On the Use of Physics in Machine Learning for Inspection and Control of Complex Processes
Computational Optical Imaging and Artificial Intelligence in Biomedical Sciences(2024)
摘要
Machine learning operators, such as neural networks, are universal function approximators—albeit, in practice, their generalization ability depends on the quality of the training data and the algorithm designer's wisdom in choosing a particular operator form, i.e. how well it matches the function at hand. Scientific machine learning is a class of methods that constrain the neural network operator by forcing its output to match time-series data from a partially known dynamical model, e.g. an ordinary or partial vector differential equation. In this talk, we make the case for regularizing optical image measurements using this approach. Applications are expected to be in processes with high-complexity constitutive relationships, such as pharmaceutical and cell manufacturing, plant biology, and ecology.
更多查看译文
AI 理解论文
溯源树
样例
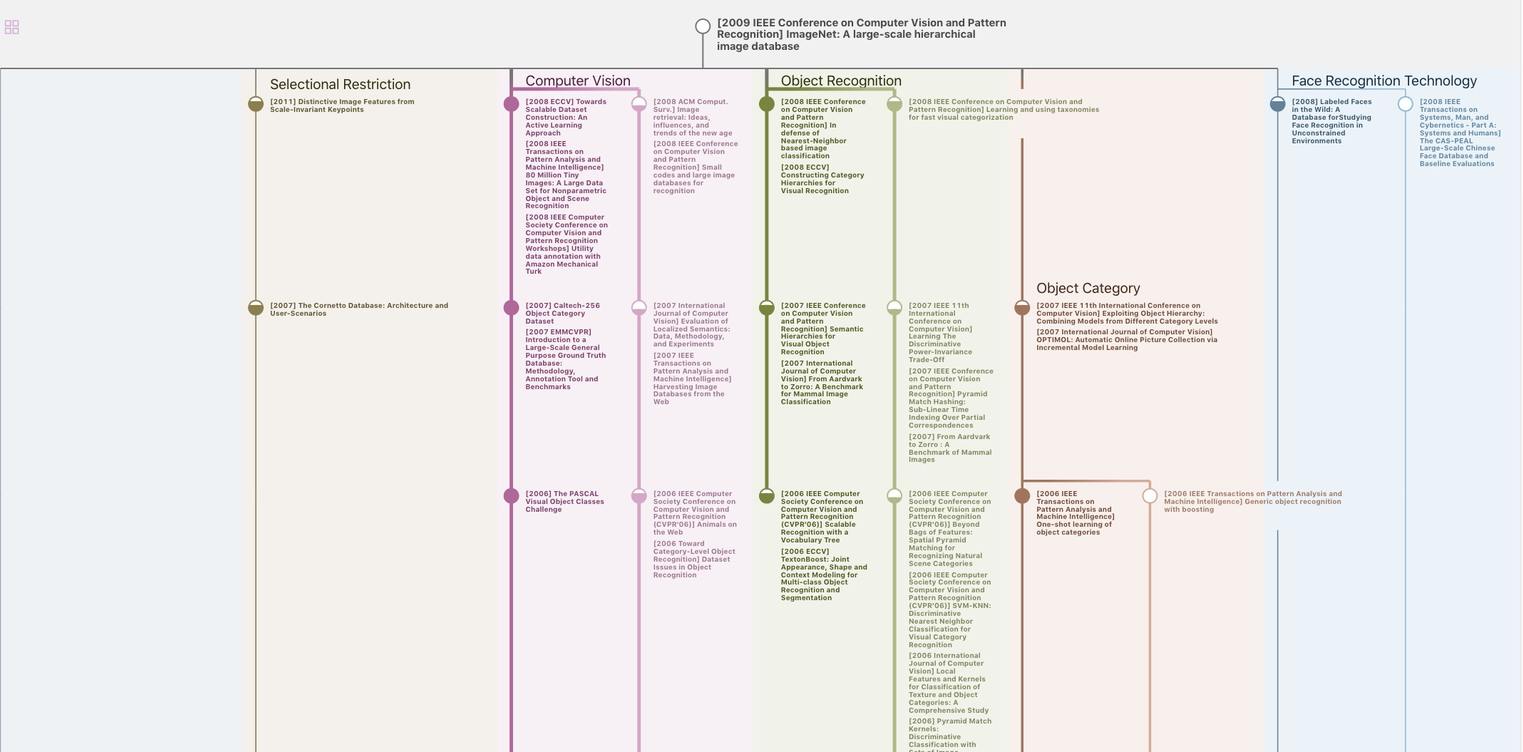
生成溯源树,研究论文发展脉络
数据免责声明
页面数据均来自互联网公开来源、合作出版商和通过AI技术自动分析结果,我们不对页面数据的有效性、准确性、正确性、可靠性、完整性和及时性做出任何承诺和保证。若有疑问,可以通过电子邮件方式联系我们:report@aminer.cn