LoRA Dropout as a Sparsity Regularizer for Overfitting Control
CoRR(2024)
摘要
Parameter-efficient fine-tuning methods, represented by LoRA, play an
essential role in adapting large-scale pre-trained models to downstream tasks.
However, fine-tuning LoRA-series models also faces the risk of overfitting on
the training dataset, and yet there's still a lack of theoretical guidance and
practical mechanism to control overfitting on LoRA-based PEFT methods. In this
paper, we propose a LoRA Dropout mechanism for the LoRA-based methods by
introducing random noises to the learnable low-rank matrices and increasing
parameter sparsity. We then demonstrate the theoretical mechanism of our LoRA
Dropout mechanism from the perspective of sparsity regularization by providing
a generalization error bound under this framework. Theoretical results show
that appropriate sparsity would help tighten the gap between empirical and
generalization risks and thereby control overfitting. Furthermore, based on the
LoRA Dropout framework, we introduce a test-time ensemble strategy and provide
theoretical evidence demonstrating that the ensemble method can further
compress the error bound, and lead to better performance during inference time.
Extensive experiments on various NLP tasks provide practical validations of the
effectiveness of our LoRA Dropout framework in improving model accuracy and
calibration.
更多查看译文
AI 理解论文
溯源树
样例
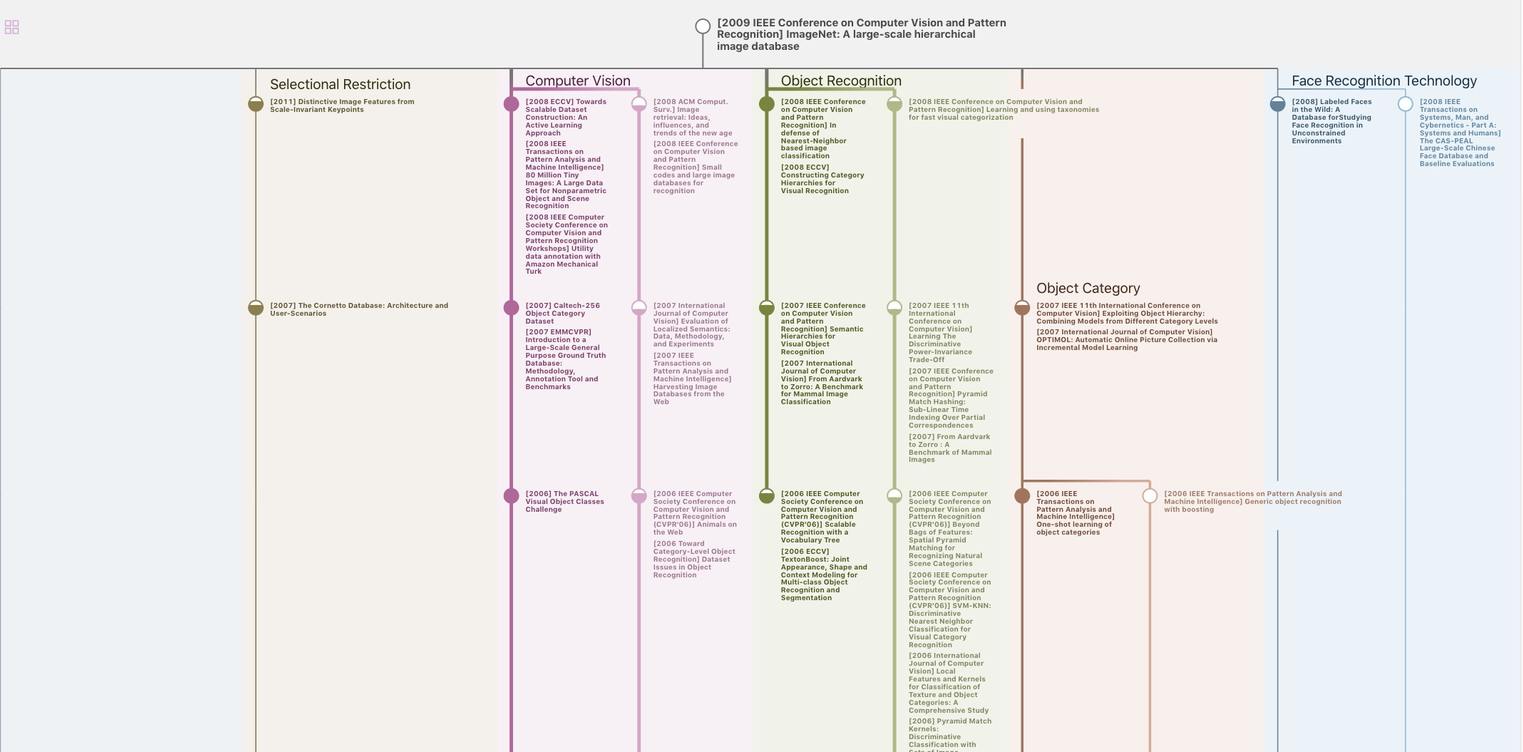
生成溯源树,研究论文发展脉络
数据免责声明
页面数据均来自互联网公开来源、合作出版商和通过AI技术自动分析结果,我们不对页面数据的有效性、准确性、正确性、可靠性、完整性和及时性做出任何承诺和保证。若有疑问,可以通过电子邮件方式联系我们:report@aminer.cn