Adversarial Text to Continuous Image Generation
CVPR 2024(2024)
摘要
Existing GAN-based text-to-image models treat images as 2D pixel arrays. In this paper, we approach the text-to-image task from a different perspective, where a 2D image is represented as an implicit neural representation (INR). We show that straightforward conditioning of the unconditional INR-based GAN method on text inputs is not enough to achieve good performance. We propose a word-level attention-based weight modulation operator that controls the generation process of INR-GAN based on hypernetworks. Our experiments on benchmark datasets show that HyperCGAN achieves competitive performance to existing pixel-based methods and retains the properties of continuous generative models. Project page link: https://kilichbek.github.io/webpagelhypercgan.
更多查看译文
关键词
Image Generation,Continuous Imaging,Continuous Generation,Input Text,Operation Module,Spatial Resolution,Image Features,Super-resolution,Attention Mechanism,Diffusion Model,Textual Information,Fewer Parameters,Textual Descriptions,Word Embedding,Words In Sentences,Textual Features,Image Synthesis,Word Level,Image X,Conditional Generative Adversarial Network,Fréchet Inception Distance,COCO Dataset,Sentence Embedding,Grid Coordinates
AI 理解论文
溯源树
样例
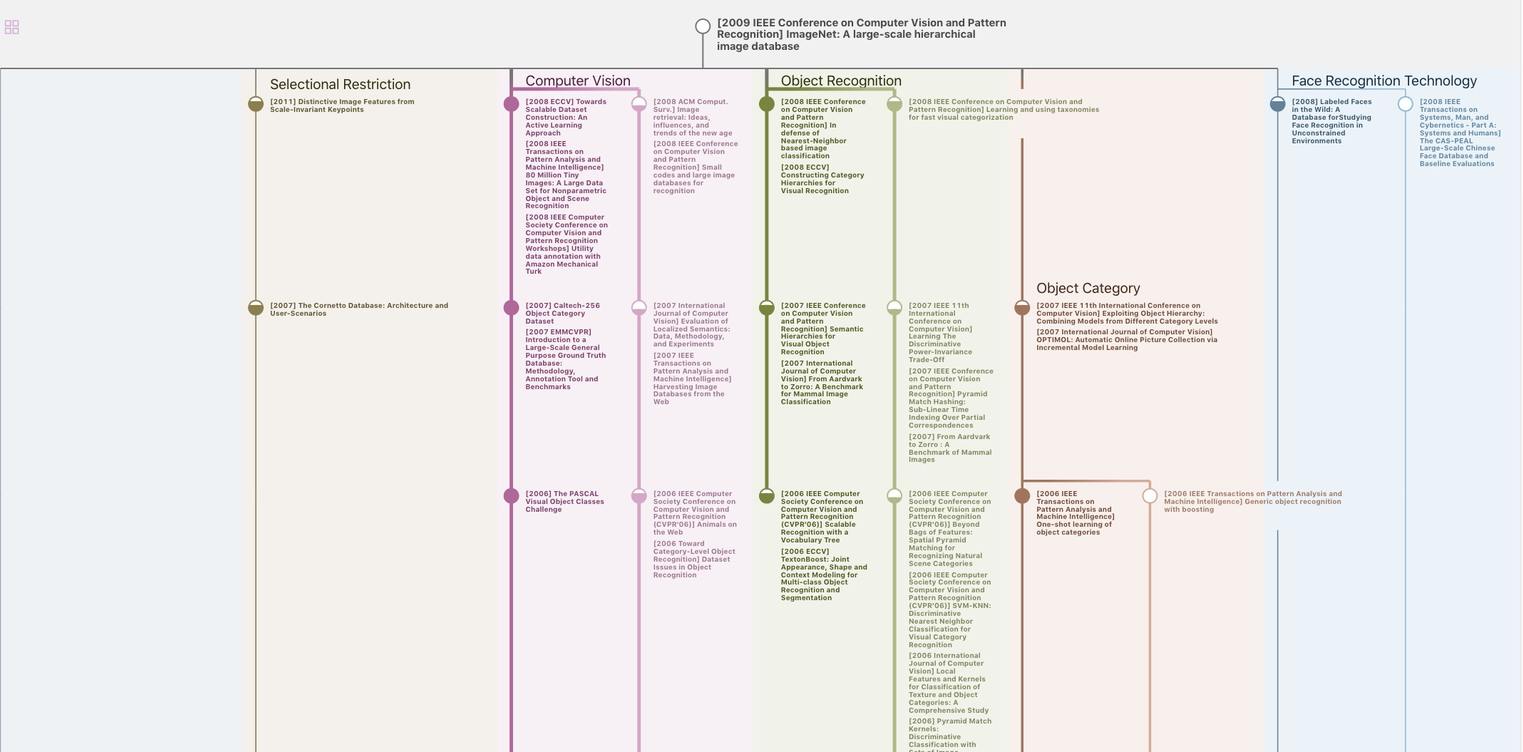
生成溯源树,研究论文发展脉络
数据免责声明
页面数据均来自互联网公开来源、合作出版商和通过AI技术自动分析结果,我们不对页面数据的有效性、准确性、正确性、可靠性、完整性和及时性做出任何承诺和保证。若有疑问,可以通过电子邮件方式联系我们:report@aminer.cn