SoundingActions: Learning How Actions Sound from Narrated Egocentric Videos
CVPR 2024(2024)
摘要
We propose a novel self-supervised embedding to learn how actions sound fromnarrated in-the-wild egocentric videos. Whereas existing methods rely oncurated data with known audio-visual correspondence, our multimodalcontrastive-consensus coding (MC3) embedding reinforces the associationsbetween audio, language, and vision when all modality pairs agree, whilediminishing those associations when any one pair does not. We show our approachcan successfully discover how the long tail of human actions sound fromegocentric video, outperforming an array of recent multimodal embeddingtechniques on two datasets (Ego4D and EPIC-Sounds) and multiple cross-modaltasks.
更多查看译文
关键词
Narrative,Egocentric Videos,Ablation,Training Data,Mutual Information,Representation Learning,Latent Space,Action Recognition,Pairwise Similarity,Random Chance,Visual Activity,Self-supervised Learning,Stage Of Loss,Contrastive Loss,Training Paradigm,Video Dataset,Embedding Learning,Consensus Score,Contrast Objective,Two-stage Training,Video Encoding
AI 理解论文
溯源树
样例
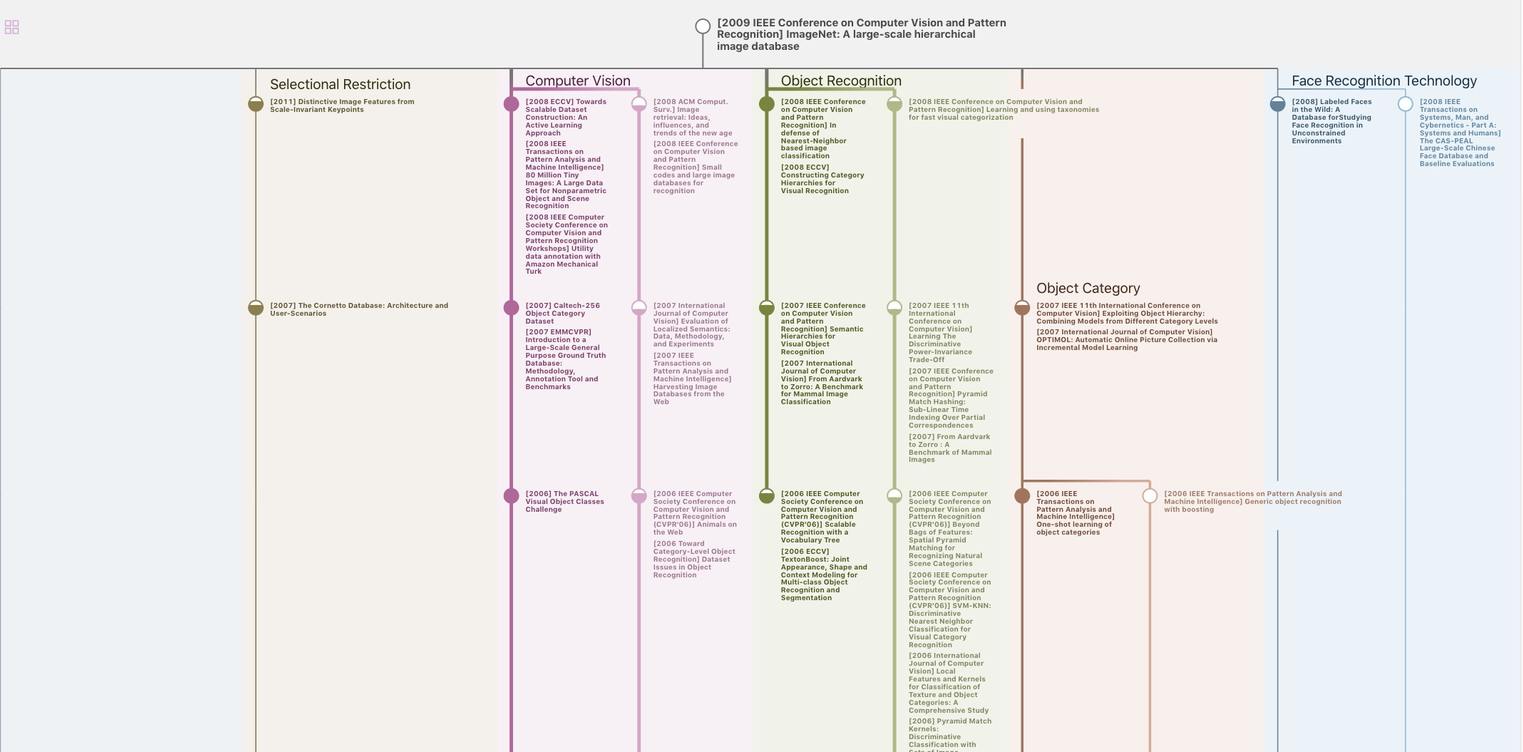
生成溯源树,研究论文发展脉络
数据免责声明
页面数据均来自互联网公开来源、合作出版商和通过AI技术自动分析结果,我们不对页面数据的有效性、准确性、正确性、可靠性、完整性和及时性做出任何承诺和保证。若有疑问,可以通过电子邮件方式联系我们:report@aminer.cn