PosSAM: Panoptic Open-vocabulary Segment Anything
CoRR(2024)
摘要
In this paper, we introduce an open-vocabulary panoptic segmentation model
that effectively unifies the strengths of the Segment Anything Model (SAM) with
the vision-language CLIP model in an end-to-end framework. While SAM excels in
generating spatially-aware masks, it's decoder falls short in recognizing
object class information and tends to oversegment without additional guidance.
Existing approaches address this limitation by using multi-stage techniques and
employing separate models to generate class-aware prompts, such as bounding
boxes or segmentation masks. Our proposed method, PosSAM is an end-to-end model
which leverages SAM's spatially rich features to produce instance-aware masks
and harnesses CLIP's semantically discriminative features for effective
instance classification. Specifically, we address the limitations of SAM and
propose a novel Local Discriminative Pooling (LDP) module leveraging
class-agnostic SAM and class-aware CLIP features for unbiased open-vocabulary
classification. Furthermore, we introduce a Mask-Aware Selective Ensembling
(MASE) algorithm that adaptively enhances the quality of generated masks and
boosts the performance of open-vocabulary classification during inference for
each image. We conducted extensive experiments to demonstrate our methods
strong generalization properties across multiple datasets, achieving
state-of-the-art performance with substantial improvements over SOTA
open-vocabulary panoptic segmentation methods. In both COCO to ADE20K and
ADE20K to COCO settings, PosSAM outperforms the previous state-of-the-art
methods by a large margin, 2.4 PQ and 4.6 PQ, respectively. Project Website:
https://vibashan.github.io/possam-web/.
更多查看译文
AI 理解论文
溯源树
样例
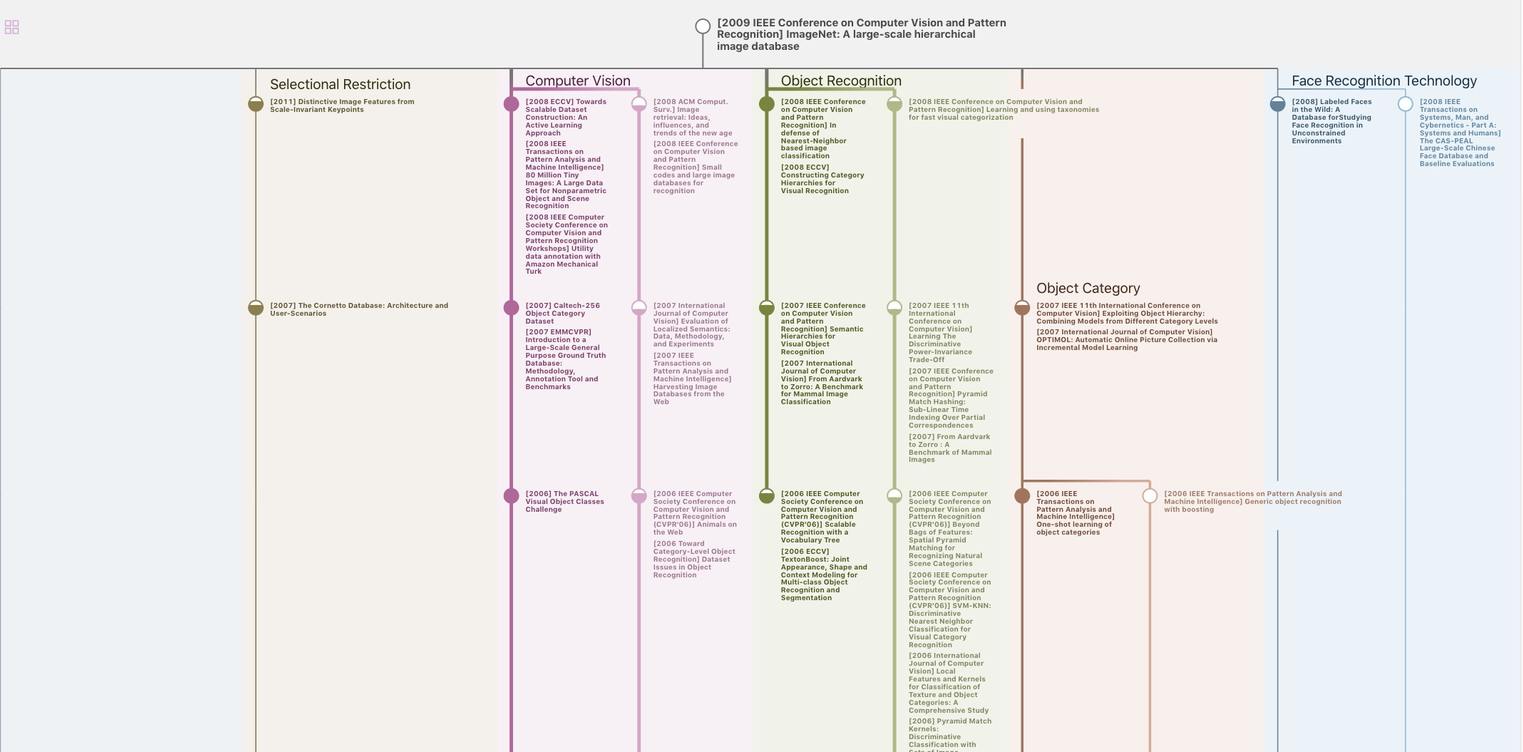
生成溯源树,研究论文发展脉络
数据免责声明
页面数据均来自互联网公开来源、合作出版商和通过AI技术自动分析结果,我们不对页面数据的有效性、准确性、正确性、可靠性、完整性和及时性做出任何承诺和保证。若有疑问,可以通过电子邮件方式联系我们:report@aminer.cn