Constrained Bayesian optimization with merit functions
arxiv(2024)
摘要
Bayesian optimization is a powerful optimization tool for problems where
native first-order derivatives are unavailable. Recently, constrained Bayesian
optimization (CBO) has been applied to many engineering applications where
constraints are essential. However, several obstacles remain with current CBO
algorithms that could prevent a wider adoption. We propose CBO algorithms using
merit functions, such as the penalty merit function, in acquisition functions,
inspired by nonlinear optimization methods, e.g., sequential quadratic
programming. Merit functions measure the potential progress of both the
objective and constraint functions, thus increasing algorithmic efficiency and
allowing infeasible initial samples. The acquisition functions with merit
functions are relaxed to have closed forms, making its implementation readily
available wherever Bayesian optimization is. We further propose a unified CBO
algorithm that can be seen as extension to the popular expected constrained
improvement (ECI) approach. We demonstrate the effectiveness and efficiency of
the proposed algorithms through numerical experiments on synthetic problems and
a practical data-driven engineering design problem in the field of plasma
physics.
更多查看译文
AI 理解论文
溯源树
样例
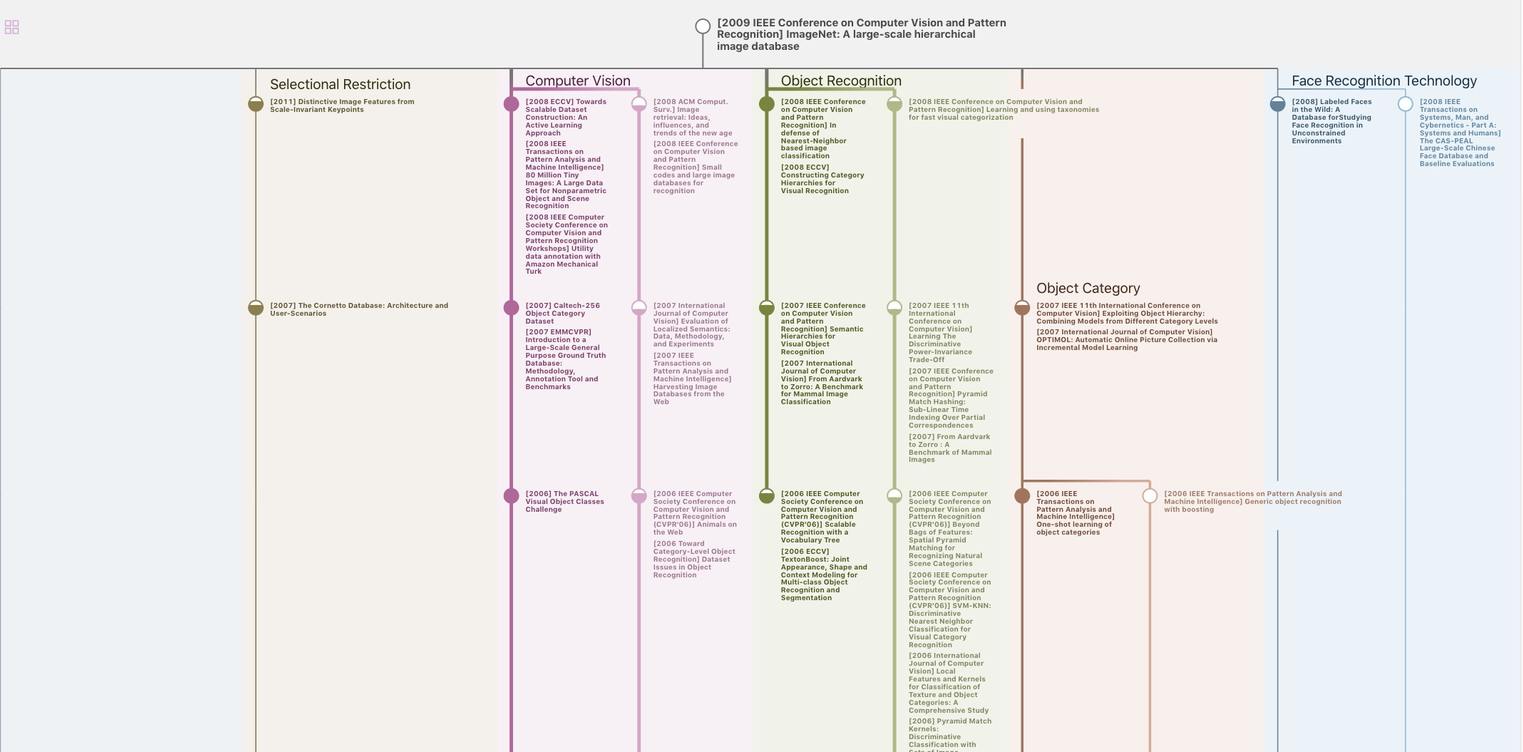
生成溯源树,研究论文发展脉络
数据免责声明
页面数据均来自互联网公开来源、合作出版商和通过AI技术自动分析结果,我们不对页面数据的有效性、准确性、正确性、可靠性、完整性和及时性做出任何承诺和保证。若有疑问,可以通过电子邮件方式联系我们:report@aminer.cn