Prompt-prompted Adaptive Structured Pruning for Efficient LLM Generation
CoRR(2024)
摘要
With the development of transformer-based large language models (LLMs), they
have been applied to many fields due to their remarkable utility, but this
comes at a considerable computational cost at deployment. Fortunately, some
methods such as pruning or constructing a mixture of experts (MoE) aim at
exploiting sparsity in transformer feedforward (FF) blocks to gain boosts in
speed and reduction in memory requirements. However, these techniques can be
very costly and inflexible in practice, as they often require training or are
restricted to specific types of architectures. To address this, we introduce
GRIFFIN, a novel training-free and calibration-free method that selects unique
FF experts at the sequence level for efficient generation across a plethora of
LLMs with different non-ReLU activation functions. This is possible due to a
critical observation that many trained LLMs naturally produce highly structured
FF activation patterns within a sequence, which we call flocking. Despite our
method's simplicity, we show with 50
the original model's performance with little to no degradation on a variety of
classification and generation tasks, all while improving latency (e.g.
1.29× and 1.25× speed-ups in Gemma 7B and Llama 2 13B,
respectively, on an NVIDIA L40). Code is available at
https://github.com/hdong920/GRIFFIN.
更多查看译文
AI 理解论文
溯源树
样例
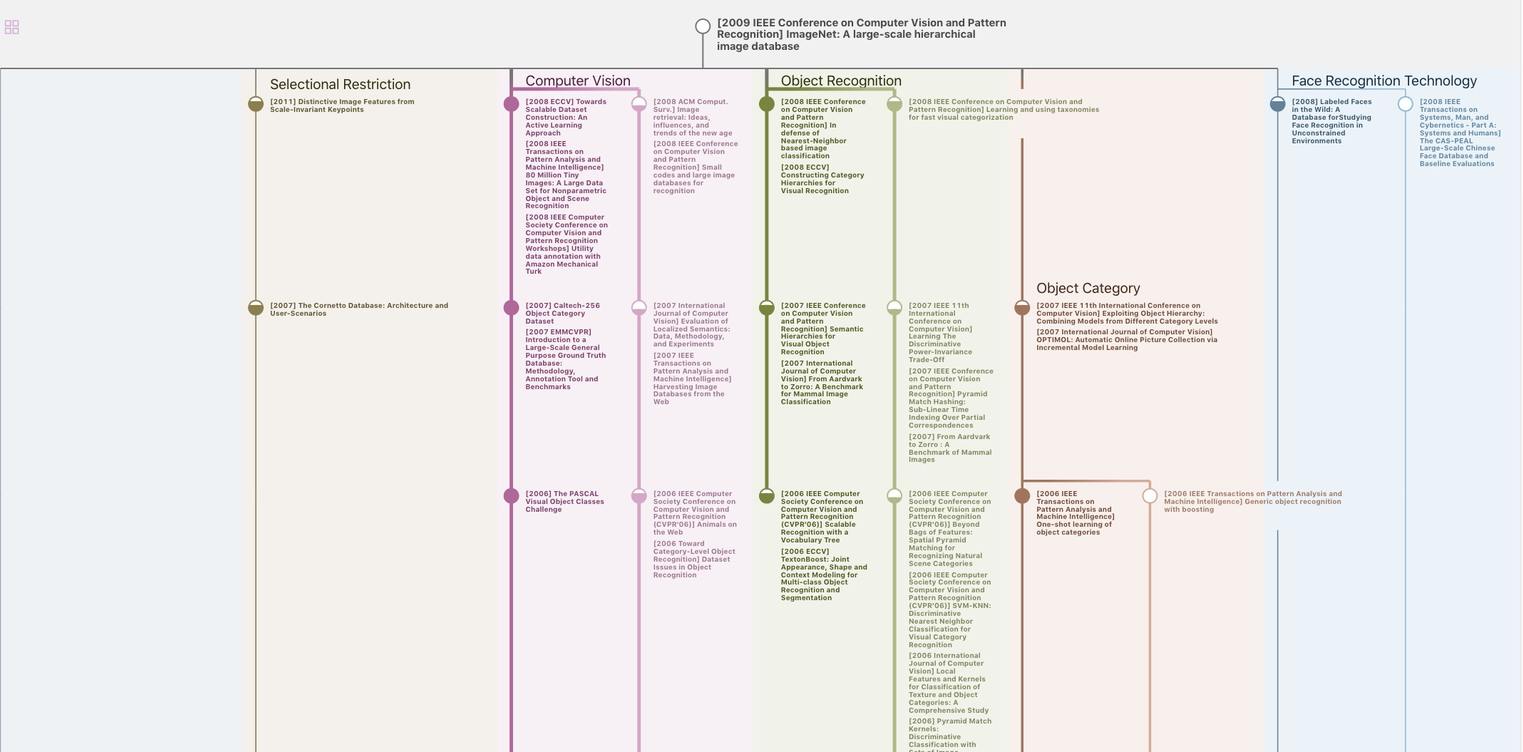
生成溯源树,研究论文发展脉络
数据免责声明
页面数据均来自互联网公开来源、合作出版商和通过AI技术自动分析结果,我们不对页面数据的有效性、准确性、正确性、可靠性、完整性和及时性做出任何承诺和保证。若有疑问,可以通过电子邮件方式联系我们:report@aminer.cn