One-Shot Image Restoration
CoRR(2024)
摘要
Image restoration, or inverse problems in image processing, has long been an
extensively studied topic. In recent years supervised learning approaches have
become a popular strategy attempting to tackle this task. Unfortunately, most
supervised learning-based methods are highly demanding in terms of
computational resources and training data (sample complexity). In addition,
trained models are sensitive to domain changes, such as varying acquisition
systems, signal sampling rates, resolution and contrast. In this work, we try
to answer a fundamental question: Can supervised learning models generalize
well solely by learning from one image or even part of an image? If so, then
what is the minimal amount of patches required to achieve acceptable
generalization? To this end, we focus on an efficient patch-based learning
framework that requires a single image input-output pair for training.
Experimental results demonstrate the applicability, robustness and
computational efficiency of the proposed approach for supervised image
deblurring and super-resolution. Our results showcase significant improvement
of learning models' sample efficiency, generalization and time complexity, that
can hopefully be leveraged for future real-time applications, and applied to
other signals and modalities.
更多查看译文
AI 理解论文
溯源树
样例
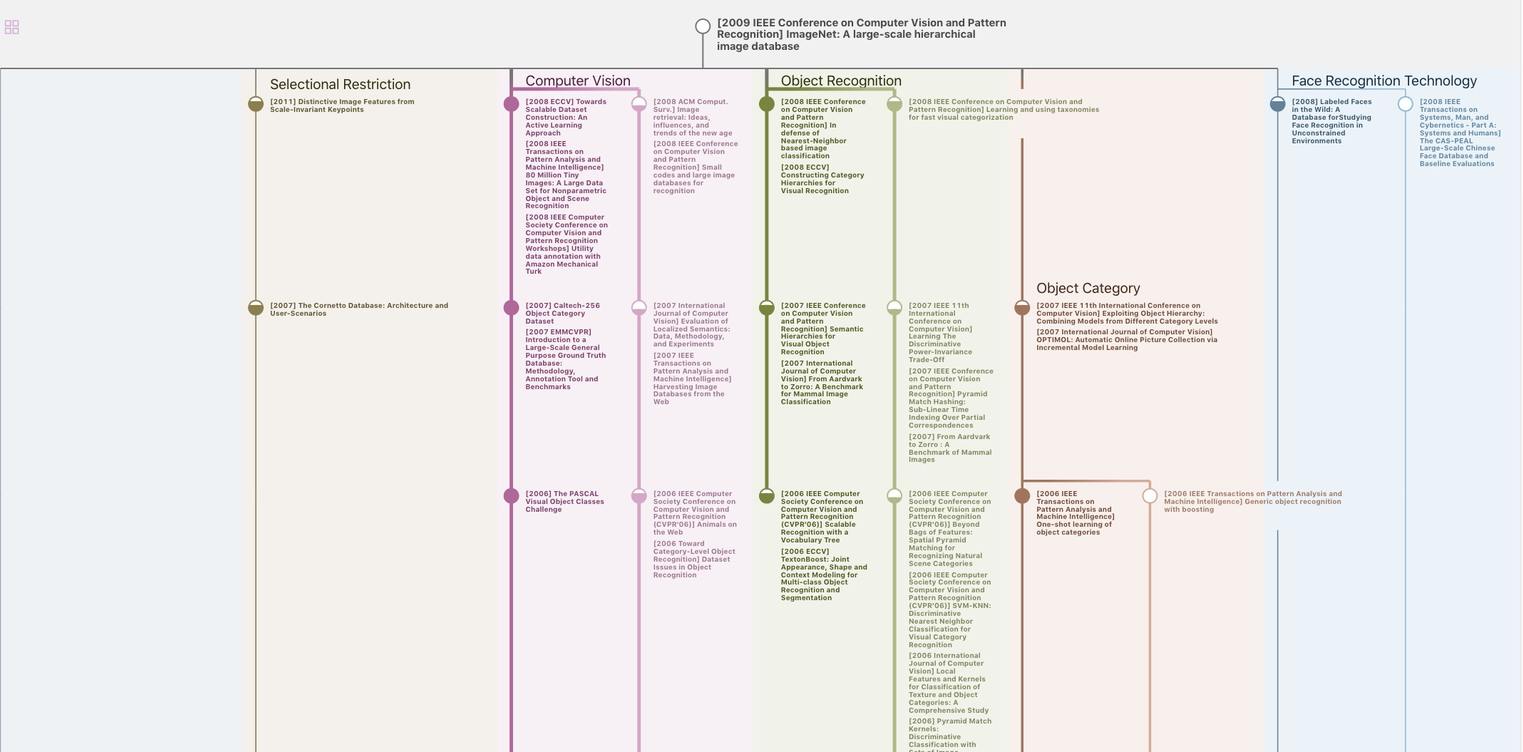
生成溯源树,研究论文发展脉络
数据免责声明
页面数据均来自互联网公开来源、合作出版商和通过AI技术自动分析结果,我们不对页面数据的有效性、准确性、正确性、可靠性、完整性和及时性做出任何承诺和保证。若有疑问,可以通过电子邮件方式联系我们:report@aminer.cn