Predicting Microbial Growth Conditions from Amino Acid Composition
crossref(2024)
摘要
The ability to grow a microbe in the laboratory enables reproducible study and engineering of its genetics. Unfortunately, the majority of microbes in the tree of life remain uncultivated because of the effort required to identify culturing conditions. Predictions of viable growth conditions to guide experimental testing would be highly desirable. While carbon and energy sources can be computationally predicted with annotated genes, it is harder to predict other requirements for growth such as oxygen, temperature, salinity, and pH. Here, we developed genome-based computational models capable of predicting oxygen tolerance (92% balanced accuracy), optimum temperature (R2=0.73), salinity (R2=0.81) and pH (R2=0.48) for novel taxonomic microbial families without requiring functional gene annotations. Using growth conditions and genome sequences of 15,596 bacteria and archaea, we found that amino acid frequencies are predictive of growth requirements. As little as two amino acids can predict oxygen tolerance with 88% balanced accuracy. Using cellular localization of proteins to compute amino acid frequencies improved prediction of pH (R2 increase of 0.36). Because these models do not rely on the presence or absence of specific genes, they can be applied to incomplete genomes, requiring as little as 10% completeness. We applied our models to predict growth requirements for all 85,205 species of sequenced bacteria and archaea and found that uncultivated species are enriched in thermophiles, anaerobes, and acidophiles. Finally, we applied our models to 3,349 environmental samples with metagenome-assembled genomes and showed that individual microbes within a community have differing growth requirements. This work guides identification of growth constraints for laboratory cultivation of diverse microbes.
更多查看译文
关键词
Microbial Metabolism
AI 理解论文
溯源树
样例
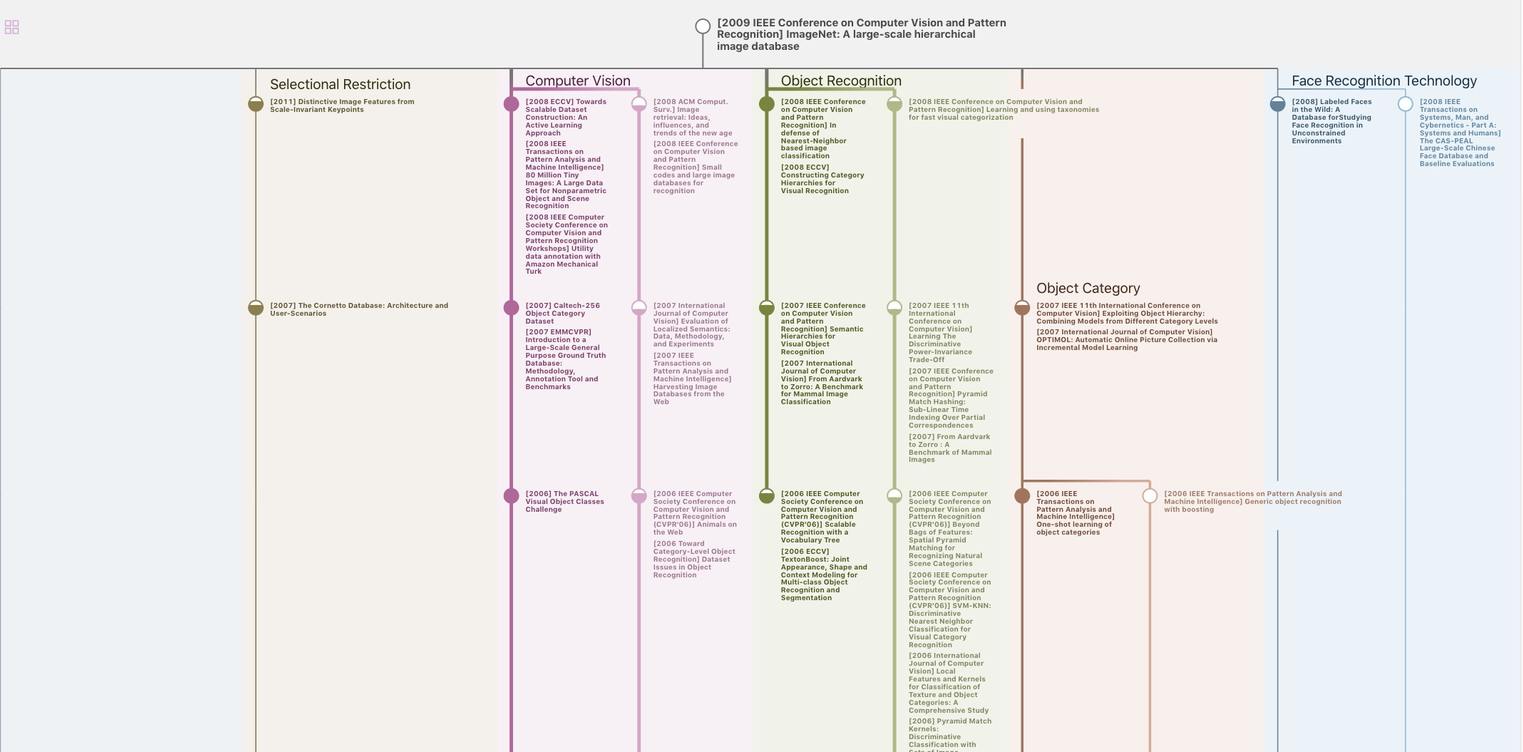
生成溯源树,研究论文发展脉络
数据免责声明
页面数据均来自互联网公开来源、合作出版商和通过AI技术自动分析结果,我们不对页面数据的有效性、准确性、正确性、可靠性、完整性和及时性做出任何承诺和保证。若有疑问,可以通过电子邮件方式联系我们:report@aminer.cn