Predicting Influenza-Like Illness Trends Based on Sentinel Surveillance Data in China from 2011 to 2019: A Modelling and Comparative Study
INFECTIOUS DISEASE MODELLING(2024)
摘要
Background: Influenza is an acute respiratory infectious disease with a significant global disease burden. Additionally, the coronavirus disease 2019 pandemic and its related nonpharmaceutical interventions (NPIs) have introduced uncertainty to the spread of influenza. However, comparative studies on the performance of innovative models and approaches used for influenza prediction are limited. Therefore, this study aimed to predict the trend of influenza-like illness (ILI) in settings with diverse climate characteristics in China based on sentinel surveillance data using three approaches and evaluate and compare their predictive performance. Methods: The generalized additive model (GAM), deep learning hybrid model based on Gate Recurrent Unit (GRU), and autoregressive moving average-generalized autoregressive conditional heteroscedasticity (ARMAdGARCH) model were established to predict the trends of ILI 1-, 2-, 3-, and 4-week-ahead in Beijing, Tianjin, Shanxi, Hubei, Chongqing, Guangdong, Hainan, and the Hong Kong Special Administrative Region in China, based on sentinel surveillance data from 2011 to 2019. Three relevant metrics, namely, Mean Absolute Percentage Error (MAPE), Root Mean Squared Error (RMSE), and R squared, were calculated to evaluate and compare the goodness of fit and robustness of the three models. Results: Considering the MAPE, RMSE, and R squared values, the ARMAdGARCH model performed best, while the GRU-based deep learning hybrid model exhibited moderate performance , GAM made predictions with the least accuracy in the eight settings in China. Additionally, the models ' predictive performance declined as the weeks ahead increased. Furthermore, blocked cross -validation indicated that all models were robust to changes in data and had low risks of overfitting. Conclusions: Our study suggested that the ARMA-GARCH model exhibited the best ac- curacy in predicting ILI trends in China compared to the GAM and GRU-based deep learning hybrid model. Therefore, in the future, the ARMA-GARCH model may be used to predict ILI trends in public health practice across diverse climatic zones, thereby contributing to influenza control and prevention efforts. (c) 2024 The Authors. Publishing services by Elsevier B.V. on behalf of KeAi Communications Co. Ltd. This is an open access article under the CC BY -NC -ND license (http://creativecommons.org/licenses/by-nc-nd/4.0/).
更多查看译文
关键词
Influenza-like illness,Influenza,Sentinel surveillance,China,Predicting,Modeling
AI 理解论文
溯源树
样例
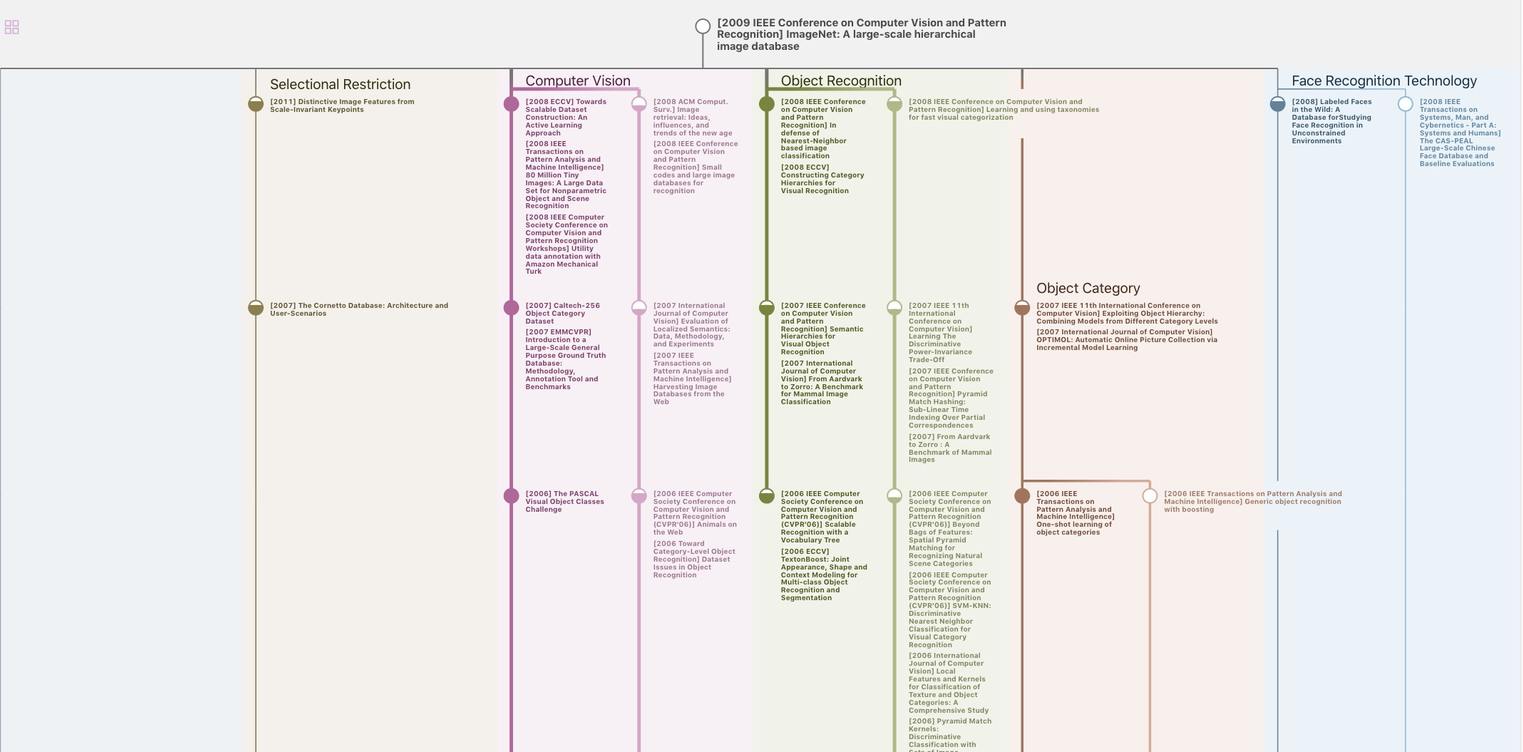
生成溯源树,研究论文发展脉络
数据免责声明
页面数据均来自互联网公开来源、合作出版商和通过AI技术自动分析结果,我们不对页面数据的有效性、准确性、正确性、可靠性、完整性和及时性做出任何承诺和保证。若有疑问,可以通过电子邮件方式联系我们:report@aminer.cn