Temporal Inconsistency-Based Active Learning.
IEEE International Conference on Acoustics, Speech, and Signal Processing(2024)
摘要
Deep supervised learning has demonstrated strong capabilities; however, such progress relies on massive and expensive data annotation. Active Learning (AL) has been introduced to selectively annotate samples, thus reducing the human labeling effort. Previous AL research has focused on employing recently trained models to design sampling strategies, based on uncertainty or representativeness. Drawing inspiration from the issue of model forgetting, we propose a novel AL framework called Temporal Inconsistency-Based Active Learning (TIR-AL). In this framework, multiple snapshots of the models across consecutive cycles are jointly utilized to select samples with higher temporal inconsistency, by computing the proposed self-weighted nuclear norm metric. Furthermore, we introduce a consistency regularization term to mitigate the issue of forgetting. Together, these components make full use of the potential of data and facilitate effective interaction within the AL loop. To demonstrate the efficacy of TIR-AL, we conducted a set of experiments illustrating how our approach outperforms state-of-the-art methods without incurring any additional training costs.
更多查看译文
关键词
Active learning,Temporal inconsistency,Consistency regularization,Self-weighted nuclear norm
AI 理解论文
溯源树
样例
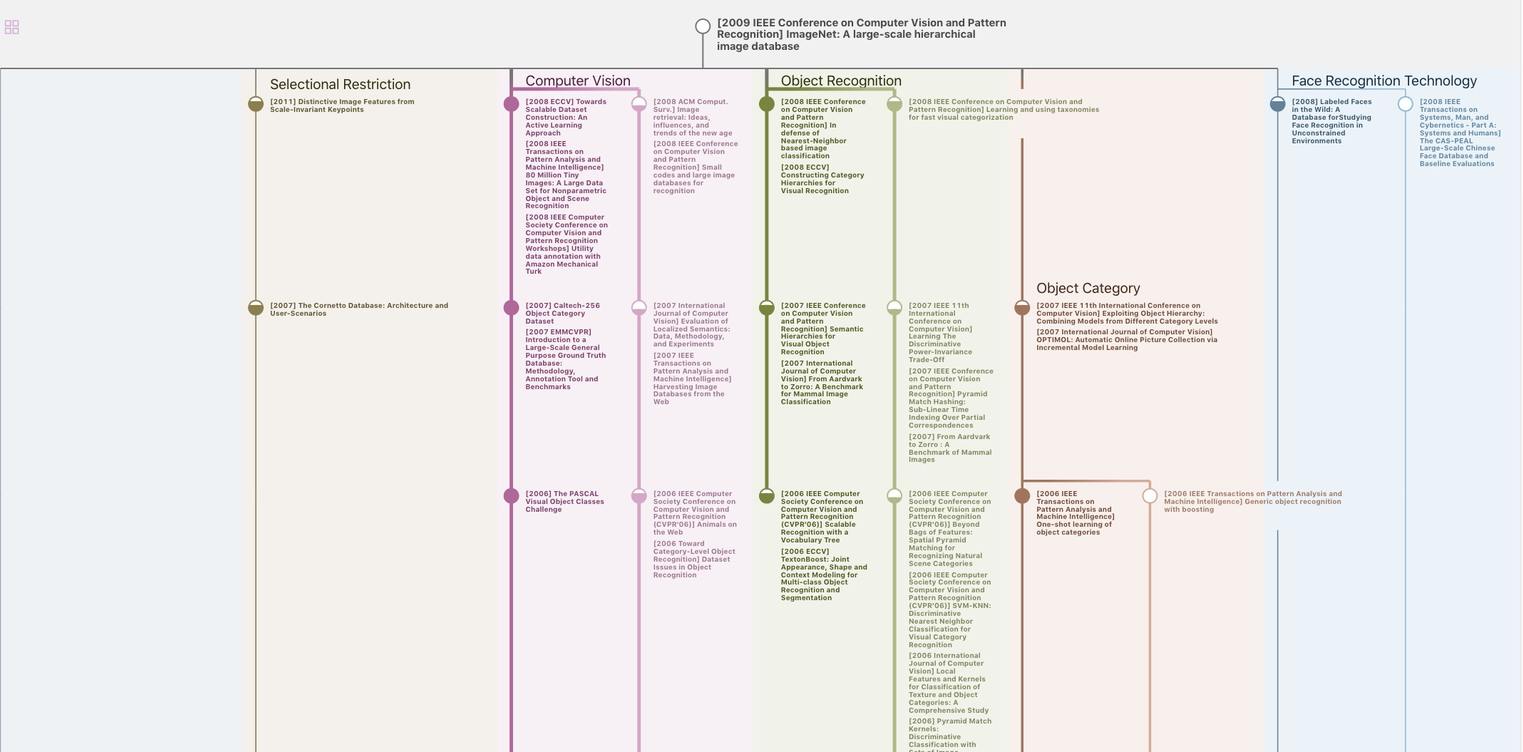
生成溯源树,研究论文发展脉络
数据免责声明
页面数据均来自互联网公开来源、合作出版商和通过AI技术自动分析结果,我们不对页面数据的有效性、准确性、正确性、可靠性、完整性和及时性做出任何承诺和保证。若有疑问,可以通过电子邮件方式联系我们:report@aminer.cn