Following the Human Thread in Social Navigation
arXiv (Cornell University)(2024)
摘要
The success of collaboration between humans and robots in shared environmentsrelies on the robot's real-time adaptation to human motion. Specifically, inSocial Navigation, the agent should be close enough to assist but ready to backup to let the human move freely, avoiding collisions. Human trajectories emergeas crucial cues in Social Navigation, but they are partially observable fromthe robot's egocentric view and computationally complex to process. We propose the first Social Dynamics Adaptation model (SDA) based on therobot's state-action history to infer the social dynamics. We propose atwo-stage Reinforcement Learning framework: the first learns to encode thehuman trajectories into social dynamics and learns a motion policy conditionedon this encoded information, the current status, and the previous action. Here,the trajectories are fully visible, i.e., assumed as privileged information. Inthe second stage, the trained policy operates without direct access totrajectories. Instead, the model infers the social dynamics solely from thehistory of previous actions and statuses in real-time. Tested on the novelHabitat 3.0 platform, SDA sets a novel state of the art (SoA) performance infinding and following humans.
更多查看译文
关键词
Storytelling,Narratives,Narrative Theory
AI 理解论文
溯源树
样例
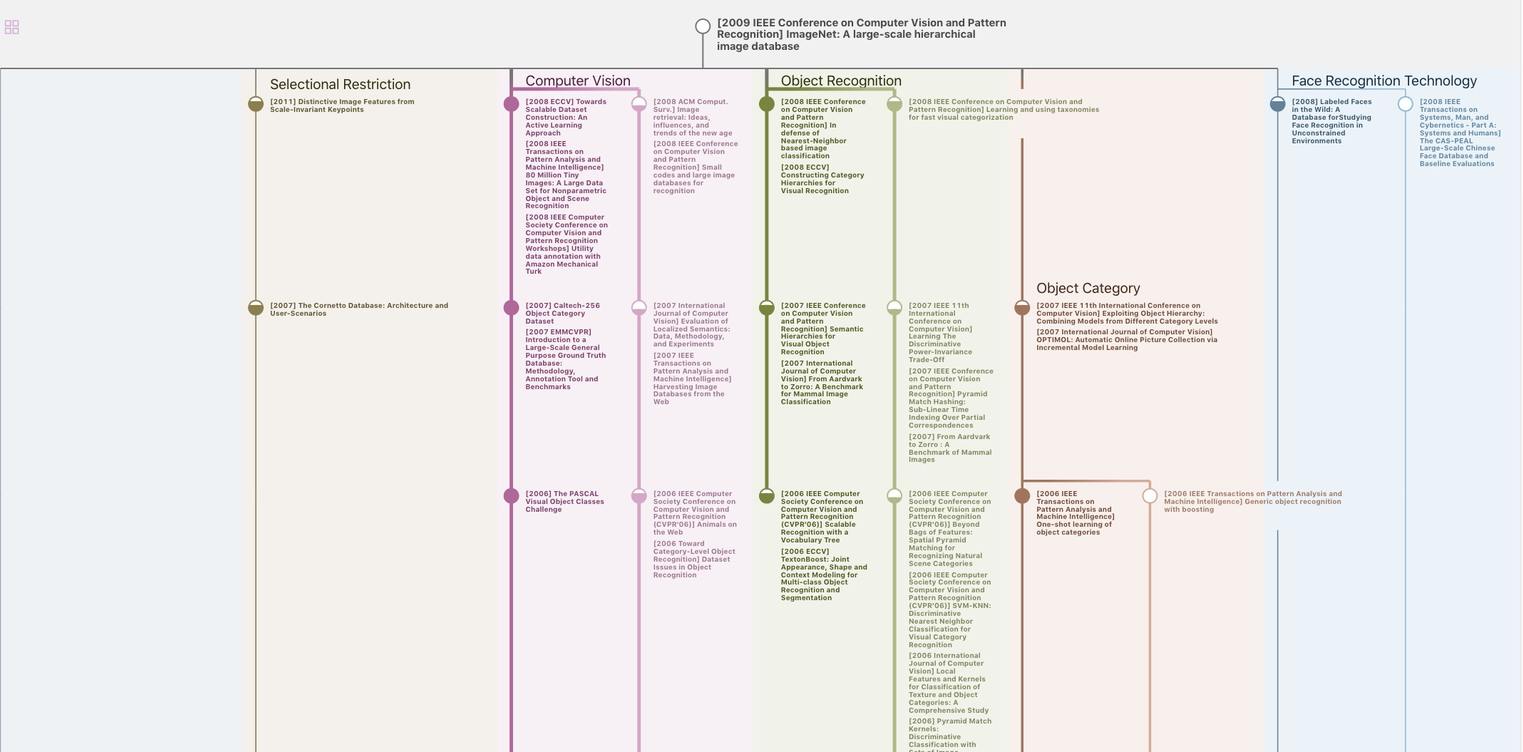
生成溯源树,研究论文发展脉络
数据免责声明
页面数据均来自互联网公开来源、合作出版商和通过AI技术自动分析结果,我们不对页面数据的有效性、准确性、正确性、可靠性、完整性和及时性做出任何承诺和保证。若有疑问,可以通过电子邮件方式联系我们:report@aminer.cn