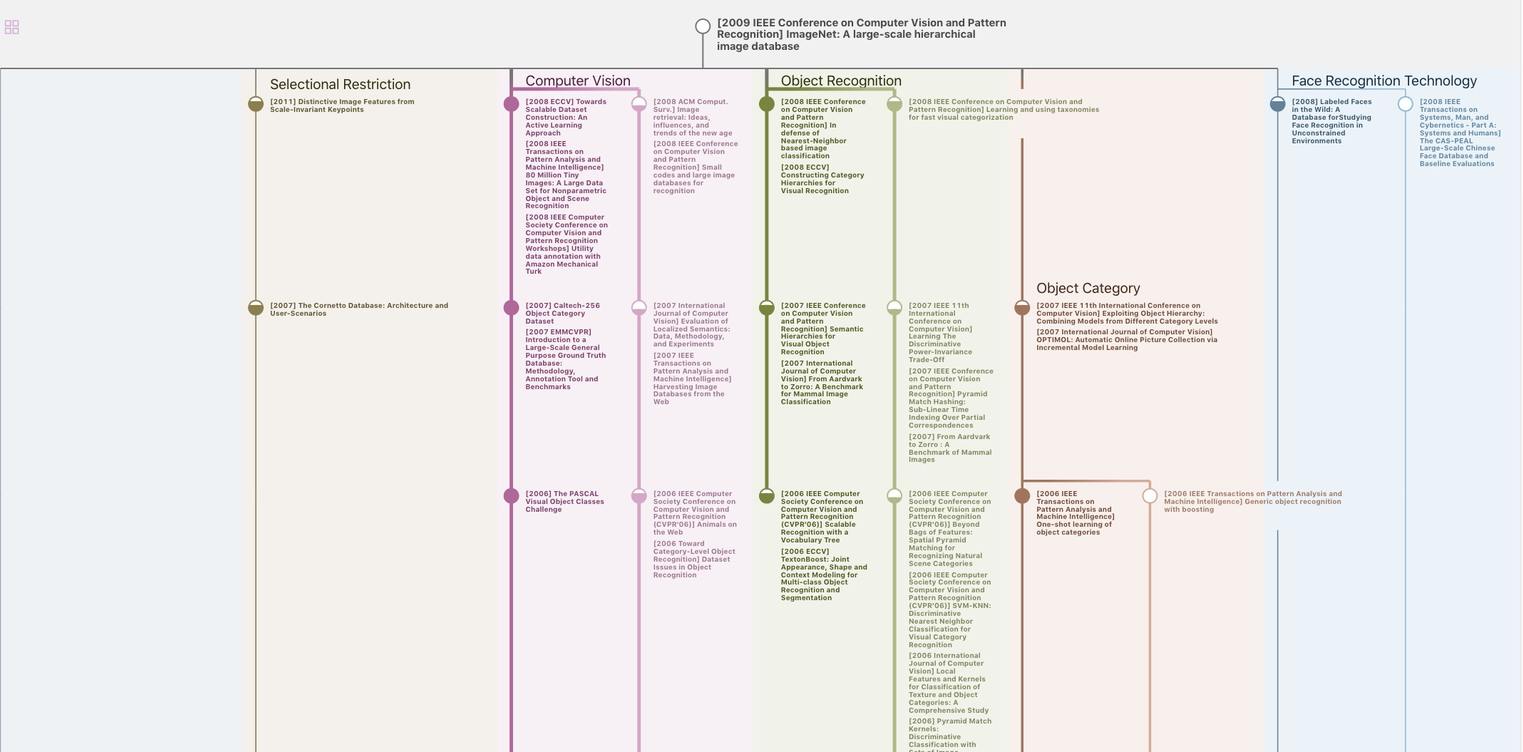
生成溯源树,研究论文发展脉络
数据免责声明
页面数据均来自互联网公开来源、合作出版商和通过AI技术自动分析结果,我们不对页面数据的有效性、准确性、正确性、可靠性、完整性和及时性做出任何承诺和保证。若有疑问,可以通过电子邮件方式联系我们:report@aminer.cn