A New Unconditionally Stable FDTD Method Based on Artificial Neural Network
IEEE TRANSACTIONS ON MICROWAVE THEORY AND TECHNIQUES(2024)
摘要
This article presents a new unconditionally stable finite-difference time-domain (FDTD) method combined with the artificial neural network (ANN) for multiscale problems. The input of ANN-FDTD is the point location of spatial grid division in FDTD, and the output is the field data at these points. At each time step, the hypothetical solution of Maxwell’s equations is constructed using a known forced excitation source and the output of ANN. The error of ANN is determined by the gradient of its output with respect to the input vector. This error value is used to update ANN parameters with the backpropagation (BP) algorithm, so the training does not have to involve labeled samples. Here, ANN is trained to ensure that the hypothetical solution satisfies the boundary conditions. ANN is trained individually at each time-marching step, and thus, the results of the previous step do not affect those of the next step. The time step of ANN-FDTD can be chosen much larger than that of the traditional FDTD for microwave components with fine structures. Moreover, all time steps can be divided into blocks to execute parallel computation. Three numerical examples are provided to validate the accuracy and efficiency of the proposed method.
更多查看译文
关键词
Time-domain analysis,Finite difference methods,Artificial neural networks,Mathematical models,Boundary conditions,Optimization,Training,Artificial neural network (ANN),finite-difference time-domain (FDTD),parallel computation,partial differential equation (PDE),unconditional stability
AI 理解论文
溯源树
样例
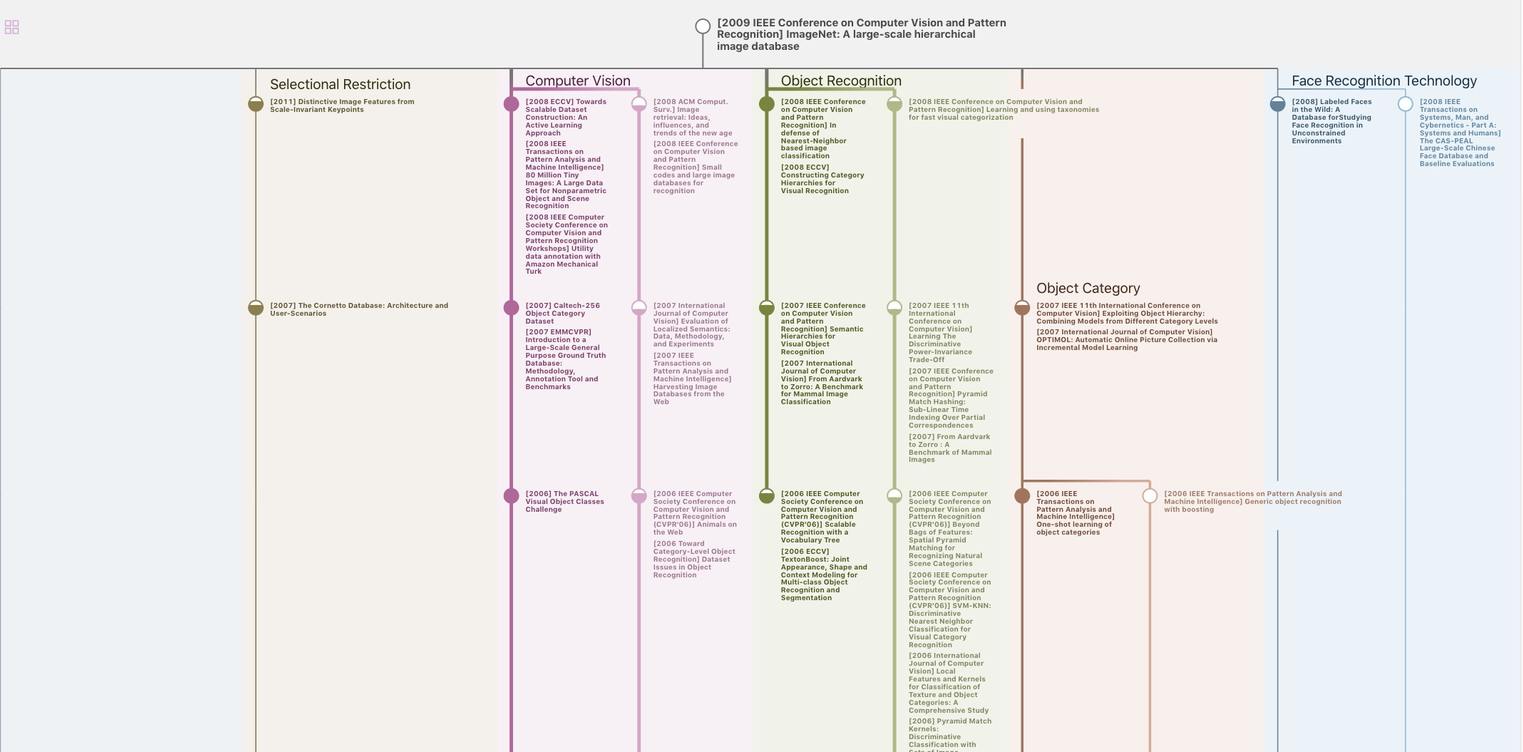
生成溯源树,研究论文发展脉络
数据免责声明
页面数据均来自互联网公开来源、合作出版商和通过AI技术自动分析结果,我们不对页面数据的有效性、准确性、正确性、可靠性、完整性和及时性做出任何承诺和保证。若有疑问,可以通过电子邮件方式联系我们:report@aminer.cn