OmniDrive: A Holistic LLM-Agent Framework for Autonomous Driving with 3D Perception, Reasoning and Planning
CoRR(2024)
摘要
The advances in multimodal large language models (MLLMs) have led to growing
interests in LLM-based autonomous driving agents to leverage their strong
reasoning capabilities. However, capitalizing on MLLMs' strong reasoning
capabilities for improved planning behavior is challenging since planning
requires full 3D situational awareness beyond 2D reasoning. To address this
challenge, our work proposes a holistic framework for strong alignment between
agent models and 3D driving tasks. Our framework starts with a novel 3D MLLM
architecture that uses sparse queries to lift and compress visual
representations into 3D before feeding them into an LLM. This query-based
representation allows us to jointly encode dynamic objects and static map
elements (e.g., traffic lanes), providing a condensed world model for
perception-action alignment in 3D. We further propose OmniDrive-nuScenes, a new
visual question-answering dataset challenging the true 3D situational awareness
of a model with comprehensive visual question-answering (VQA) tasks, including
scene description, traffic regulation, 3D grounding, counterfactual reasoning,
decision making and planning. Extensive studies show the effectiveness of the
proposed architecture as well as the importance of the VQA tasks for reasoning
and planning in complex 3D scenes.
更多查看译文
AI 理解论文
溯源树
样例
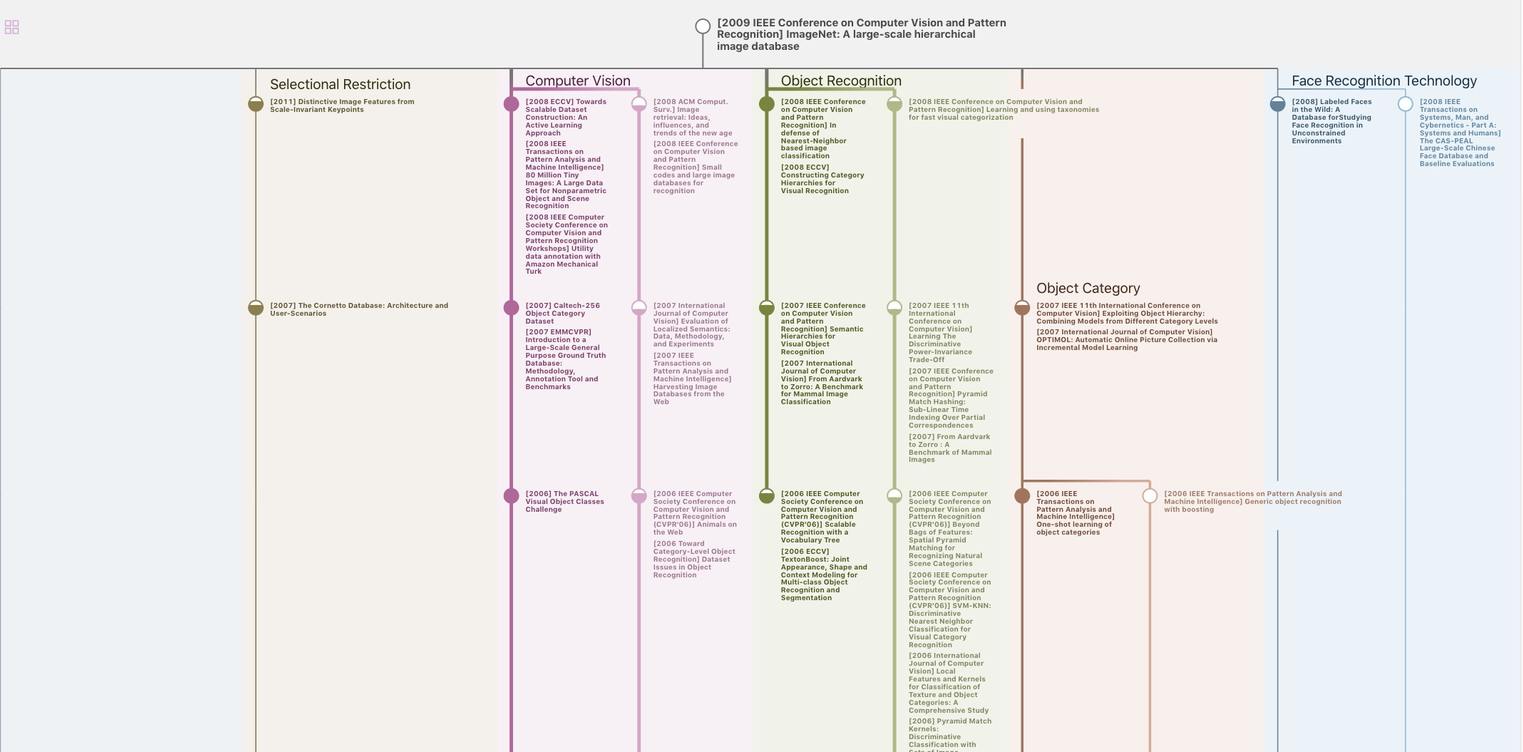
生成溯源树,研究论文发展脉络
数据免责声明
页面数据均来自互联网公开来源、合作出版商和通过AI技术自动分析结果,我们不对页面数据的有效性、准确性、正确性、可靠性、完整性和及时性做出任何承诺和保证。若有疑问,可以通过电子邮件方式联系我们:report@aminer.cn