AI and Memory Wall
IEEE MICRO(2024)
摘要
The availability of unprecedented unsupervised training data, along withneural scaling laws, has resulted in an unprecedented surge in model size andcompute requirements for serving/training LLMs. However, the main performancebottleneck is increasingly shifting to memory bandwidth. Over the past 20years, peak server hardware FLOPS has been scaling at 3.0x/2yrs, outpacing thegrowth of DRAM and interconnect bandwidth, which have only scaled at 1.6 and1.4 times every 2 years, respectively. This disparity has made memory, ratherthan compute, the primary bottleneck in AI applications, particularly inserving. Here, we analyze encoder and decoder Transformer models and show howmemory bandwidth can become the dominant bottleneck for decoder models. Weargue for a redesign in model architecture, training, and deployment strategiesto overcome this memory limitation.
更多查看译文
关键词
Computational modeling,Training,Transformers,Bandwidth,Arithmetic,Hardware,Data models
AI 理解论文
溯源树
样例
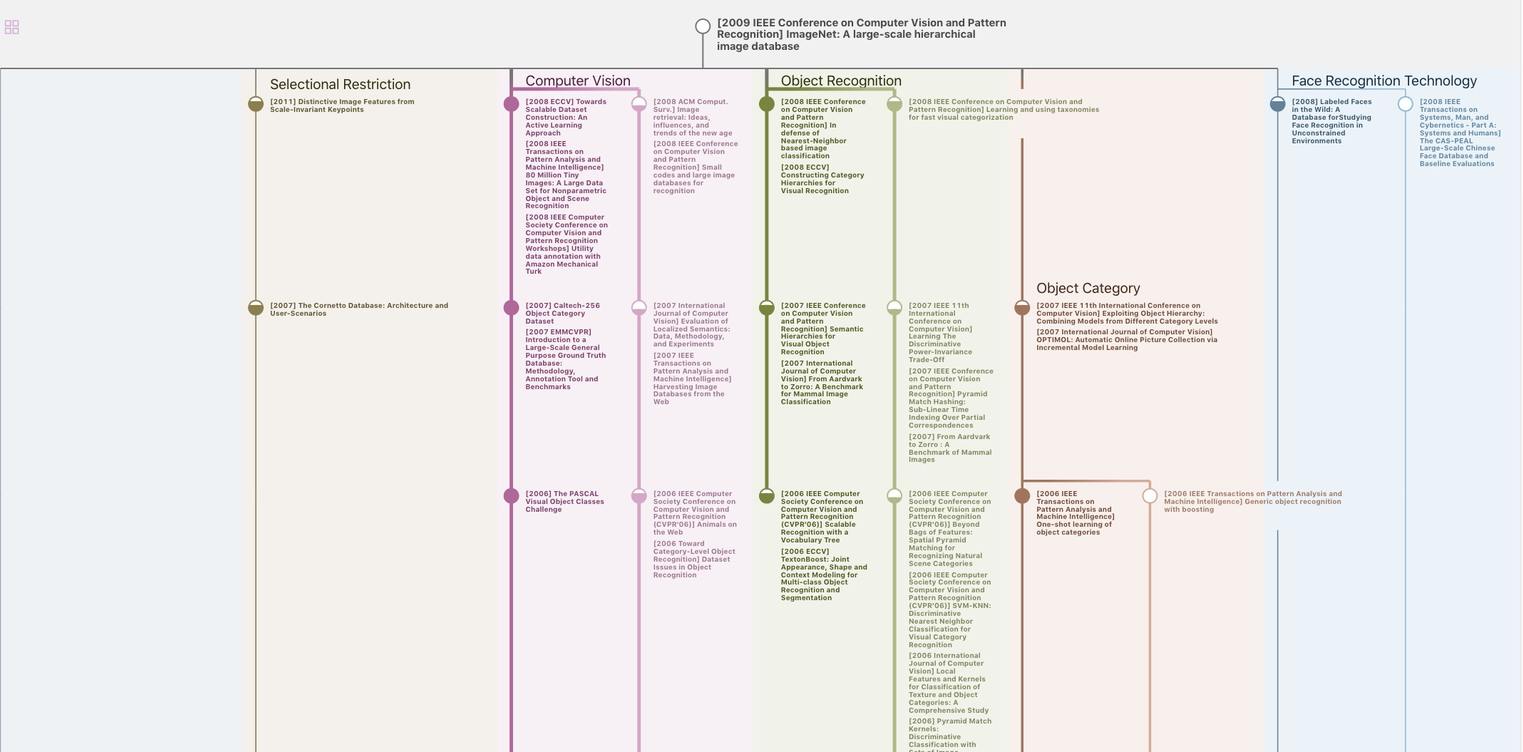
生成溯源树,研究论文发展脉络
数据免责声明
页面数据均来自互联网公开来源、合作出版商和通过AI技术自动分析结果,我们不对页面数据的有效性、准确性、正确性、可靠性、完整性和及时性做出任何承诺和保证。若有疑问,可以通过电子邮件方式联系我们:report@aminer.cn