FutureDepth: Learning to Predict the Future Improves Video Depth Estimation
European Conference on Computer Vision(2024)
摘要
In this paper, we propose a novel video depth estimation approach,FutureDepth, which enables the model to implicitly leverage multi-frame andmotion cues to improve depth estimation by making it learn to predict thefuture at training. More specifically, we propose a future prediction network,F-Net, which takes the features of multiple consecutive frames and is trainedto predict multi-frame features one time step ahead iteratively. In this way,F-Net learns the underlying motion and correspondence information, and weincorporate its features into the depth decoding process. Additionally, toenrich the learning of multiframe correspondence cues, we further leverage areconstruction network, R-Net, which is trained via adaptively maskedauto-encoding of multiframe feature volumes. At inference time, both F-Net andR-Net are used to produce queries to work with the depth decoder, as well as afinal refinement network. Through extensive experiments on several benchmarks,i.e., NYUDv2, KITTI, DDAD, and Sintel, which cover indoor, driving, andopen-domain scenarios, we show that FutureDepth significantly improves uponbaseline models, outperforms existing video depth estimation methods, and setsnew state-of-the-art (SOTA) accuracy. Furthermore, FutureDepth is moreefficient than existing SOTA video depth estimation models and has similarlatencies when comparing to monocular models
更多查看译文
关键词
Depth Estimation,Monocular Depth Estimation,Representation Learning,Deep Learning,Feature Matching
AI 理解论文
溯源树
样例
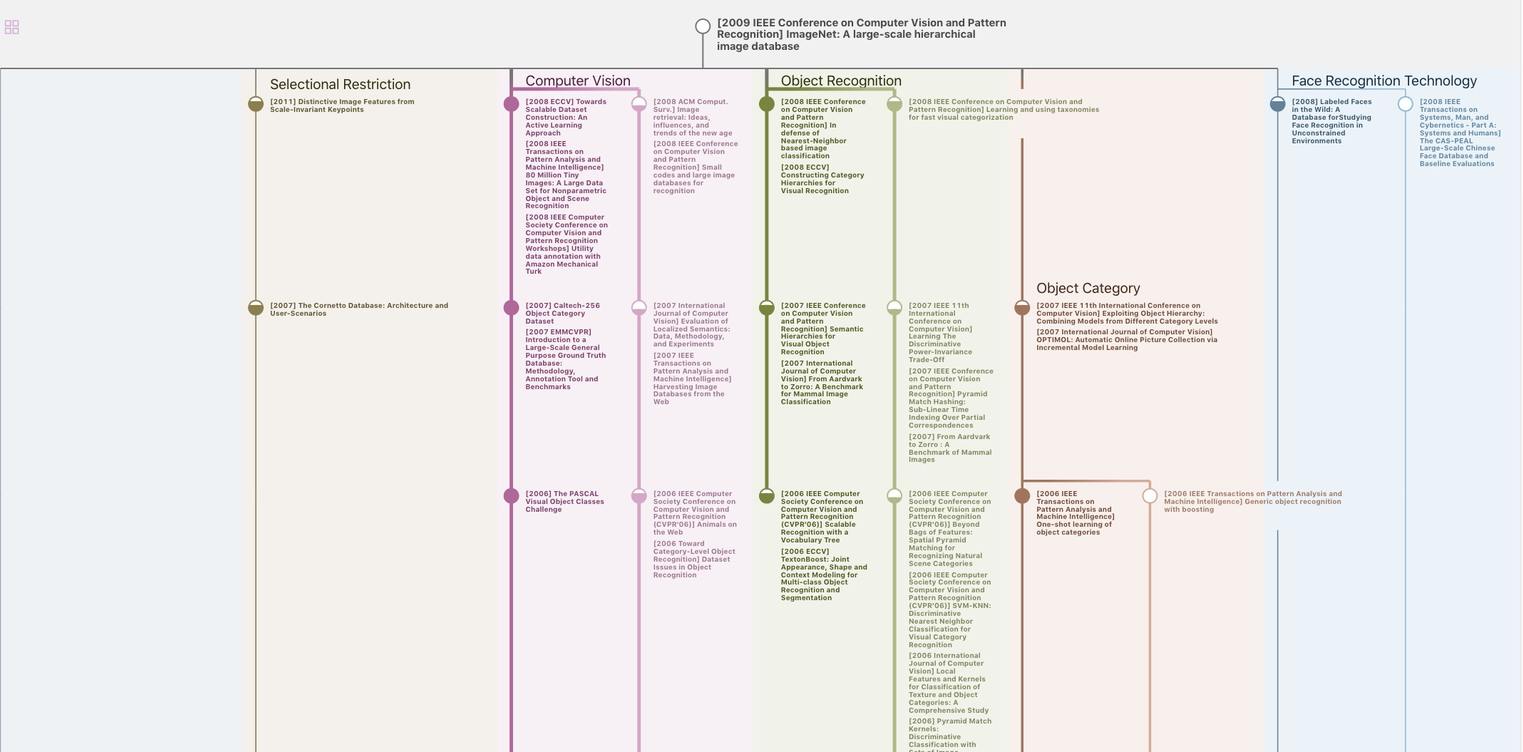
生成溯源树,研究论文发展脉络
数据免责声明
页面数据均来自互联网公开来源、合作出版商和通过AI技术自动分析结果,我们不对页面数据的有效性、准确性、正确性、可靠性、完整性和及时性做出任何承诺和保证。若有疑问,可以通过电子邮件方式联系我们:report@aminer.cn