RMem: Restricted Memory Banks Improve Video Object Segmentation
CVPR 2024(2024)
摘要
With recent video object segmentation (VOS) benchmarks evolving tochallenging scenarios, we revisit a simple but overlooked strategy: restrictingthe size of memory banks. This diverges from the prevalent practice ofexpanding memory banks to accommodate extensive historical information. Ourspecially designed "memory deciphering" study offers a pivotal insightunderpinning such a strategy: expanding memory banks, while seeminglybeneficial, actually increases the difficulty for VOS modules to decoderelevant features due to the confusion from redundant information. Byrestricting memory banks to a limited number of essential frames, we achieve anotable improvement in VOS accuracy. This process balances the importance andfreshness of frames to maintain an informative memory bank within a boundedcapacity. Additionally, restricted memory banks reduce the training-inferencediscrepancy in memory lengths compared with continuous expansion. This fostersnew opportunities in temporal reasoning and enables us to introduce thepreviously overlooked "temporal positional embedding." Finally, our insightsare embodied in "RMem" ("R" for restricted), a simple yet effective VOSmodification that excels at challenging VOS scenarios and establishes new stateof the art for object state changes (on the VOST dataset) and long videos (onthe Long Videos dataset). Our code and demo are available athttps://restricted-memory.github.io/.
更多查看译文
关键词
video object segmentation,embodied ai,egocentric vision,video understanding
AI 理解论文
溯源树
样例
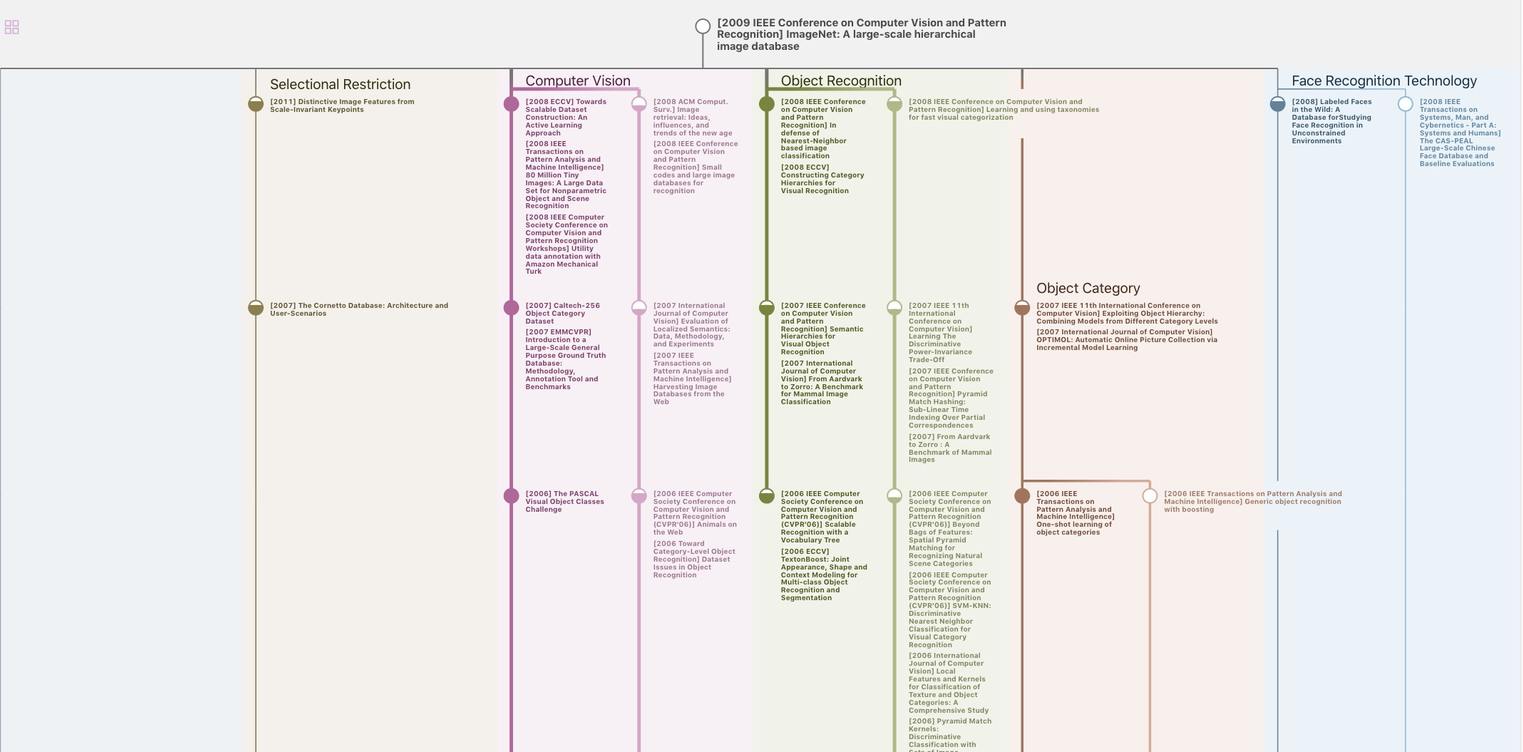
生成溯源树,研究论文发展脉络
数据免责声明
页面数据均来自互联网公开来源、合作出版商和通过AI技术自动分析结果,我们不对页面数据的有效性、准确性、正确性、可靠性、完整性和及时性做出任何承诺和保证。若有疑问,可以通过电子邮件方式联系我们:report@aminer.cn