Text-Enhanced Data-free Approach for Federated Class-Incremental Learning
CVPR 2024(2024)
摘要
Federated Class-Incremental Learning (FCIL) is an underexplored yet pivotalissue, involving the dynamic addition of new classes in the context offederated learning. In this field, Data-Free Knowledge Transfer (DFKT) plays acrucial role in addressing catastrophic forgetting and data privacy problems.However, prior approaches lack the crucial synergy between DFKT and the modeltraining phases, causing DFKT to encounter difficulties in generatinghigh-quality data from a non-anchored latent space of the old task model. Inthis paper, we introduce LANDER (Label Text Centered Data-Free KnowledgeTransfer) to address this issue by utilizing label text embeddings (LTE)produced by pretrained language models. Specifically, during the model trainingphase, our approach treats LTE as anchor points and constrains the featureembeddings of corresponding training samples around them, enriching thesurrounding area with more meaningful information. In the DFKT phase, by usingthese LTE anchors, LANDER can synthesize more meaningful samples, therebyeffectively addressing the forgetting problem. Additionally, instead of tightlyconstraining embeddings toward the anchor, the Bounding Loss is introduced toencourage sample embeddings to remain flexible within a defined radius. Thisapproach preserves the natural differences in sample embeddings and mitigatesthe embedding overlap caused by heterogeneous federated settings. Extensiveexperiments conducted on CIFAR100, Tiny-ImageNet, and ImageNet demonstrate thatLANDER significantly outperforms previous methods and achieves state-of-the-artperformance in FCIL. The code is available athttps://github.com/tmtuan1307/lander.
更多查看译文
关键词
federated learning,continual learning,data-free knowledge distillation,knowledge transfer,text embedding
AI 理解论文
溯源树
样例
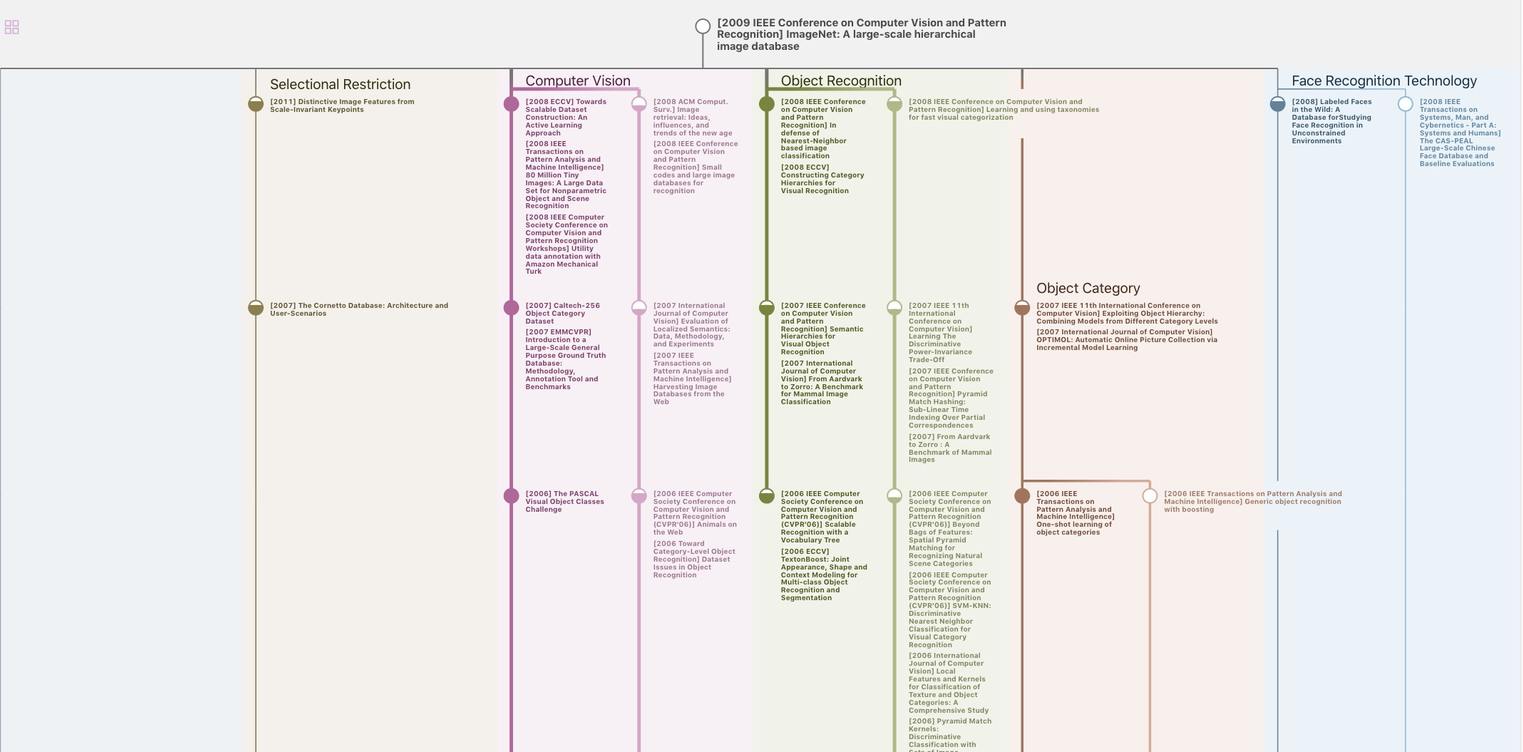
生成溯源树,研究论文发展脉络
数据免责声明
页面数据均来自互联网公开来源、合作出版商和通过AI技术自动分析结果,我们不对页面数据的有效性、准确性、正确性、可靠性、完整性和及时性做出任何承诺和保证。若有疑问,可以通过电子邮件方式联系我们:report@aminer.cn