SnAG: Scalable and Accurate Video Grounding
CVPR 2024(2024)
摘要
Temporal grounding of text descriptions in videos is a central problem invision-language learning and video understanding. Existing methods oftenprioritize accuracy over scalability – they have been optimized for groundingonly a few text queries within short videos, and fail to scale up to longvideos with hundreds of queries. In this paper, we study the effect ofcross-modal fusion on the scalability of video grounding models. Our analysisestablishes late fusion as a more cost-effective fusion scheme for long-formvideos with many text queries. Moreover, it leads us to a novel, video-centricsampling scheme for efficient training. Based on these findings, we presentSnAG, a simple baseline for scalable and accurate video grounding. Withoutbells and whistles, SnAG is 43state of the art for long-form video grounding on the challenging MAD dataset,while achieving highly competitive results on short videos.
更多查看译文
关键词
Video understanding,Temporal Sentence Grounding,Vision-Language Learning
AI 理解论文
溯源树
样例
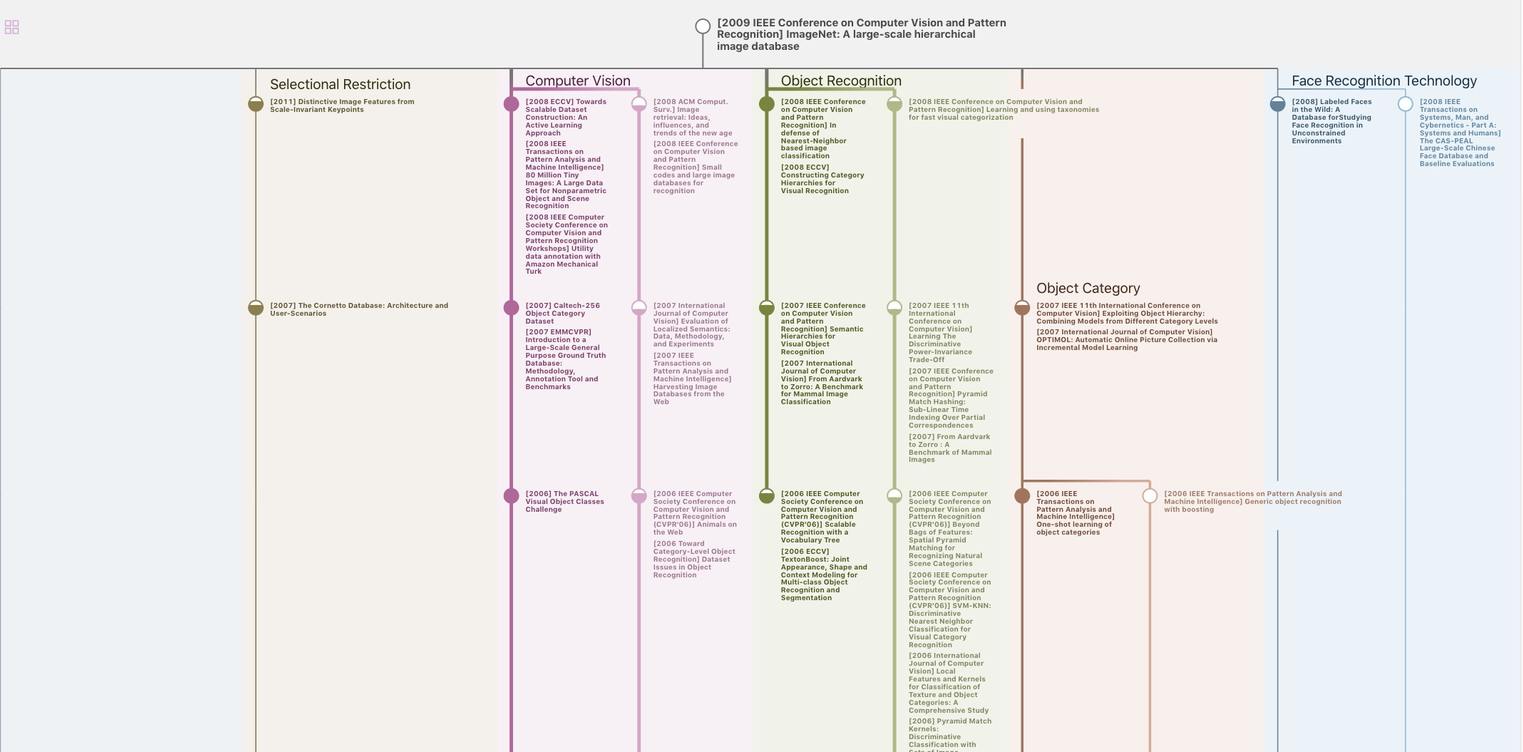
生成溯源树,研究论文发展脉络
数据免责声明
页面数据均来自互联网公开来源、合作出版商和通过AI技术自动分析结果,我们不对页面数据的有效性、准确性、正确性、可靠性、完整性和及时性做出任何承诺和保证。若有疑问,可以通过电子邮件方式联系我们:report@aminer.cn