Pin-opt: Graph Representation Learning for Large-scale Pin Assignment Optimization of Microbumps Considering Signal and Power Integrity
IEEE TRANSACTIONS ON COMPONENTS PACKAGING AND MANUFACTURING TECHNOLOGY(2024)
摘要
In this work, we propose a deep reinforcement learning (DRL) framework called Pin-opt, designed to create a reusable solver capable of optimizing pin assignment to minimize signal integrity (SI) and power integrity (PI) degradation in microbump packages. The increasing data rates of high-bandwidth systems have made SI/PI issues critical for ensuring the reliability of these systems. While previous research using meta-heuristic methods has optimized pin assignment to reduce SI/PI degradation in similar vertical interconnections, these approaches tend to be inflexible, providing problem-specific solutions suitable only for square-shaped pin arrangements. Our approach, Pin-opt, leverages the advantages of a learning-based method to create a practical solution applicable to pin maps of any shape and with a very large pin count. By representing pins as graphs during the training process, Pin-opt becomes adaptable to any pin arrangement and demonstrates significant performance improvements when solving large-scale pin assignment problems. We evaluate the performance, computational cost, reusability, and scalability of Pin-opt by comparing it to the genetic algorithm, a conventional meta-heuristic method used for solving optimization tasks. To demonstrate its practicality, Pin-opt is also applied to a pin map of high bandwidth memory (HBM).
更多查看译文
关键词
Pins,Packaging,Manufacturing,Degradation,Training,Task analysis,Metaheuristics,Deep reinforcement learning (DRL),graph representation learning,machine learning,pin assignment,power integrity (PI),signal integrity (SI)
AI 理解论文
溯源树
样例
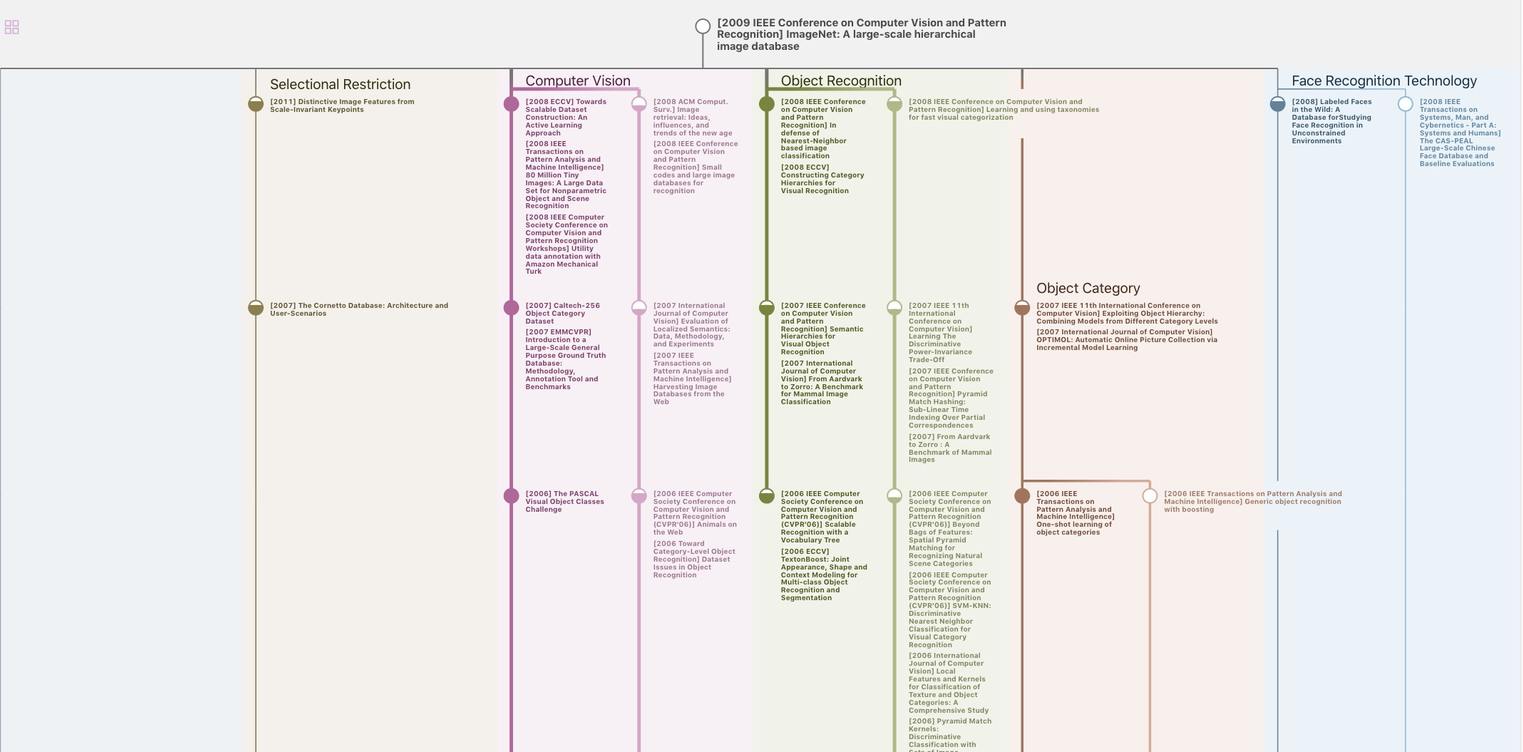
生成溯源树,研究论文发展脉络
数据免责声明
页面数据均来自互联网公开来源、合作出版商和通过AI技术自动分析结果,我们不对页面数据的有效性、准确性、正确性、可靠性、完整性和及时性做出任何承诺和保证。若有疑问,可以通过电子邮件方式联系我们:report@aminer.cn