MoMA: Multimodal LLM Adapter for Fast Personalized Image Generation
European Conference on Computer Vision(2024)
摘要
In this paper, we present MoMA: an open-vocabulary, training-freepersonalized image model that boasts flexible zero-shot capabilities. Asfoundational text-to-image models rapidly evolve, the demand for robustimage-to-image translation grows. Addressing this need, MoMA specializes insubject-driven personalized image generation. Utilizing an open-source,Multimodal Large Language Model (MLLM), we train MoMA to serve a dual role asboth a feature extractor and a generator. This approach effectively synergizesreference image and text prompt information to produce valuable image features,facilitating an image diffusion model. To better leverage the generatedfeatures, we further introduce a novel self-attention shortcut method thatefficiently transfers image features to an image diffusion model, improving theresemblance of the target object in generated images. Remarkably, as atuning-free plug-and-play module, our model requires only a single referenceimage and outperforms existing methods in generating images with high detailfidelity, enhanced identity-preservation and prompt faithfulness. Our work isopen-source, thereby providing universal access to these advancements.
更多查看译文
关键词
Image Annotation,Image Retrieval,Feature Matching,Local Descriptors,Scalable Compression
AI 理解论文
溯源树
样例
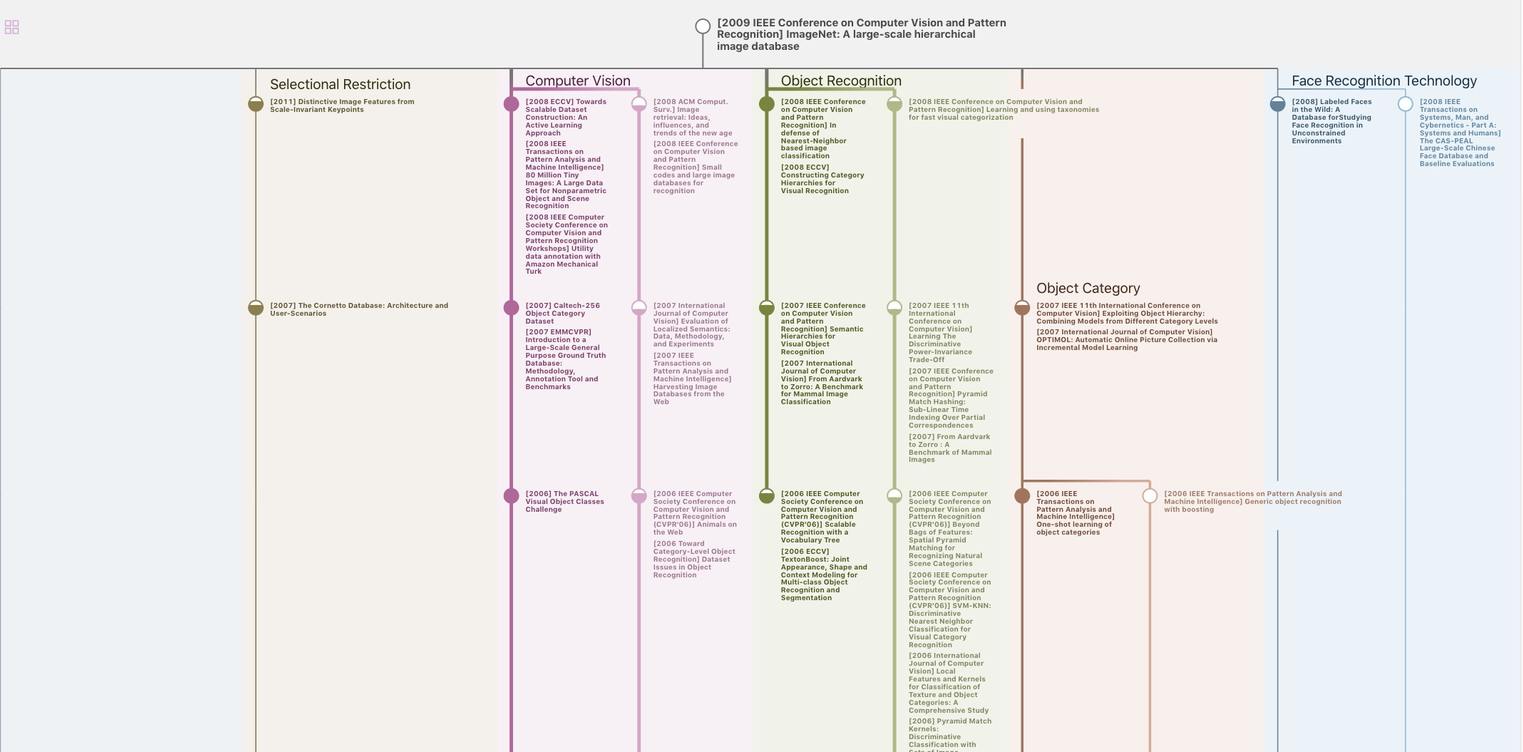
生成溯源树,研究论文发展脉络
数据免责声明
页面数据均来自互联网公开来源、合作出版商和通过AI技术自动分析结果,我们不对页面数据的有效性、准确性、正确性、可靠性、完整性和及时性做出任何承诺和保证。若有疑问,可以通过电子邮件方式联系我们:report@aminer.cn